کد مقاله | کد نشریه | سال انتشار | مقاله انگلیسی | نسخه تمام متن |
---|---|---|---|---|
6868722 | 1440033 | 2018 | 18 صفحه PDF | دانلود رایگان |
عنوان انگلیسی مقاله ISI
Clustering sparse binary data with hierarchical Bayesian Bernoulli mixture model
دانلود مقاله + سفارش ترجمه
دانلود مقاله ISI انگلیسی
رایگان برای ایرانیان
کلمات کلیدی
موضوعات مرتبط
مهندسی و علوم پایه
مهندسی کامپیوتر
نظریه محاسباتی و ریاضیات
پیش نمایش صفحه اول مقاله
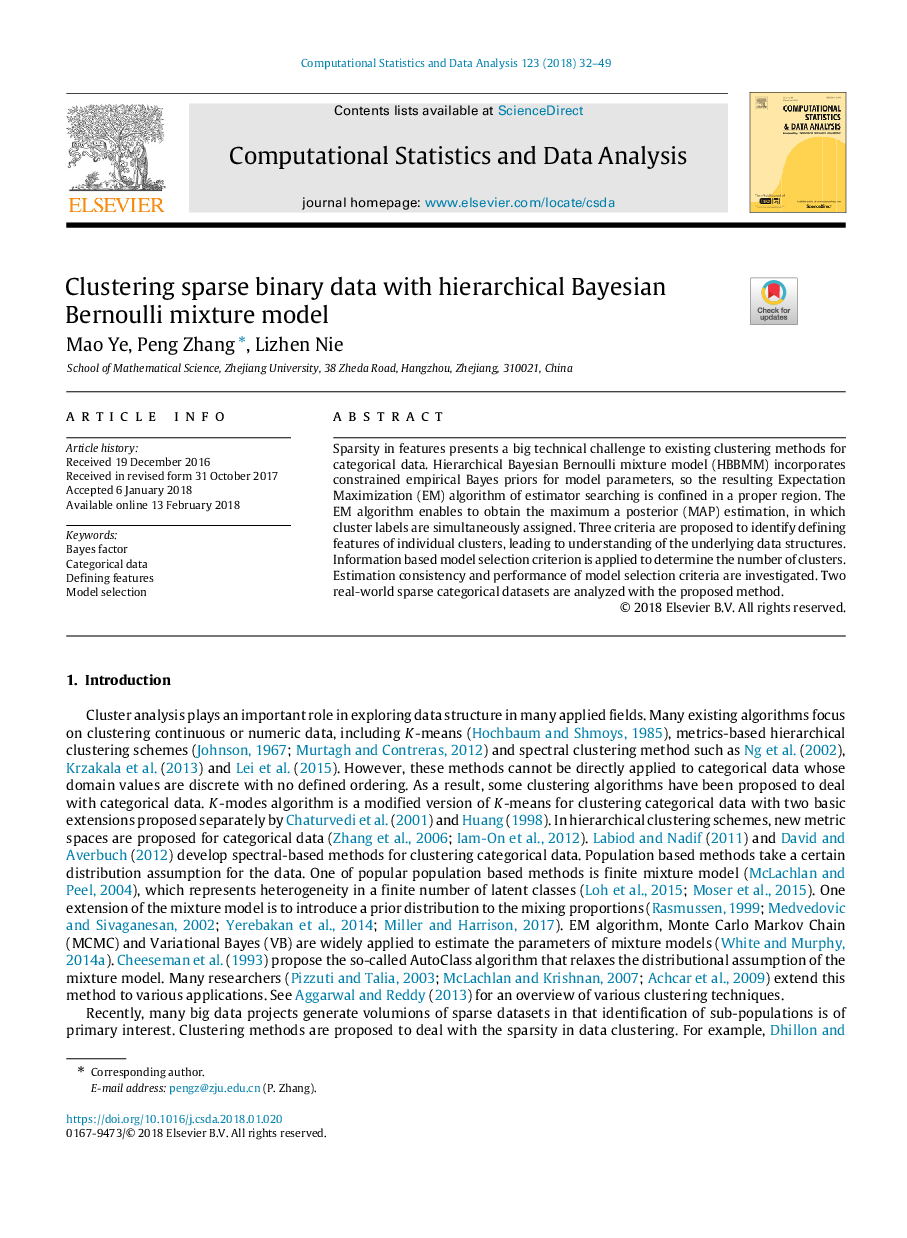
چکیده انگلیسی
Sparsity in features presents a big technical challenge to existing clustering methods for categorical data. Hierarchical Bayesian Bernoulli mixture model (HBBMM) incorporates constrained empirical Bayes priors for model parameters, so the resulting Expectation Maximization (EM) algorithm of estimator searching is confined in a proper region. The EM algorithm enables to obtain the maximum a posterior (MAP) estimation, in which cluster labels are simultaneously assigned. Three criteria are proposed to identify defining features of individual clusters, leading to understanding of the underlying data structures. Information based model selection criterion is applied to determine the number of clusters. Estimation consistency and performance of model selection criteria are investigated. Two real-world sparse categorical datasets are analyzed with the proposed method.
ناشر
Database: Elsevier - ScienceDirect (ساینس دایرکت)
Journal: Computational Statistics & Data Analysis - Volume 123, July 2018, Pages 32-49
Journal: Computational Statistics & Data Analysis - Volume 123, July 2018, Pages 32-49
نویسندگان
Mao Ye, Peng Zhang, Lizhen Nie,