کد مقاله | کد نشریه | سال انتشار | مقاله انگلیسی | نسخه تمام متن |
---|---|---|---|---|
6903657 | 1446992 | 2018 | 41 صفحه PDF | دانلود رایگان |
عنوان انگلیسی مقاله ISI
A league championship algorithm equipped with network structure and backward Q-learning for extracting stock trading rules
دانلود مقاله + سفارش ترجمه
دانلود مقاله ISI انگلیسی
رایگان برای ایرانیان
کلمات کلیدی
موضوعات مرتبط
مهندسی و علوم پایه
مهندسی کامپیوتر
نرم افزارهای علوم کامپیوتر
پیش نمایش صفحه اول مقاله
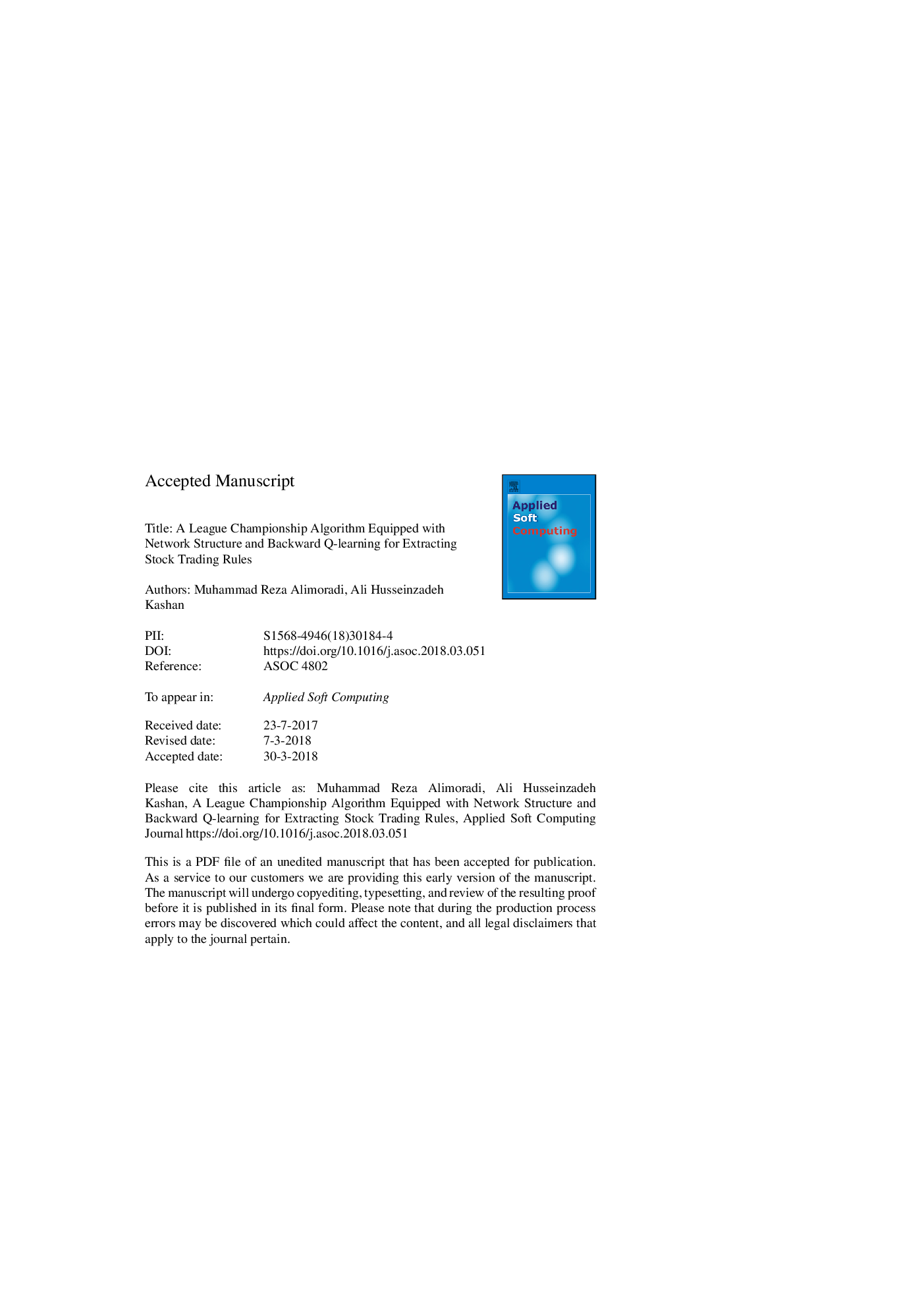
چکیده انگلیسی
Buying and selling stocks are the main activities of investment in the financial markets. During several years, financial experts have designed various indices and indicators to develop intelligent methods to decide whether buy or sell or no trade a specific stock. In this paper, the league championship algorithm (LCA) is adapted and equipped with a network structure (which provides the implicit memory function, compact structure and gives the ability of reusing nodes) for stock trading rule extraction process. The proposed algorithm is able to extract and save various stock trading rules for various kinds of stock market conditions. Each individual in LCA makes role as a sport team which could learn from weaknesses and strengths of others to enhance its performance. The network structure used for each individual is equipped with reinforcement learning to strength the searching power and extracting better rules. The backward Q-learning (BQL), which is a combination of Sarsa learning and Q-learning algorithms, is also hybridized with LCA to increase the learning speed to extract better rules. A trend clustering technique is also developed to determine uptrends and downtrends and to remedy delay in the trend direction reflection along with moving average of stock prices. Finally, to use the trading rules extracted and saved in the rule pools, a new approach through average matching degree is introduced which could create trinary signals of buying, selling, and no trade. The effectiveness of the proposed approach is tested on a set of 20 companies, which have high liquidity and large market capitalization, chosen from various fields of Tehran Stock Exchange. The used historical price data was adjusted through factors of dividends and capital increase. Computational tests and simulations indicate that our hybrid method clearly offers higher profits and lower losses than those of genetic network programming method with rule accumulation (GNP-RA) on all stocks and those of the Buy&Hold strategy on 17 out of 20 instances (85%), especially when the price uptrends are sharp.
ناشر
Database: Elsevier - ScienceDirect (ساینس دایرکت)
Journal: Applied Soft Computing - Volume 68, July 2018, Pages 478-493
Journal: Applied Soft Computing - Volume 68, July 2018, Pages 478-493
نویسندگان
Muhammad Reza Alimoradi, Ali Husseinzadeh Kashan,