کد مقاله | کد نشریه | سال انتشار | مقاله انگلیسی | نسخه تمام متن |
---|---|---|---|---|
6861514 | 1439253 | 2018 | 10 صفحه PDF | دانلود رایگان |
عنوان انگلیسی مقاله ISI
CPLR: Collaborative pairwise learning to rank for personalized recommendation
دانلود مقاله + سفارش ترجمه
دانلود مقاله ISI انگلیسی
رایگان برای ایرانیان
کلمات کلیدی
موضوعات مرتبط
مهندسی و علوم پایه
مهندسی کامپیوتر
هوش مصنوعی
پیش نمایش صفحه اول مقاله
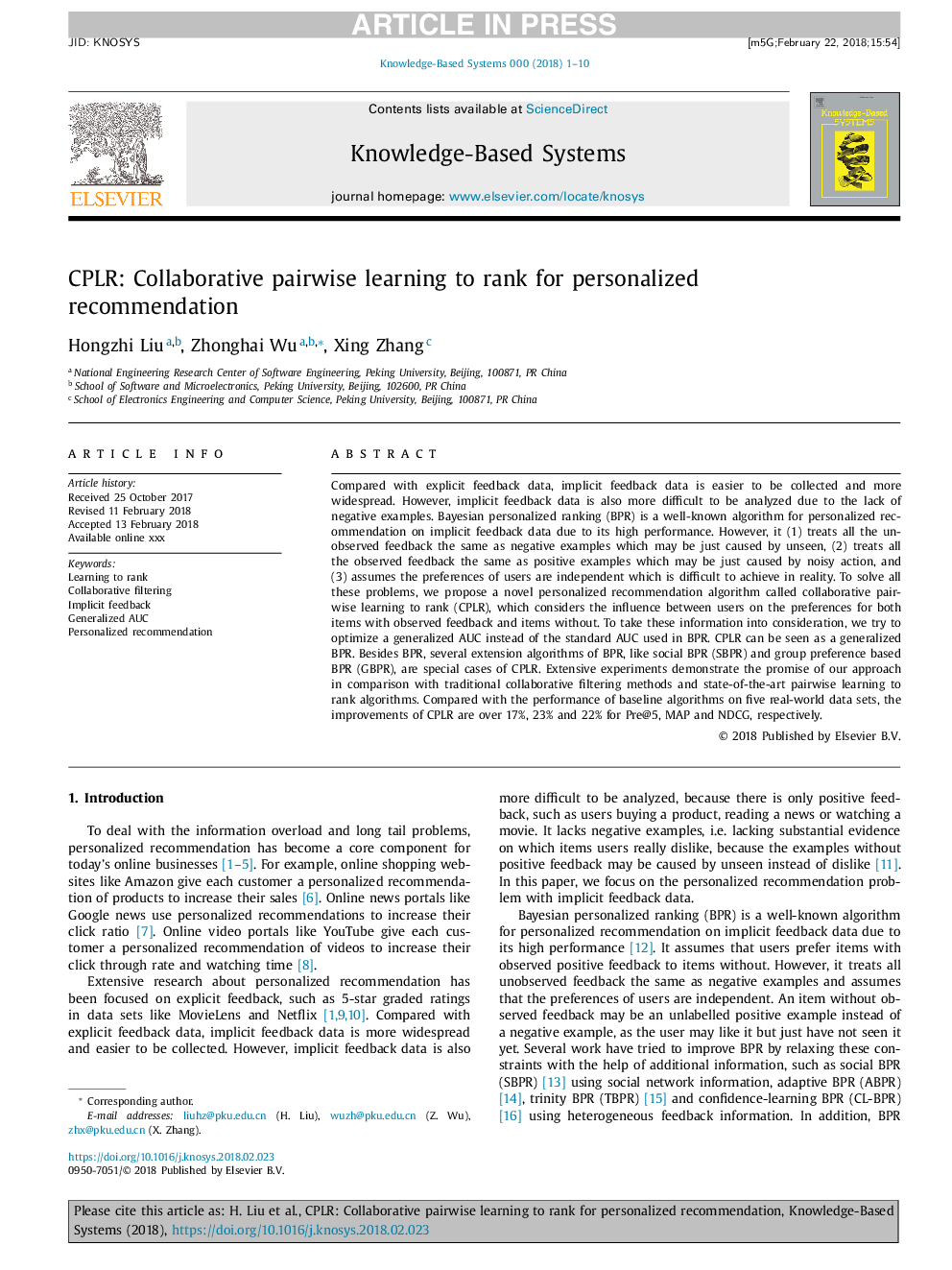
چکیده انگلیسی
Compared with explicit feedback data, implicit feedback data is easier to be collected and more widespread. However, implicit feedback data is also more difficult to be analyzed due to the lack of negative examples. Bayesian personalized ranking (BPR) is a well-known algorithm for personalized recommendation on implicit feedback data due to its high performance. However, it (1) treats all the unobserved feedback the same as negative examples which may be just caused by unseen, (2) treats all the observed feedback the same as positive examples which may be just caused by noisy action, and (3) assumes the preferences of users are independent which is difficult to achieve in reality. To solve all these problems, we propose a novel personalized recommendation algorithm called collaborative pairwise learning to rank (CPLR), which considers the influence between users on the preferences for both items with observed feedback and items without. To take these information into consideration, we try to optimize a generalized AUC instead of the standard AUC used in BPR. CPLR can be seen as a generalized BPR. Besides BPR, several extension algorithms of BPR, like social BPR (SBPR) and group preference based BPR (GBPR), are special cases of CPLR. Extensive experiments demonstrate the promise of our approach in comparison with traditional collaborative filtering methods and state-of-the-art pairwise learning to rank algorithms. Compared with the performance of baseline algorithms on five real-world data sets, the improvements of CPLR are over 17%, 23% and 22% for Pre@5, MAP and NDCG, respectively.
ناشر
Database: Elsevier - ScienceDirect (ساینس دایرکت)
Journal: Knowledge-Based Systems - Volume 148, 15 May 2018, Pages 31-40
Journal: Knowledge-Based Systems - Volume 148, 15 May 2018, Pages 31-40
نویسندگان
Hongzhi Liu, Zhonghai Wu, Xing Zhang,