کد مقاله | کد نشریه | سال انتشار | مقاله انگلیسی | نسخه تمام متن |
---|---|---|---|---|
6862831 | 1439397 | 2018 | 22 صفحه PDF | دانلود رایگان |
عنوان انگلیسی مقاله ISI
Training sparse least squares support vector machines by the QR decomposition
دانلود مقاله + سفارش ترجمه
دانلود مقاله ISI انگلیسی
رایگان برای ایرانیان
کلمات کلیدی
موضوعات مرتبط
مهندسی و علوم پایه
مهندسی کامپیوتر
هوش مصنوعی
پیش نمایش صفحه اول مقاله
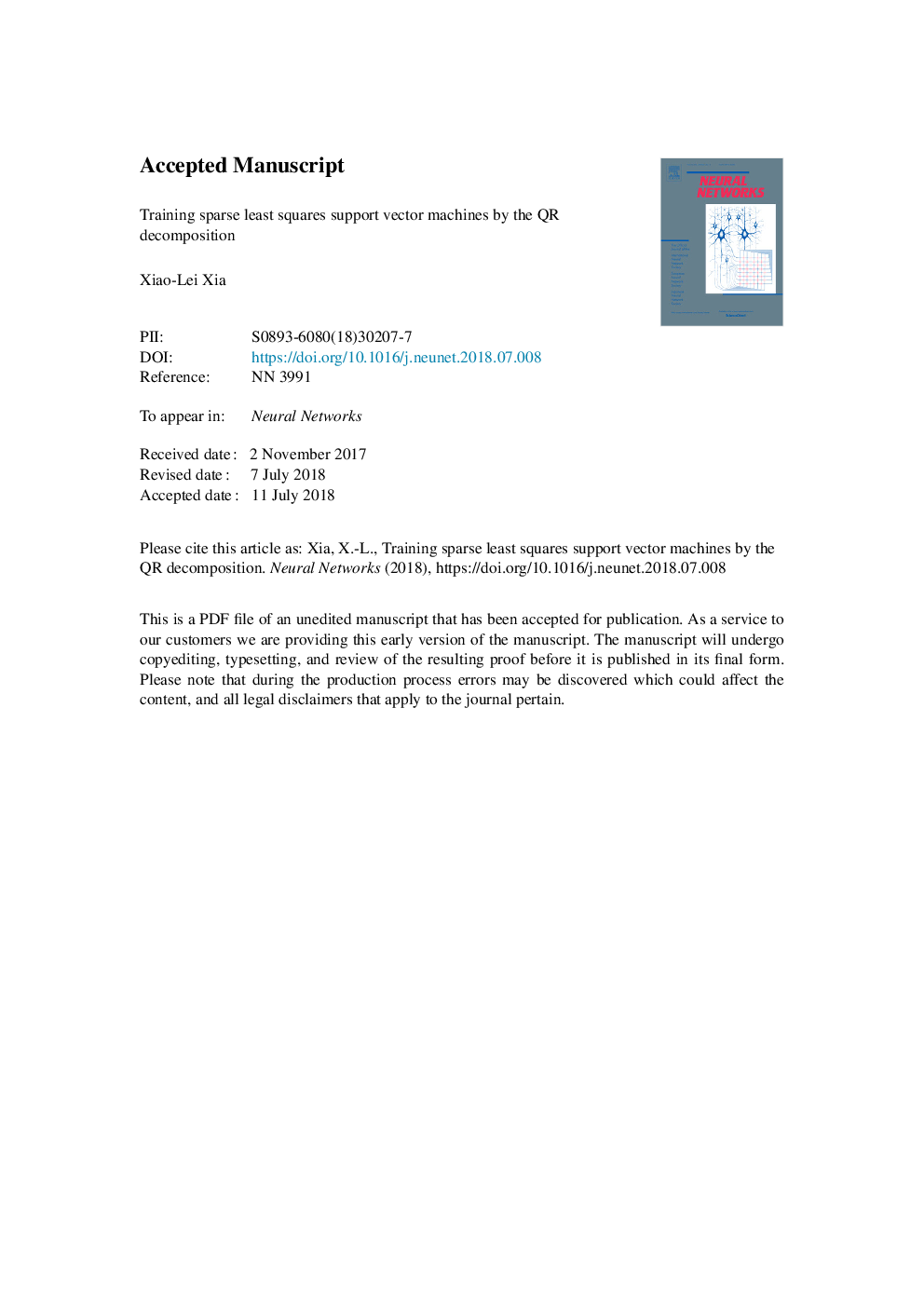
چکیده انگلیسی
The solution of an LS-SVM has suffered from the problem of non-sparseness. The paper proposed to apply the KMP algorithm, with the number of support vectors as the regularization parameter, to tackle the non-sparseness problem of LS-SVMs. The idea of the kernel matching pursuit (KMP) algorithm was first revisited from the perspective of the QR decomposition of the kernel matrix on the training set. Strategies are further developed to select those support vectors which minimize the leave-one-out cross validation error of the resultant sparse LS-SVM model. It is demonstrated that the LOOCV of the sparse LS-SVM can be computed accurately and efficiently. Experimental results on benchmark datasets showed that, compared to the SVM and variants sparse LS-SVM models, the proposed sparse LS-SVM models developed upon KMP algorithms maintained comparable performance in terms of both accuracy and sparsity.
ناشر
Database: Elsevier - ScienceDirect (ساینس دایرکت)
Journal: Neural Networks - Volume 106, October 2018, Pages 175-184
Journal: Neural Networks - Volume 106, October 2018, Pages 175-184
نویسندگان
Xiao-Lei Xia,