کد مقاله | کد نشریه | سال انتشار | مقاله انگلیسی | نسخه تمام متن |
---|---|---|---|---|
6753463 | 1430812 | 2018 | 15 صفحه PDF | دانلود رایگان |
عنوان انگلیسی مقاله ISI
Sliding window denoising K-Singular Value Decomposition and its application on rolling bearing impact fault diagnosis
دانلود مقاله + سفارش ترجمه
دانلود مقاله ISI انگلیسی
رایگان برای ایرانیان
کلمات کلیدی
موضوعات مرتبط
مهندسی و علوم پایه
سایر رشته های مهندسی
مهندسی عمران و سازه
پیش نمایش صفحه اول مقاله
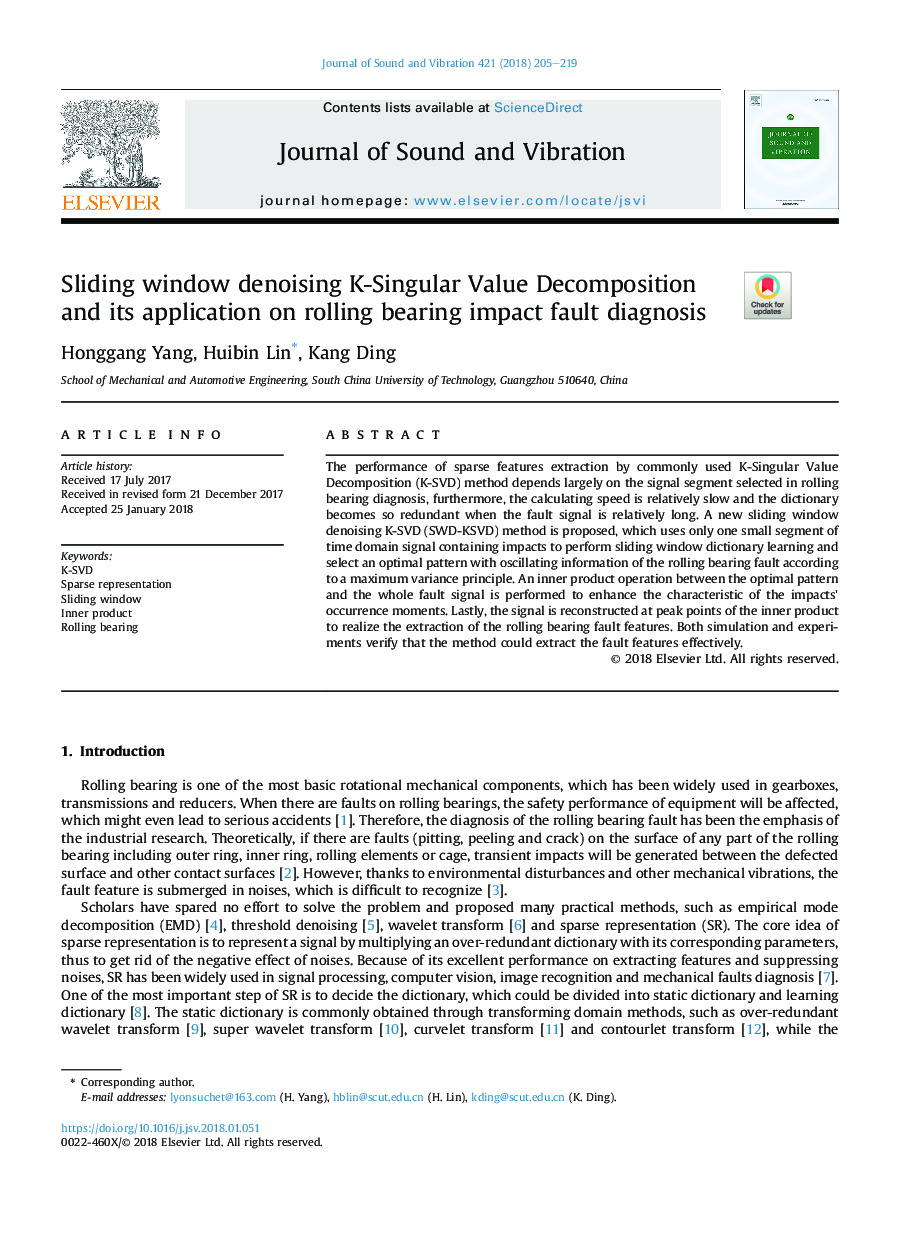
چکیده انگلیسی
The performance of sparse features extraction by commonly used K-Singular Value Decomposition (K-SVD) method depends largely on the signal segment selected in rolling bearing diagnosis, furthermore, the calculating speed is relatively slow and the dictionary becomes so redundant when the fault signal is relatively long. A new sliding window denoising K-SVD (SWD-KSVD) method is proposed, which uses only one small segment of time domain signal containing impacts to perform sliding window dictionary learning and select an optimal pattern with oscillating information of the rolling bearing fault according to a maximum variance principle. An inner product operation between the optimal pattern and the whole fault signal is performed to enhance the characteristic of the impacts' occurrence moments. Lastly, the signal is reconstructed at peak points of the inner product to realize the extraction of the rolling bearing fault features. Both simulation and experiments verify that the method could extract the fault features effectively.
ناشر
Database: Elsevier - ScienceDirect (ساینس دایرکت)
Journal: Journal of Sound and Vibration - Volume 421, 12 May 2018, Pages 205-219
Journal: Journal of Sound and Vibration - Volume 421, 12 May 2018, Pages 205-219
نویسندگان
Honggang Yang, Huibin Lin, Kang Ding,