کد مقاله | کد نشریه | سال انتشار | مقاله انگلیسی | نسخه تمام متن |
---|---|---|---|---|
6869226 | 681495 | 2016 | 21 صفحه PDF | دانلود رایگان |
عنوان انگلیسی مقاله ISI
Iteratively reweighted adaptive lasso for conditional heteroscedastic time series with applications to AR-ARCH type processes
دانلود مقاله + سفارش ترجمه
دانلود مقاله ISI انگلیسی
رایگان برای ایرانیان
کلمات کلیدی
موضوعات مرتبط
مهندسی و علوم پایه
مهندسی کامپیوتر
نظریه محاسباتی و ریاضیات
پیش نمایش صفحه اول مقاله
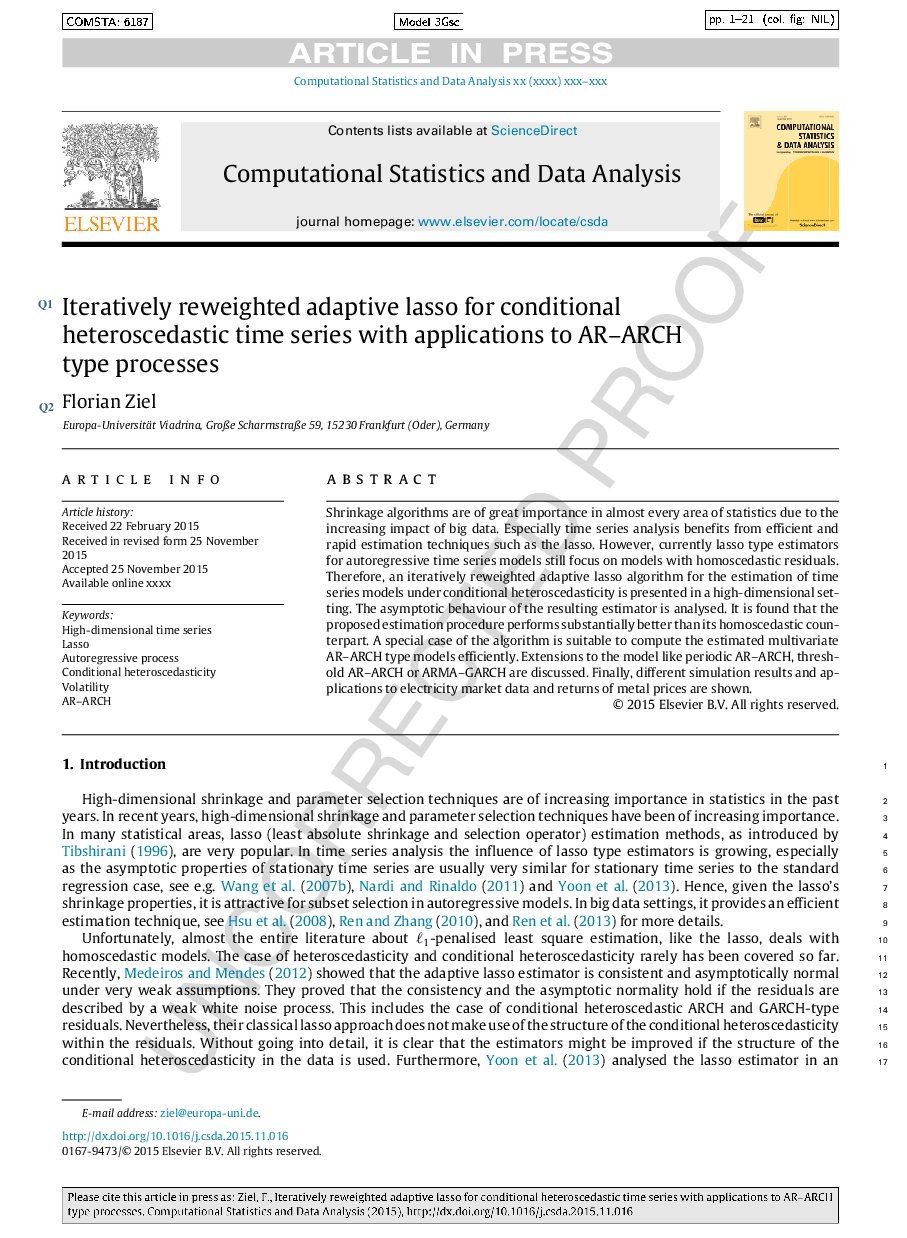
چکیده انگلیسی
Shrinkage algorithms are of great importance in almost every area of statistics due to the increasing impact of big data. Especially time series analysis benefits from efficient and rapid estimation techniques such as the lasso. However, currently lasso type estimators for autoregressive time series models still focus on models with homoscedastic residuals. Therefore, an iteratively reweighted adaptive lasso algorithm for the estimation of time series models under conditional heteroscedasticity is presented in a high-dimensional setting. The asymptotic behaviour of the resulting estimator is analysed. It is found that the proposed estimation procedure performs substantially better than its homoscedastic counterpart. A special case of the algorithm is suitable to compute the estimated multivariate AR-ARCH type models efficiently. Extensions to the model like periodic AR-ARCH, threshold AR-ARCH or ARMA-GARCH are discussed. Finally, different simulation results and applications to electricity market data and returns of metal prices are shown.
ناشر
Database: Elsevier - ScienceDirect (ساینس دایرکت)
Journal: Computational Statistics & Data Analysis - Volume 100, August 2016, Pages 773-793
Journal: Computational Statistics & Data Analysis - Volume 100, August 2016, Pages 773-793
نویسندگان
Florian Ziel,