کد مقاله | کد نشریه | سال انتشار | مقاله انگلیسی | نسخه تمام متن |
---|---|---|---|---|
6299390 | 1617920 | 2015 | 11 صفحه PDF | دانلود رایگان |
عنوان انگلیسی مقاله ISI
Citizen science and field survey observations provide comparable results for mapping Vancouver Island White-tailed Ptarmigan (Lagopus leucura saxatilis) distributions
دانلود مقاله + سفارش ترجمه
دانلود مقاله ISI انگلیسی
رایگان برای ایرانیان
کلمات کلیدی
موضوعات مرتبط
علوم زیستی و بیوفناوری
علوم کشاورزی و بیولوژیک
بوم شناسی، تکامل، رفتار و سامانه شناسی
پیش نمایش صفحه اول مقاله
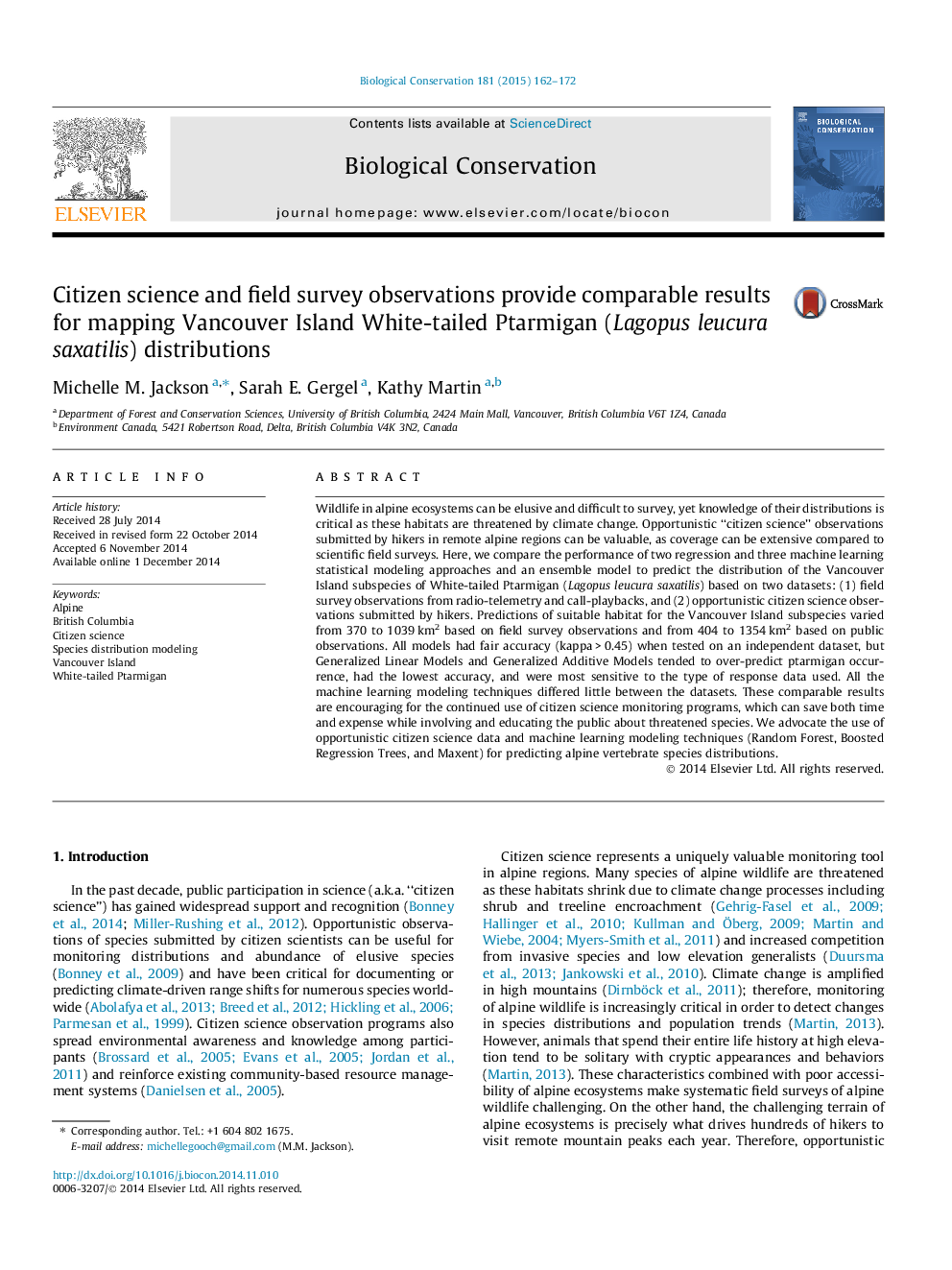
چکیده انگلیسی
Wildlife in alpine ecosystems can be elusive and difficult to survey, yet knowledge of their distributions is critical as these habitats are threatened by climate change. Opportunistic “citizen science” observations submitted by hikers in remote alpine regions can be valuable, as coverage can be extensive compared to scientific field surveys. Here, we compare the performance of two regression and three machine learning statistical modeling approaches and an ensemble model to predict the distribution of the Vancouver Island subspecies of White-tailed Ptarmigan (Lagopus leucura saxatilis) based on two datasets: (1) field survey observations from radio-telemetry and call-playbacks, and (2) opportunistic citizen science observations submitted by hikers. Predictions of suitable habitat for the Vancouver Island subspecies varied from 370 to 1039 km2 based on field survey observations and from 404 to 1354 km2 based on public observations. All models had fair accuracy (kappa > 0.45) when tested on an independent dataset, but Generalized Linear Models and Generalized Additive Models tended to over-predict ptarmigan occurrence, had the lowest accuracy, and were most sensitive to the type of response data used. All the machine learning modeling techniques differed little between the datasets. These comparable results are encouraging for the continued use of citizen science monitoring programs, which can save both time and expense while involving and educating the public about threatened species. We advocate the use of opportunistic citizen science data and machine learning modeling techniques (Random Forest, Boosted Regression Trees, and Maxent) for predicting alpine vertebrate species distributions.
ناشر
Database: Elsevier - ScienceDirect (ساینس دایرکت)
Journal: Biological Conservation - Volume 181, January 2015, Pages 162-172
Journal: Biological Conservation - Volume 181, January 2015, Pages 162-172
نویسندگان
Michelle M. Jackson, Sarah E. Gergel, Kathy Martin,