کد مقاله | کد نشریه | سال انتشار | مقاله انگلیسی | نسخه تمام متن |
---|---|---|---|---|
6861513 | 1439253 | 2018 | 14 صفحه PDF | دانلود رایگان |
عنوان انگلیسی مقاله ISI
Scaling KNN multi-class twin support vector machine via safe instance reduction
دانلود مقاله + سفارش ترجمه
دانلود مقاله ISI انگلیسی
رایگان برای ایرانیان
موضوعات مرتبط
مهندسی و علوم پایه
مهندسی کامپیوتر
هوش مصنوعی
پیش نمایش صفحه اول مقاله
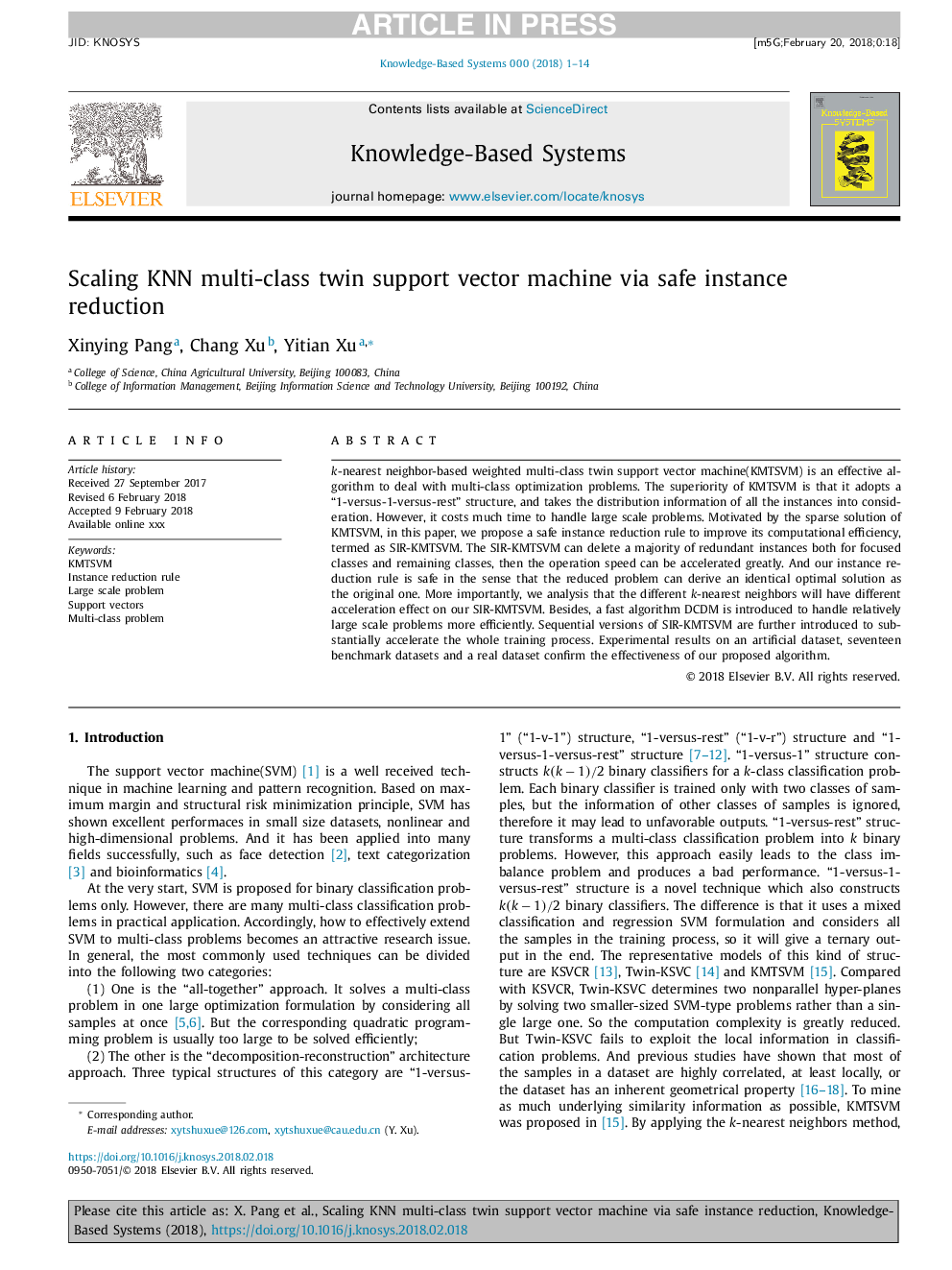
چکیده انگلیسی
k-nearest neighbor-based weighted multi-class twin support vector machine(KMTSVM) is an effective algorithm to deal with multi-class optimization problems. The superiority of KMTSVM is that it adopts a “1-versus-1-versus-rest” structure, and takes the distribution information of all the instances into consideration. However, it costs much time to handle large scale problems. Motivated by the sparse solution of KMTSVM, in this paper, we propose a safe instance reduction rule to improve its computational efficiency, termed as SIR-KMTSVM. The SIR-KMTSVM can delete a majority of redundant instances both for focused classes and remaining classes, then the operation speed can be accelerated greatly. And our instance reduction rule is safe in the sense that the reduced problem can derive an identical optimal solution as the original one. More importantly, we analysis that the different k-nearest neighbors will have different acceleration effect on our SIR-KMTSVM. Besides, a fast algorithm DCDM is introduced to handle relatively large scale problems more efficiently. Sequential versions of SIR-KMTSVM are further introduced to substantially accelerate the whole training process. Experimental results on an artificial dataset, seventeen benchmark datasets and a real dataset confirm the effectiveness of our proposed algorithm.
ناشر
Database: Elsevier - ScienceDirect (ساینس دایرکت)
Journal: Knowledge-Based Systems - Volume 148, 15 May 2018, Pages 17-30
Journal: Knowledge-Based Systems - Volume 148, 15 May 2018, Pages 17-30
نویسندگان
Xinying Pang, Chang Xu, Yitian Xu,