کد مقاله | کد نشریه | سال انتشار | مقاله انگلیسی | نسخه تمام متن |
---|---|---|---|---|
6921828 | 1448219 | 2018 | 13 صفحه PDF | دانلود رایگان |
عنوان انگلیسی مقاله ISI
Short-term traffic forecasting: An adaptive ST-KNN model that considers spatial heterogeneity
دانلود مقاله + سفارش ترجمه
دانلود مقاله ISI انگلیسی
رایگان برای ایرانیان
موضوعات مرتبط
مهندسی و علوم پایه
مهندسی کامپیوتر
نرم افزارهای علوم کامپیوتر
پیش نمایش صفحه اول مقاله
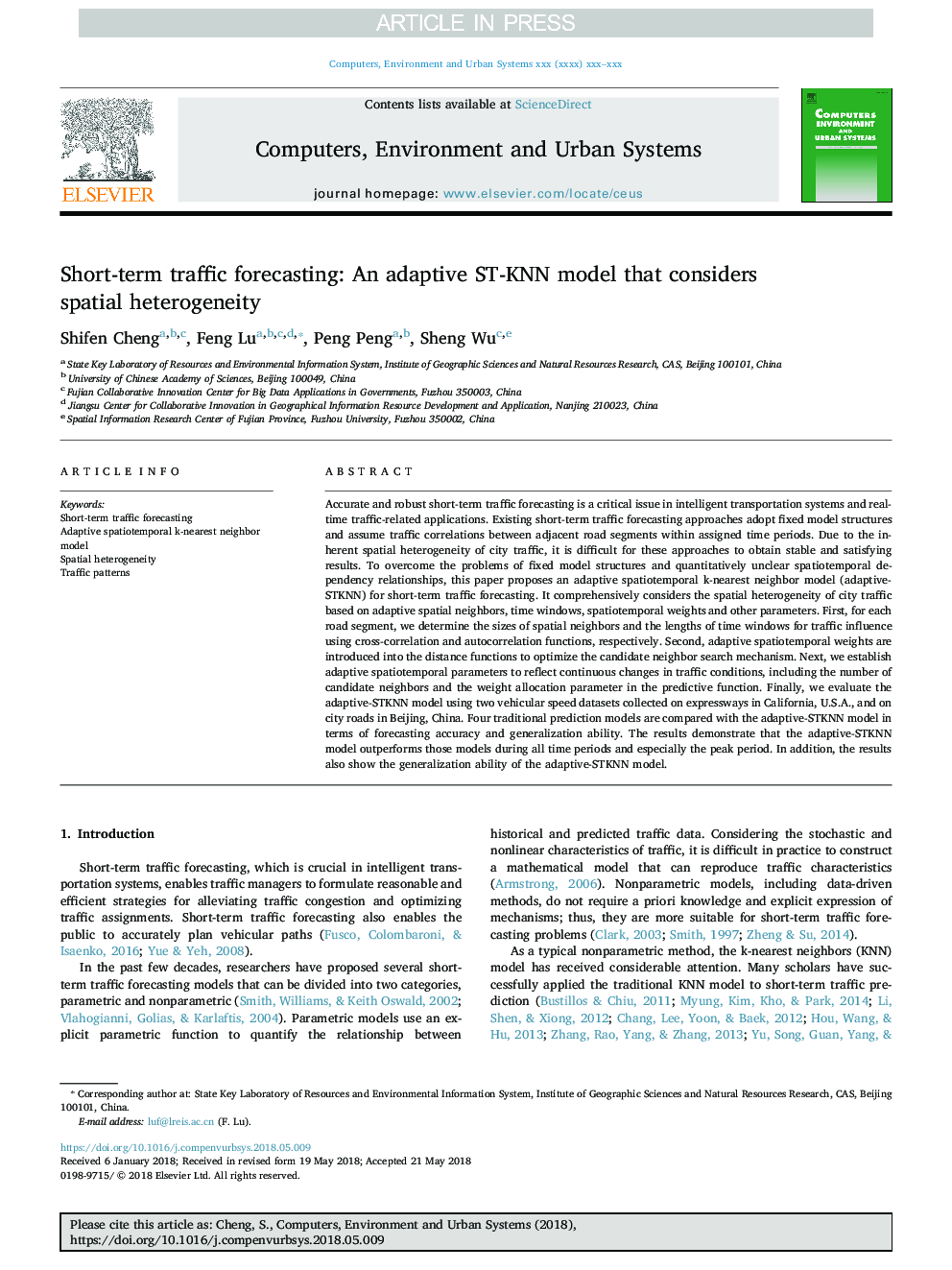
چکیده انگلیسی
Accurate and robust short-term traffic forecasting is a critical issue in intelligent transportation systems and real-time traffic-related applications. Existing short-term traffic forecasting approaches adopt fixed model structures and assume traffic correlations between adjacent road segments within assigned time periods. Due to the inherent spatial heterogeneity of city traffic, it is difficult for these approaches to obtain stable and satisfying results. To overcome the problems of fixed model structures and quantitatively unclear spatiotemporal dependency relationships, this paper proposes an adaptive spatiotemporal k-nearest neighbor model (adaptive-STKNN) for short-term traffic forecasting. It comprehensively considers the spatial heterogeneity of city traffic based on adaptive spatial neighbors, time windows, spatiotemporal weights and other parameters. First, for each road segment, we determine the sizes of spatial neighbors and the lengths of time windows for traffic influence using cross-correlation and autocorrelation functions, respectively. Second, adaptive spatiotemporal weights are introduced into the distance functions to optimize the candidate neighbor search mechanism. Next, we establish adaptive spatiotemporal parameters to reflect continuous changes in traffic conditions, including the number of candidate neighbors and the weight allocation parameter in the predictive function. Finally, we evaluate the adaptive-STKNN model using two vehicular speed datasets collected on expressways in California, U.S.A., and on city roads in Beijing, China. Four traditional prediction models are compared with the adaptive-STKNN model in terms of forecasting accuracy and generalization ability. The results demonstrate that the adaptive-STKNN model outperforms those models during all time periods and especially the peak period. In addition, the results also show the generalization ability of the adaptive-STKNN model.
ناشر
Database: Elsevier - ScienceDirect (ساینس دایرکت)
Journal: Computers, Environment and Urban Systems - Volume 71, September 2018, Pages 186-198
Journal: Computers, Environment and Urban Systems - Volume 71, September 2018, Pages 186-198
نویسندگان
Shifen Cheng, Feng Lu, Peng Peng, Sheng Wu,