کد مقاله | کد نشریه | سال انتشار | مقاله انگلیسی | نسخه تمام متن |
---|---|---|---|---|
6922470 | 865071 | 2016 | 36 صفحه PDF | دانلود رایگان |
عنوان انگلیسی مقاله ISI
Recognition of geochemical anomalies using a deep autoencoder network
دانلود مقاله + سفارش ترجمه
دانلود مقاله ISI انگلیسی
رایگان برای ایرانیان
کلمات کلیدی
موضوعات مرتبط
مهندسی و علوم پایه
مهندسی کامپیوتر
نرم افزارهای علوم کامپیوتر
پیش نمایش صفحه اول مقاله
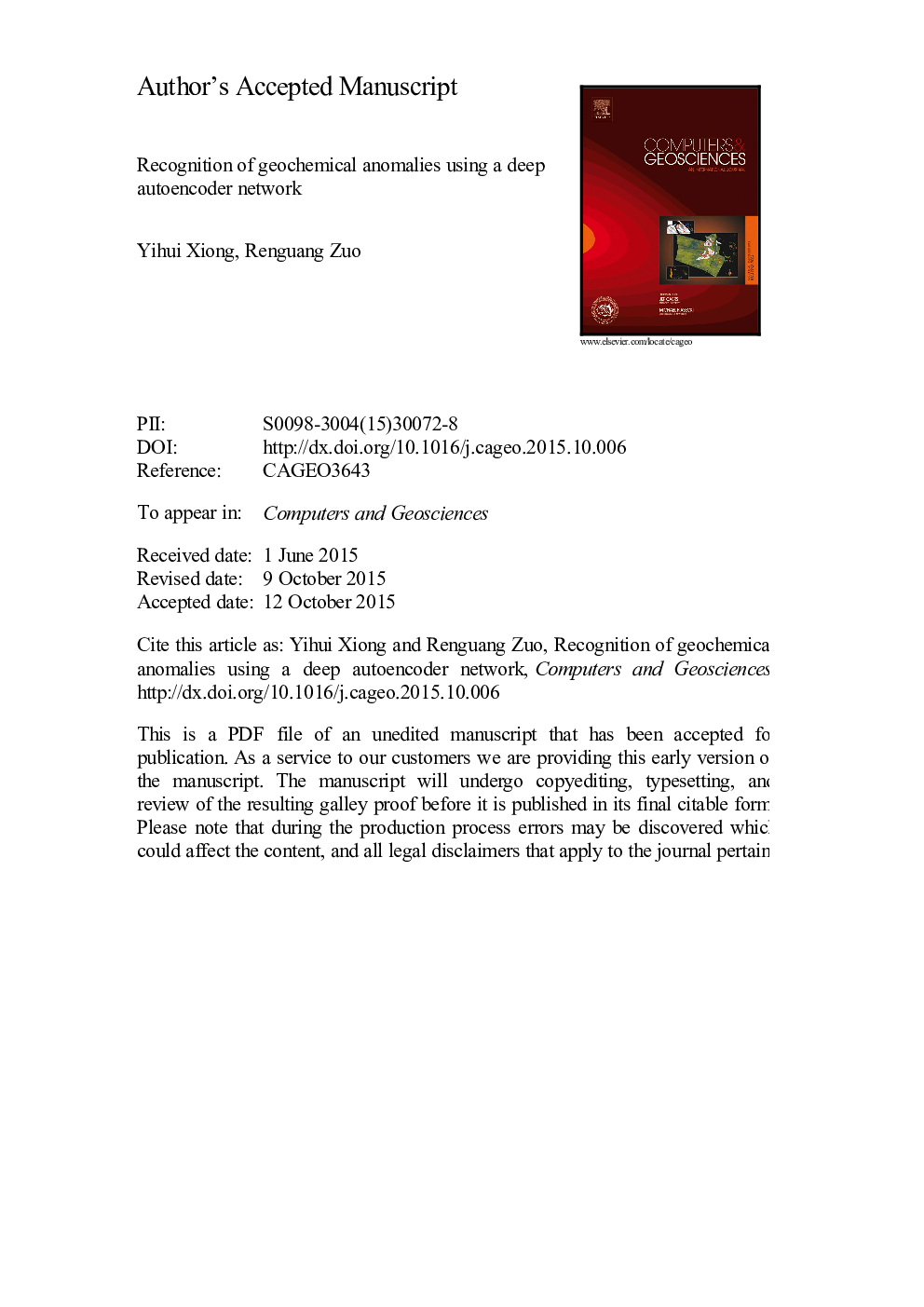
چکیده انگلیسی
In this paper, we train an autoencoder network to encode and reconstruct a geochemical sample population with unknown complex multivariate probability distributions. During the training, small probability samples contribute little to the autoencoder network. These samples can be recognized by the trained model as anomalous samples due to their comparatively higher reconstructed errors. The southwestern Fujian district (China) is chosen as a case study area. A variety of learning rates, iterations, and the size of each hidden layer are constructing and training the deep autoencoder networks on all the geochemical samples. The reconstruction error (or, anomaly score) of each training sample is used to recognize multivariate geochemical anomalies associated with Fe polymetallic mineralization. By comparing the results obtained with a continuous restricted Boltzmann machine, we conclude that the autoencoder network can be trained to recognize multivariate geochemical anomalies. Most of the known skarn-type Fe deposits are located in areas with high reconstruction errors or anomaly scores in the anomaly map, indicating that these anomalies may be related to Fe mineralization.
ناشر
Database: Elsevier - ScienceDirect (ساینس دایرکت)
Journal: Computers & Geosciences - Volume 86, January 2016, Pages 75-82
Journal: Computers & Geosciences - Volume 86, January 2016, Pages 75-82
نویسندگان
Yihui Xiong, Renguang Zuo,