کد مقاله | کد نشریه | سال انتشار | مقاله انگلیسی | نسخه تمام متن |
---|---|---|---|---|
6858603 | 1438286 | 2018 | 15 صفحه PDF | دانلود رایگان |
عنوان انگلیسی مقاله ISI
BLOSS: Effective meta-blocking with almost no effort
دانلود مقاله + سفارش ترجمه
دانلود مقاله ISI انگلیسی
رایگان برای ایرانیان
موضوعات مرتبط
مهندسی و علوم پایه
مهندسی کامپیوتر
هوش مصنوعی
پیش نمایش صفحه اول مقاله
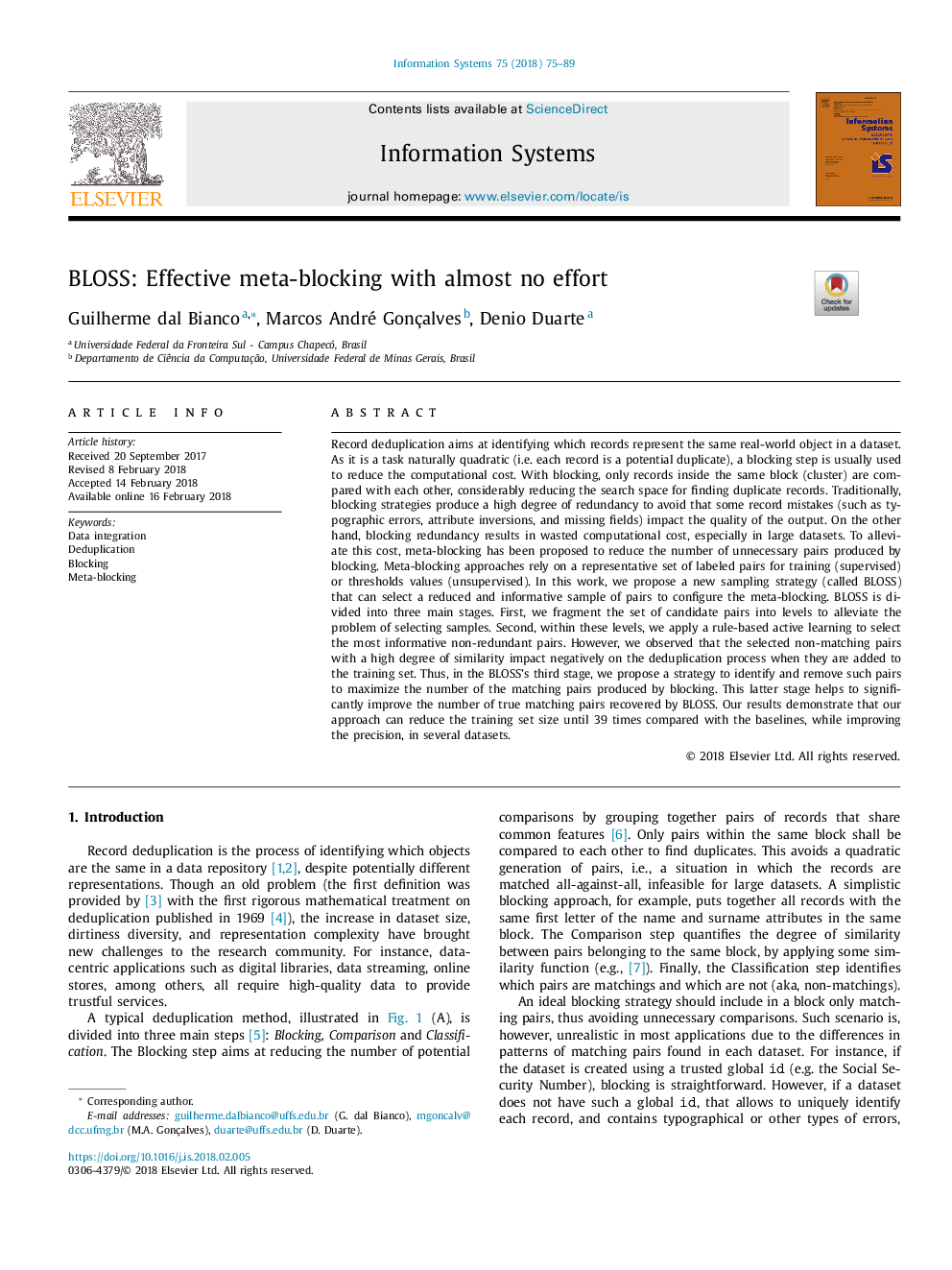
چکیده انگلیسی
Record deduplication aims at identifying which records represent the same real-world object in a dataset. As it is a task naturally quadratic (i.e. each record is a potential duplicate), a blocking step is usually used to reduce the computational cost. With blocking, only records inside the same block (cluster) are compared with each other, considerably reducing the search space for finding duplicate records. Traditionally, blocking strategies produce a high degree of redundancy to avoid that some record mistakes (such as typographic errors, attribute inversions, and missing fields) impact the quality of the output. On the other hand, blocking redundancy results in wasted computational cost, especially in large datasets. To alleviate this cost, meta-blocking has been proposed to reduce the number of unnecessary pairs produced by blocking. Meta-blocking approaches rely on a representative set of labeled pairs for training (supervised) or thresholds values (unsupervised). In this work, we propose a new sampling strategy (called BLOSS) that can select a reduced and informative sample of pairs to configure the meta-blocking. BLOSS is divided into three main stages. First, we fragment the set of candidate pairs into levels to alleviate the problem of selecting samples. Second, within these levels, we apply a rule-based active learning to select the most informative non-redundant pairs. However, we observed that the selected non-matching pairs with a high degree of similarity impact negatively on the deduplication process when they are added to the training set. Thus, in the BLOSS's third stage, we propose a strategy to identify and remove such pairs to maximize the number of the matching pairs produced by blocking. This latter stage helps to significantly improve the number of true matching pairs recovered by BLOSS. Our results demonstrate that our approach can reduce the training set size until 39 times compared with the baselines, while improving the precision, in several datasets.
ناشر
Database: Elsevier - ScienceDirect (ساینس دایرکت)
Journal: Information Systems - Volume 75, June 2018, Pages 75-89
Journal: Information Systems - Volume 75, June 2018, Pages 75-89
نویسندگان
Guilherme dal Bianco, Marcos André Gonçalves, Denio Duarte,