کد مقاله | کد نشریه | سال انتشار | مقاله انگلیسی | نسخه تمام متن |
---|---|---|---|---|
6939110 | 1449968 | 2018 | 54 صفحه PDF | دانلود رایگان |
عنوان انگلیسی مقاله ISI
â2,1ââ1 regularized nonlinear multi-task representation learning based cognitive performance prediction of Alzheimer's disease
دانلود مقاله + سفارش ترجمه
دانلود مقاله ISI انگلیسی
رایگان برای ایرانیان
کلمات کلیدی
موضوعات مرتبط
مهندسی و علوم پایه
مهندسی کامپیوتر
چشم انداز کامپیوتر و تشخیص الگو
پیش نمایش صفحه اول مقاله
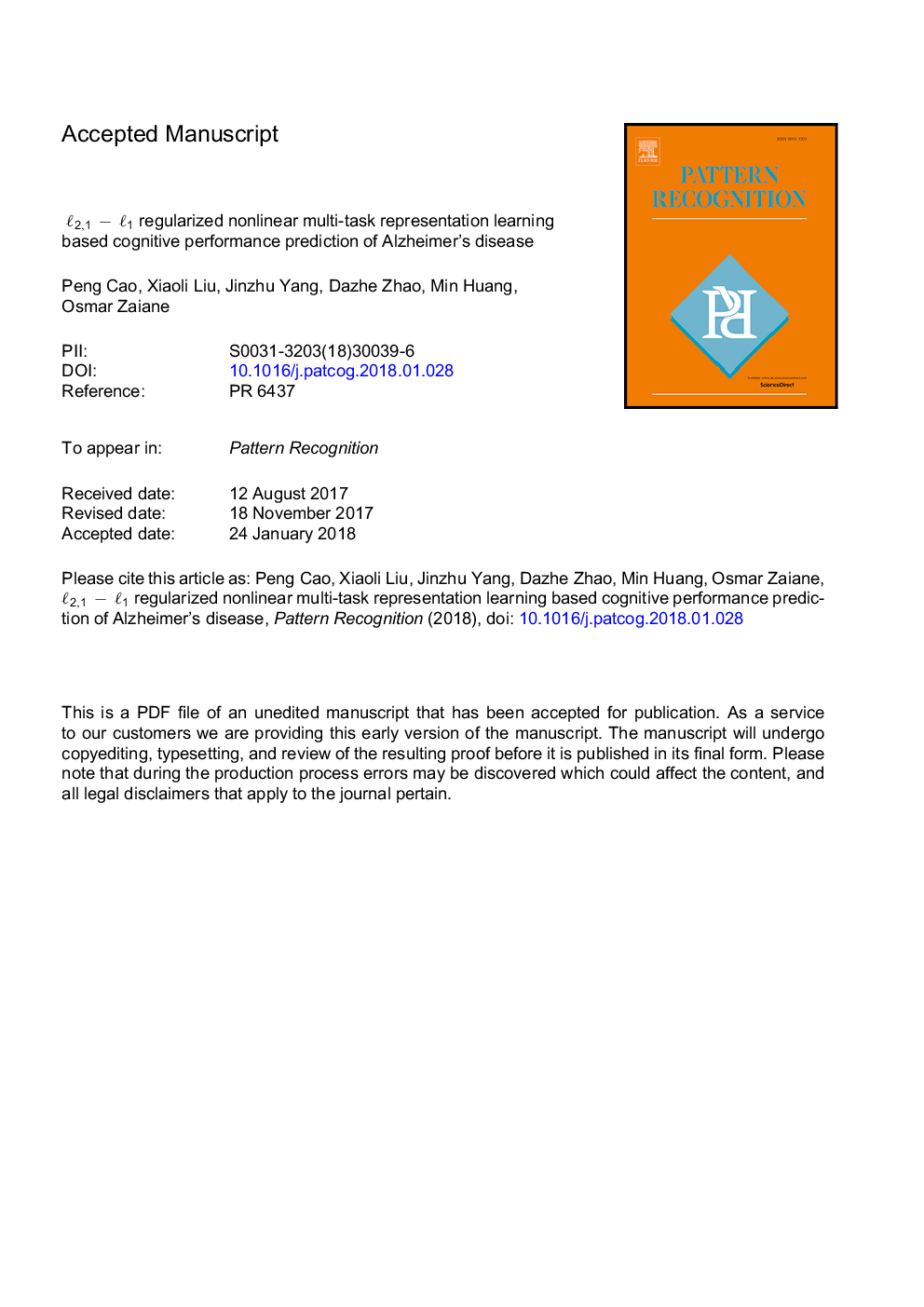
چکیده انگلیسی
Alzheimer's disease (AD) has been not only a substantial financial burden to the health care system but also the emotional hardship to patients and their families. Predicting cognitive performance of subjects from their magnetic resonance imaging (MRI) measures and identifying relevant imaging biomarkers are important research topics in the study of Alzheimer's disease. Many previous works formulate the prediction task as a linear regression problem. The most critical limitation is that they assume a linear relationship between the MRI features and the cognitive outcomes. The linear models in original MRI feature spaces can be limited by their inability to exploit the nonlinear relation between the MRI features and cognitive measure prediction tasks. To better capture the complicated but more flexible relationship between the cognitive scores and the neuroimaging measures, we propose a â2,1ââ1 norm regularized multi-kernel multi-task feature learning formulation with a joint sparsity inducing regularization. The formulation facilitates the shared kernel functions, as well as the high dimensional features in the kernel induced feature spaces simultaneously, to look for the common representation that are useful for all tasks by promoting use of few kernels and few learned features in each kernel. For optimization, we develop an alternating optimization method to effectively solve the proposed mixed norm regularized formulation. We evaluate the performance of the proposed method using the Alzheimer's Disease Neuroimaging Initiative (ADNI) datasets and demonstrate that our proposed methods achieve not only clearly improved prediction performance for cognitive measurements with single MRI modality or multi-modalities data, but also a compact set of highly suggestive biomarkers relevant to AD.
ناشر
Database: Elsevier - ScienceDirect (ساینس دایرکت)
Journal: Pattern Recognition - Volume 79, July 2018, Pages 195-215
Journal: Pattern Recognition - Volume 79, July 2018, Pages 195-215
نویسندگان
Peng Cao, Xiaoli Liu, Jinzhu Yang, Dazhe Zhao, Min Huang, Osmar Zaiane,