کد مقاله | کد نشریه | سال انتشار | مقاله انگلیسی | نسخه تمام متن |
---|---|---|---|---|
6915482 | 1447399 | 2018 | 27 صفحه PDF | دانلود رایگان |
عنوان انگلیسی مقاله ISI
Sparse polynomial chaos expansions via compressed sensing and D-optimal design
دانلود مقاله + سفارش ترجمه
دانلود مقاله ISI انگلیسی
رایگان برای ایرانیان
کلمات کلیدی
موضوعات مرتبط
مهندسی و علوم پایه
مهندسی کامپیوتر
نرم افزارهای علوم کامپیوتر
پیش نمایش صفحه اول مقاله
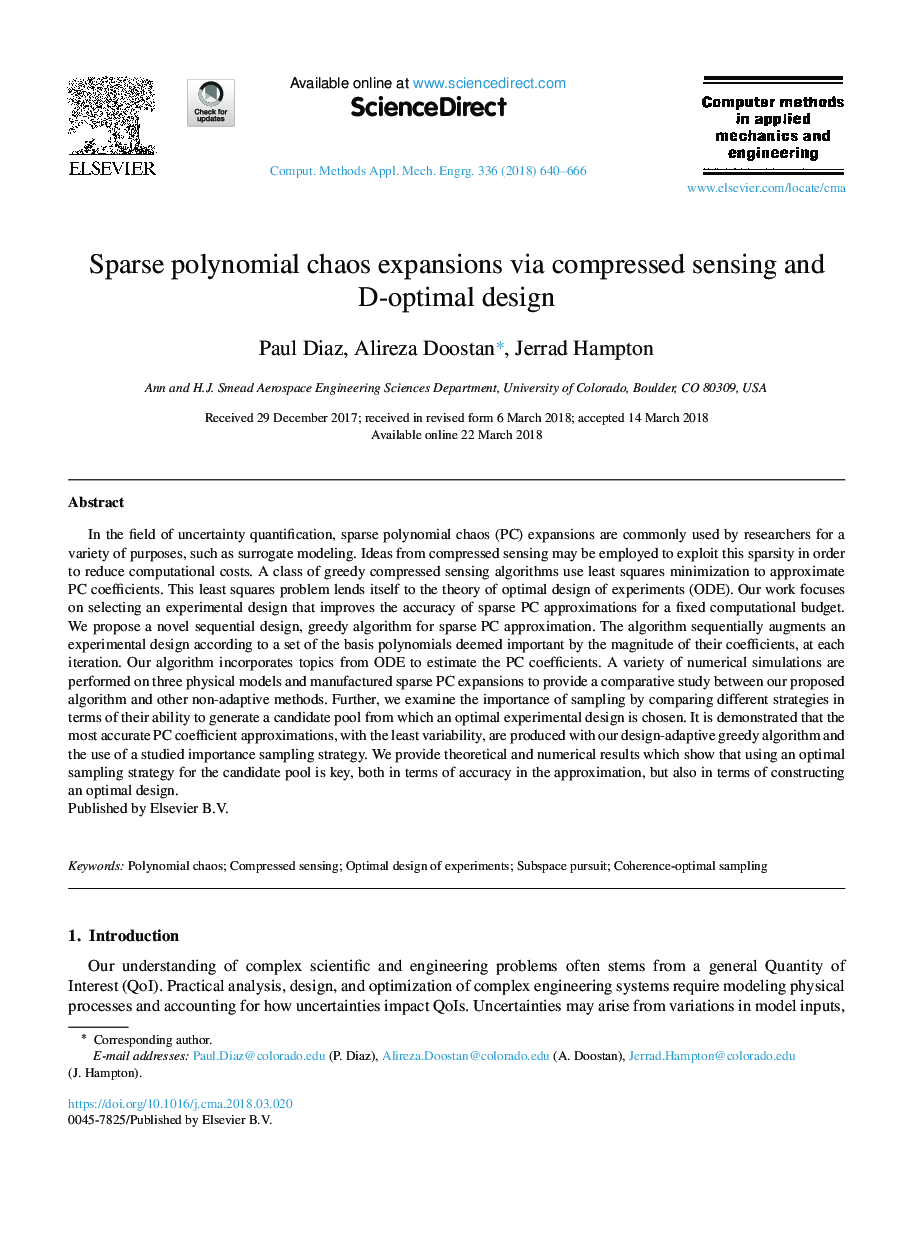
چکیده انگلیسی
In the field of uncertainty quantification, sparse polynomial chaos (PC) expansions are commonly used by researchers for a variety of purposes, such as surrogate modeling. Ideas from compressed sensing may be employed to exploit this sparsity in order to reduce computational costs. A class of greedy compressed sensing algorithms use least squares minimization to approximate PC coefficients. This least squares problem lends itself to the theory of optimal design of experiments (ODE). Our work focuses on selecting an experimental design that improves the accuracy of sparse PC approximations for a fixed computational budget. We propose a novel sequential design, greedy algorithm for sparse PC approximation. The algorithm sequentially augments an experimental design according to a set of the basis polynomials deemed important by the magnitude of their coefficients, at each iteration. Our algorithm incorporates topics from ODE to estimate the PC coefficients. A variety of numerical simulations are performed on three physical models and manufactured sparse PC expansions to provide a comparative study between our proposed algorithm and other non-adaptive methods. Further, we examine the importance of sampling by comparing different strategies in terms of their ability to generate a candidate pool from which an optimal experimental design is chosen. It is demonstrated that the most accurate PC coefficient approximations, with the least variability, are produced with our design-adaptive greedy algorithm and the use of a studied importance sampling strategy. We provide theoretical and numerical results which show that using an optimal sampling strategy for the candidate pool is key, both in terms of accuracy in the approximation, but also in terms of constructing an optimal design.
ناشر
Database: Elsevier - ScienceDirect (ساینس دایرکت)
Journal: Computer Methods in Applied Mechanics and Engineering - Volume 336, 1 July 2018, Pages 640-666
Journal: Computer Methods in Applied Mechanics and Engineering - Volume 336, 1 July 2018, Pages 640-666
نویسندگان
Paul Diaz, Alireza Doostan, Jerrad Hampton,