کد مقاله | کد نشریه | سال انتشار | مقاله انگلیسی | نسخه تمام متن |
---|---|---|---|---|
6680045 | 1428065 | 2018 | 14 صفحه PDF | دانلود رایگان |
عنوان انگلیسی مقاله ISI
Data-driven Urban Energy Simulation (DUE-S): A framework for integrating engineering simulation and machine learning methods in a multi-scale urban energy modeling workflow
دانلود مقاله + سفارش ترجمه
دانلود مقاله ISI انگلیسی
رایگان برای ایرانیان
کلمات کلیدی
موضوعات مرتبط
مهندسی و علوم پایه
مهندسی انرژی
مهندسی انرژی و فناوری های برق
پیش نمایش صفحه اول مقاله
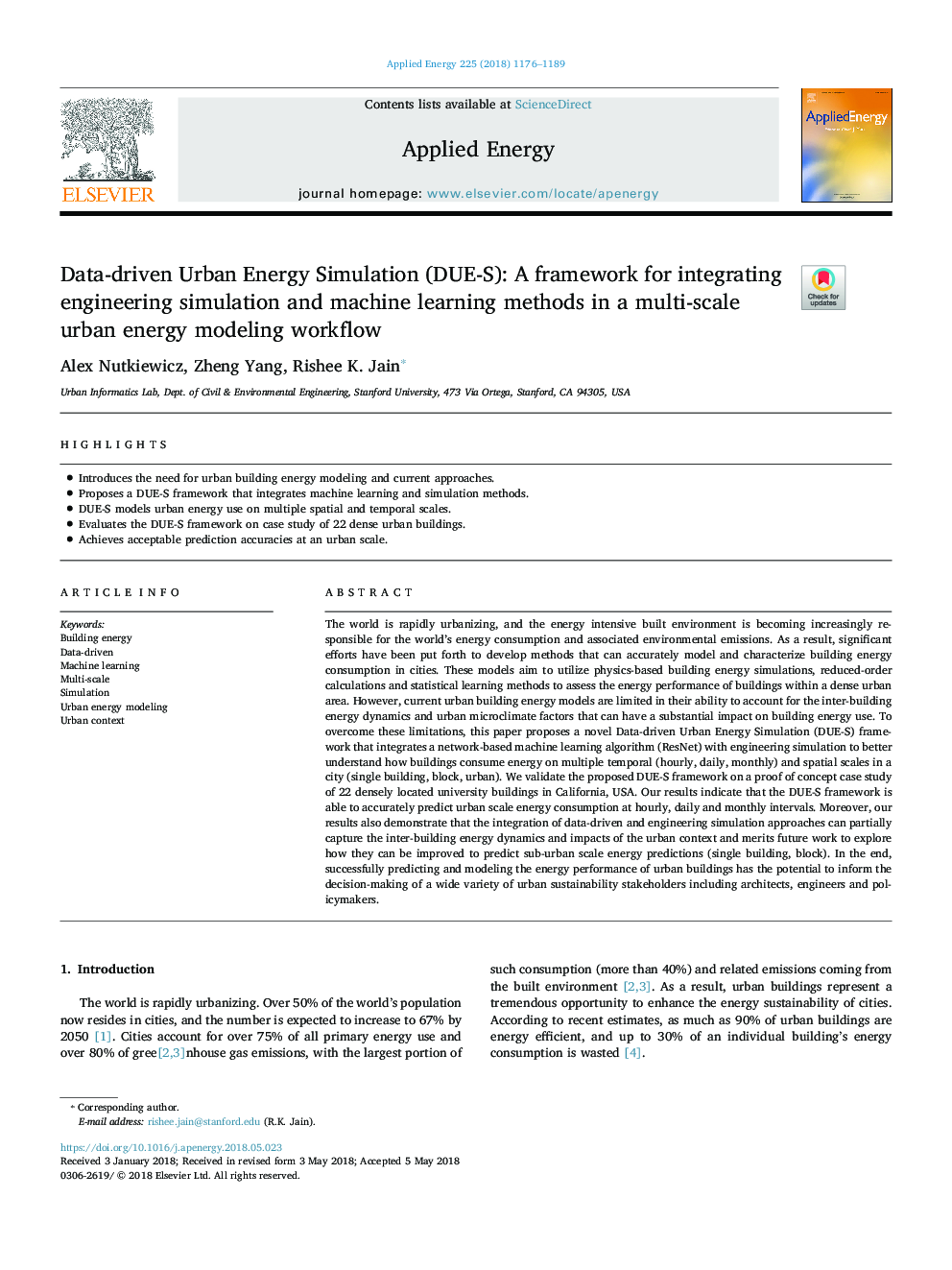
چکیده انگلیسی
The world is rapidly urbanizing, and the energy intensive built environment is becoming increasingly responsible for the world's energy consumption and associated environmental emissions. As a result, significant efforts have been put forth to develop methods that can accurately model and characterize building energy consumption in cities. These models aim to utilize physics-based building energy simulations, reduced-order calculations and statistical learning methods to assess the energy performance of buildings within a dense urban area. However, current urban building energy models are limited in their ability to account for the inter-building energy dynamics and urban microclimate factors that can have a substantial impact on building energy use. To overcome these limitations, this paper proposes a novel Data-driven Urban Energy Simulation (DUE-S) framework that integrates a network-based machine learning algorithm (ResNet) with engineering simulation to better understand how buildings consume energy on multiple temporal (hourly, daily, monthly) and spatial scales in a city (single building, block, urban). We validate the proposed DUE-S framework on a proof of concept case study of 22 densely located university buildings in California, USA. Our results indicate that the DUE-S framework is able to accurately predict urban scale energy consumption at hourly, daily and monthly intervals. Moreover, our results also demonstrate that the integration of data-driven and engineering simulation approaches can partially capture the inter-building energy dynamics and impacts of the urban context and merits future work to explore how they can be improved to predict sub-urban scale energy predictions (single building, block). In the end, successfully predicting and modeling the energy performance of urban buildings has the potential to inform the decision-making of a wide variety of urban sustainability stakeholders including architects, engineers and policymakers.
ناشر
Database: Elsevier - ScienceDirect (ساینس دایرکت)
Journal: Applied Energy - Volume 225, 1 September 2018, Pages 1176-1189
Journal: Applied Energy - Volume 225, 1 September 2018, Pages 1176-1189
نویسندگان
Alex Nutkiewicz, Zheng Yang, Rishee K. Jain,