کد مقاله | کد نشریه | سال انتشار | مقاله انگلیسی | نسخه تمام متن |
---|---|---|---|---|
6876628 | 1442530 | 2018 | 14 صفحه PDF | دانلود رایگان |
عنوان انگلیسی مقاله ISI
Jointly learning shape descriptors and their correspondence via deep triplet CNNs
دانلود مقاله + سفارش ترجمه
دانلود مقاله ISI انگلیسی
رایگان برای ایرانیان
موضوعات مرتبط
مهندسی و علوم پایه
مهندسی کامپیوتر
گرافیک کامپیوتری و طراحی به کمک کامپیوتر
پیش نمایش صفحه اول مقاله
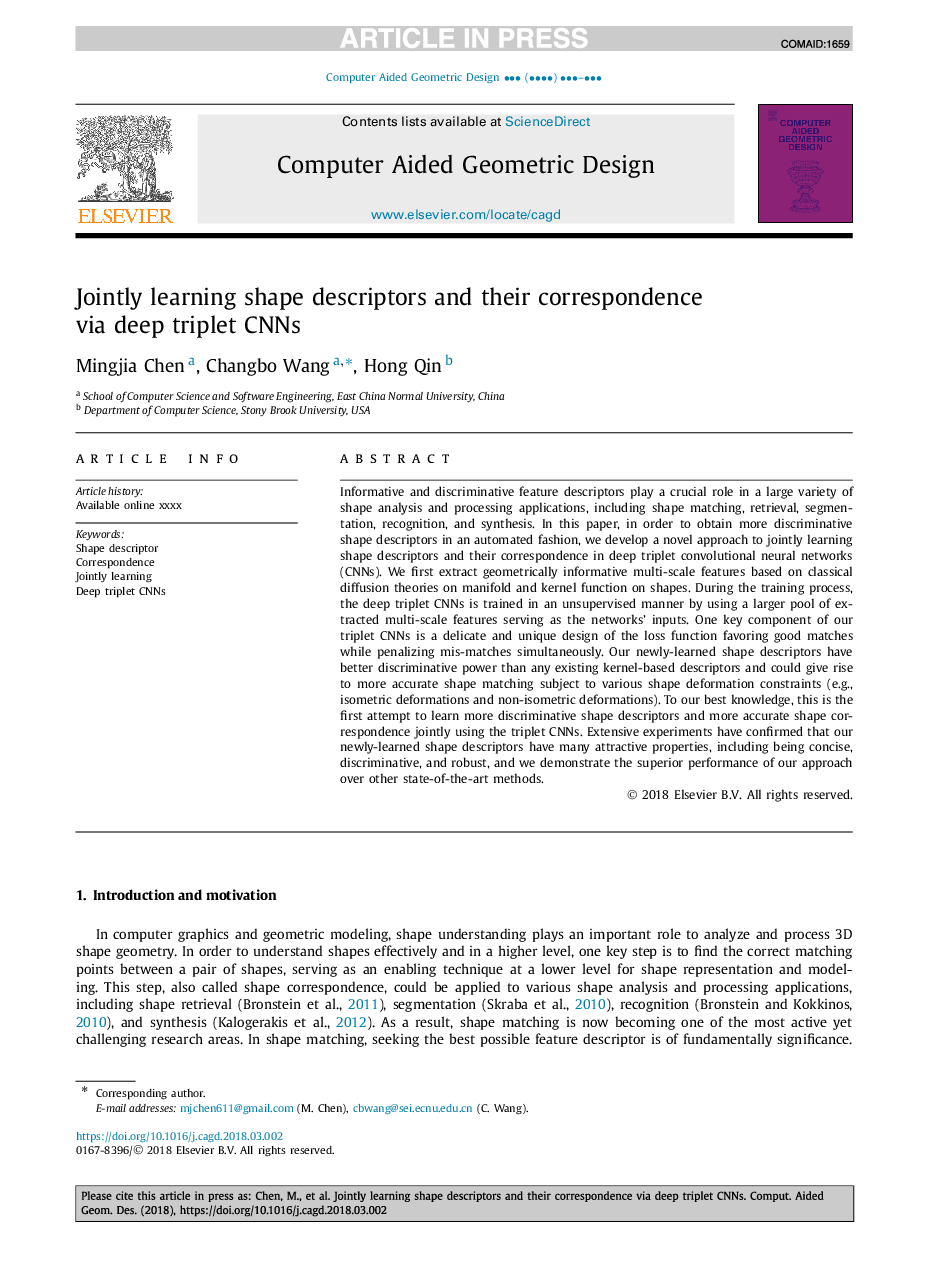
چکیده انگلیسی
Informative and discriminative feature descriptors play a crucial role in a large variety of shape analysis and processing applications, including shape matching, retrieval, segmentation, recognition, and synthesis. In this paper, in order to obtain more discriminative shape descriptors in an automated fashion, we develop a novel approach to jointly learning shape descriptors and their correspondence in deep triplet convolutional neural networks (CNNs). We first extract geometrically informative multi-scale features based on classical diffusion theories on manifold and kernel function on shapes. During the training process, the deep triplet CNNs is trained in an unsupervised manner by using a larger pool of extracted multi-scale features serving as the networks' inputs. One key component of our triplet CNNs is a delicate and unique design of the loss function favoring good matches while penalizing mis-matches simultaneously. Our newly-learned shape descriptors have better discriminative power than any existing kernel-based descriptors and could give rise to more accurate shape matching subject to various shape deformation constraints (e.g., isometric deformations and non-isometric deformations). To our best knowledge, this is the first attempt to learn more discriminative shape descriptors and more accurate shape correspondence jointly using the triplet CNNs. Extensive experiments have confirmed that our newly-learned shape descriptors have many attractive properties, including being concise, discriminative, and robust, and we demonstrate the superior performance of our approach over other state-of-the-art methods.
ناشر
Database: Elsevier - ScienceDirect (ساینس دایرکت)
Journal: Computer Aided Geometric Design - Volume 62, May 2018, Pages 192-205
Journal: Computer Aided Geometric Design - Volume 62, May 2018, Pages 192-205
نویسندگان
Mingjia Chen, Changbo Wang, Hong Qin,