کد مقاله | کد نشریه | سال انتشار | مقاله انگلیسی | نسخه تمام متن |
---|---|---|---|---|
6868638 | 1440029 | 2018 | 37 صفحه PDF | دانلود رایگان |
عنوان انگلیسی مقاله ISI
Mode jumping MCMC for Bayesian variable selection in GLMM
دانلود مقاله + سفارش ترجمه
دانلود مقاله ISI انگلیسی
رایگان برای ایرانیان
کلمات کلیدی
موضوعات مرتبط
مهندسی و علوم پایه
مهندسی کامپیوتر
نظریه محاسباتی و ریاضیات
پیش نمایش صفحه اول مقاله
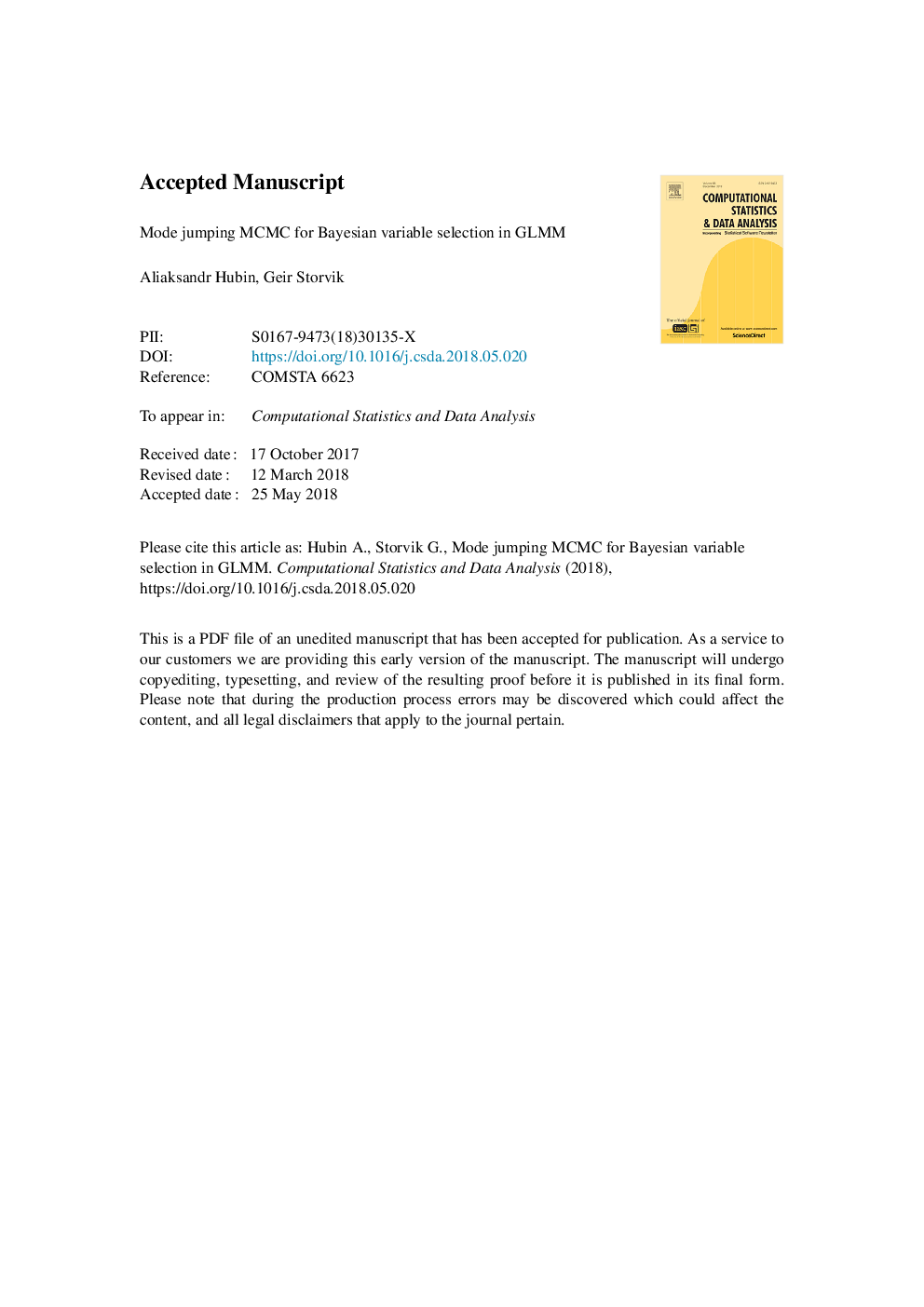
چکیده انگلیسی
Generalized linear mixed models (GLMM) are used for inference and prediction in a wide range of different applications providing a powerful scientific tool. An increasing number of sources of data are becoming available, introducing a variety of candidate explanatory variables for these models. Selection of an optimal combination of variables is thus becoming crucial. In a Bayesian setting, the posterior distribution of the models, based on the observed data, can be viewed as a relevant measure for the model evidence. The number of possible models increases exponentially in the number of candidate variables. Moreover, the space of models has numerous local extrema in terms of posterior model probabilities. To resolve these issues a novel MCMC algorithm for the search through the model space via efficient mode jumping for GLMMs is introduced. The algorithm is based on that marginal likelihoods can be efficiently calculated within each model. It is recommended that either exact expressions or precise approximations of marginal likelihoods are applied. The suggested algorithm is applied to simulated data, the famous U.S. crime data, protein activity data and epigenetic data and is compared to several existing approaches.
ناشر
Database: Elsevier - ScienceDirect (ساینس دایرکت)
Journal: Computational Statistics & Data Analysis - Volume 127, November 2018, Pages 281-297
Journal: Computational Statistics & Data Analysis - Volume 127, November 2018, Pages 281-297
نویسندگان
Aliaksandr Hubin, Geir Storvik,