کد مقاله | کد نشریه | سال انتشار | مقاله انگلیسی | نسخه تمام متن |
---|---|---|---|---|
6922271 | 1448274 | 2018 | 35 صفحه PDF | دانلود رایگان |
عنوان انگلیسی مقاله ISI
Intelligent inversion method for pre-stack seismic big data based on MapReduce
دانلود مقاله + سفارش ترجمه
دانلود مقاله ISI انگلیسی
رایگان برای ایرانیان
کلمات کلیدی
موضوعات مرتبط
مهندسی و علوم پایه
مهندسی کامپیوتر
نرم افزارهای علوم کامپیوتر
پیش نمایش صفحه اول مقاله
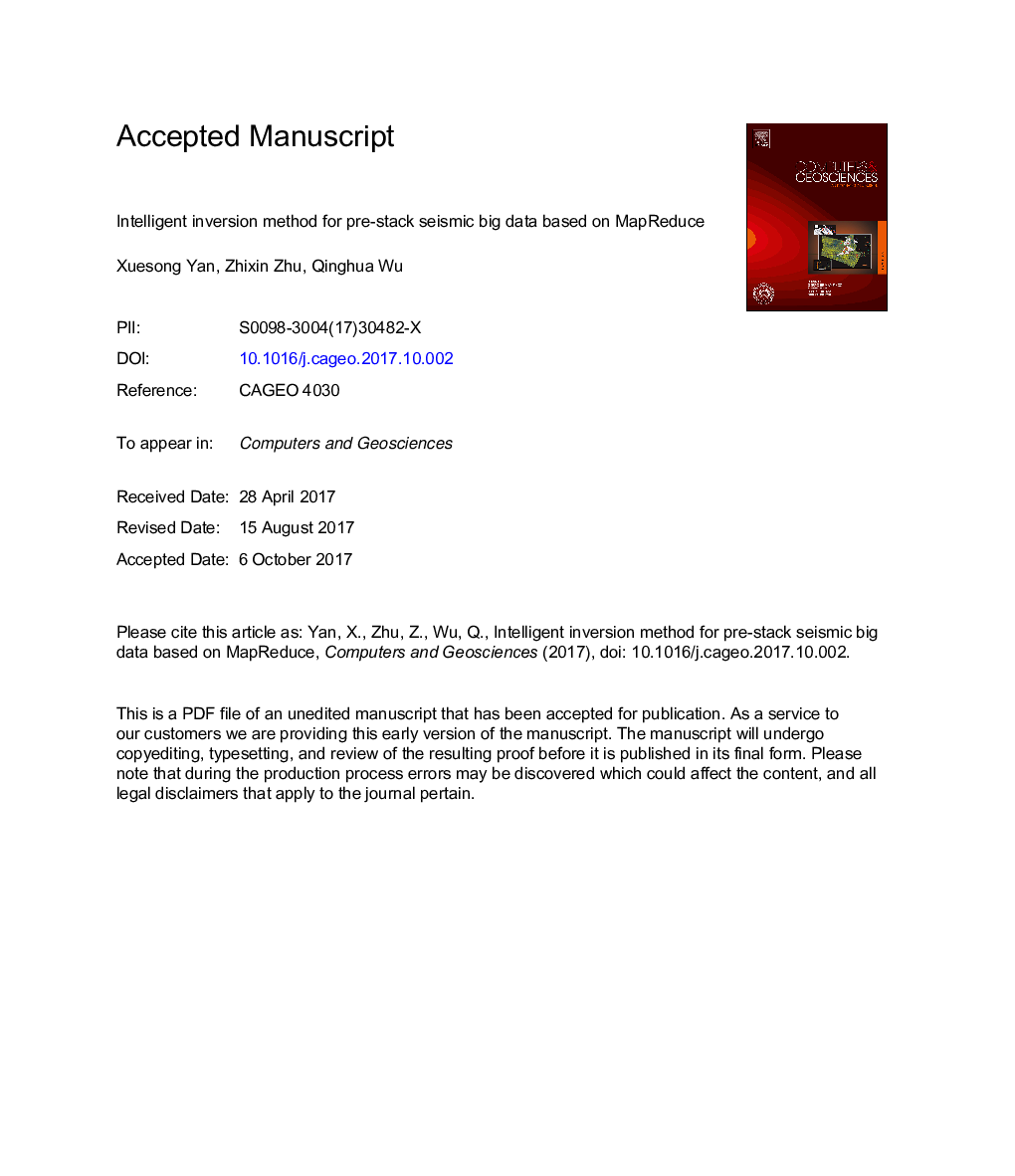
چکیده انگلیسی
Seismic exploration is a method of oil exploration that uses seismic information; that is, according to the inversion of seismic information, the useful information of the reservoir parameters can be obtained to carry out exploration effectively. Pre-stack data are characterised by a large amount of data, abundant information, and so on, and according to its inversion, the abundant information of the reservoir parameters can be obtained. Owing to the large amount of pre-stack seismic data, existing single-machine environments have not been able to meet the computational needs of the huge amount of data; thus, the development of a method with a high efficiency and the speed to solve the inversion problem of pre-stack seismic data is urgently needed. The optimisation of the elastic parameters by using a genetic algorithm easily falls into a local optimum, which results in a non-obvious inversion effect, especially for the optimisation effect of the density. Therefore, an intelligent optimisation algorithm is proposed in this paper and used for the elastic parameter inversion of pre-stack seismic data. This algorithm improves the population initialisation strategy by using the Gardner formula and the genetic operation of the algorithm, and the improved algorithm obtains better inversion results when carrying out a model test with logging data. All of the elastic parameters obtained by inversion and the logging curve of theoretical model are fitted well, which effectively improves the inversion precision of the density. This algorithm was implemented with a MapReduce model to solve the seismic big data inversion problem. The experimental results show that the parallel model can effectively reduce the running time of the algorithm.
ناشر
Database: Elsevier - ScienceDirect (ساینس دایرکت)
Journal: Computers & Geosciences - Volume 110, January 2018, Pages 81-89
Journal: Computers & Geosciences - Volume 110, January 2018, Pages 81-89
نویسندگان
Xuesong Yan, Zhixin Zhu, Qinghua Wu,