کد مقاله | کد نشریه | سال انتشار | مقاله انگلیسی | نسخه تمام متن |
---|---|---|---|---|
6757212 | 1431094 | 2018 | 16 صفحه PDF | دانلود رایگان |
عنوان انگلیسی مقاله ISI
Data-driven modeling of vortex-induced vibration of a long-span suspension bridge using decision tree learning and support vector regression
دانلود مقاله + سفارش ترجمه
دانلود مقاله ISI انگلیسی
رایگان برای ایرانیان
کلمات کلیدی
موضوعات مرتبط
مهندسی و علوم پایه
مهندسی انرژی
انرژی های تجدید پذیر، توسعه پایدار و محیط زیست
پیش نمایش صفحه اول مقاله
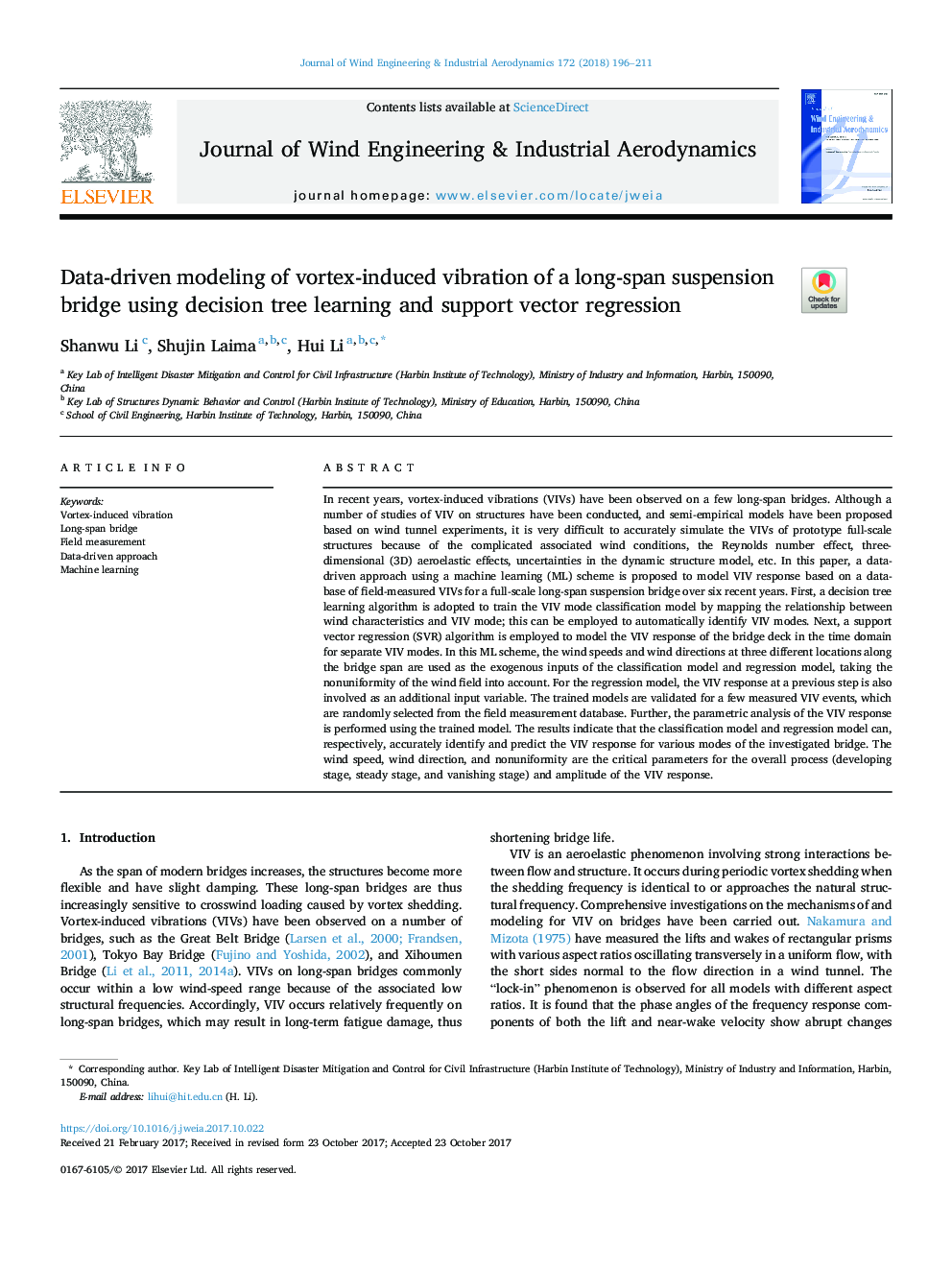
چکیده انگلیسی
In recent years, vortex-induced vibrations (VIVs) have been observed on a few long-span bridges. Although a number of studies of VIV on structures have been conducted, and semi-empirical models have been proposed based on wind tunnel experiments, it is very difficult to accurately simulate the VIVs of prototype full-scale structures because of the complicated associated wind conditions, the Reynolds number effect, three-dimensional (3D) aeroelastic effects, uncertainties in the dynamic structure model, etc. In this paper, a data-driven approach using a machine learning (ML) scheme is proposed to model VIV response based on a database of field-measured VIVs for a full-scale long-span suspension bridge over six recent years. First, a decision tree learning algorithm is adopted to train the VIV mode classification model by mapping the relationship between wind characteristics and VIV mode; this can be employed to automatically identify VIV modes. Next, a support vector regression (SVR) algorithm is employed to model the VIV response of the bridge deck in the time domain for separate VIV modes. In this ML scheme, the wind speeds and wind directions at three different locations along the bridge span are used as the exogenous inputs of the classification model and regression model, taking the nonuniformity of the wind field into account. For the regression model, the VIV response at a previous step is also involved as an additional input variable. The trained models are validated for a few measured VIV events, which are randomly selected from the field measurement database. Further, the parametric analysis of the VIV response is performed using the trained model. The results indicate that the classification model and regression model can, respectively, accurately identify and predict the VIV response for various modes of the investigated bridge. The wind speed, wind direction, and nonuniformity are the critical parameters for the overall process (developing stage, steady stage, and vanishing stage) and amplitude of the VIV response.
ناشر
Database: Elsevier - ScienceDirect (ساینس دایرکت)
Journal: Journal of Wind Engineering and Industrial Aerodynamics - Volume 172, January 2018, Pages 196-211
Journal: Journal of Wind Engineering and Industrial Aerodynamics - Volume 172, January 2018, Pages 196-211
نویسندگان
Shanwu Li, Shujin Laima, Hui Li,