کد مقاله | کد نشریه | سال انتشار | مقاله انگلیسی | نسخه تمام متن |
---|---|---|---|---|
6939160 | 1449969 | 2018 | 37 صفحه PDF | دانلود رایگان |
عنوان انگلیسی مقاله ISI
SVM based multi-label learning with missing labels for image annotation
دانلود مقاله + سفارش ترجمه
دانلود مقاله ISI انگلیسی
رایگان برای ایرانیان
کلمات کلیدی
موضوعات مرتبط
مهندسی و علوم پایه
مهندسی کامپیوتر
چشم انداز کامپیوتر و تشخیص الگو
پیش نمایش صفحه اول مقاله
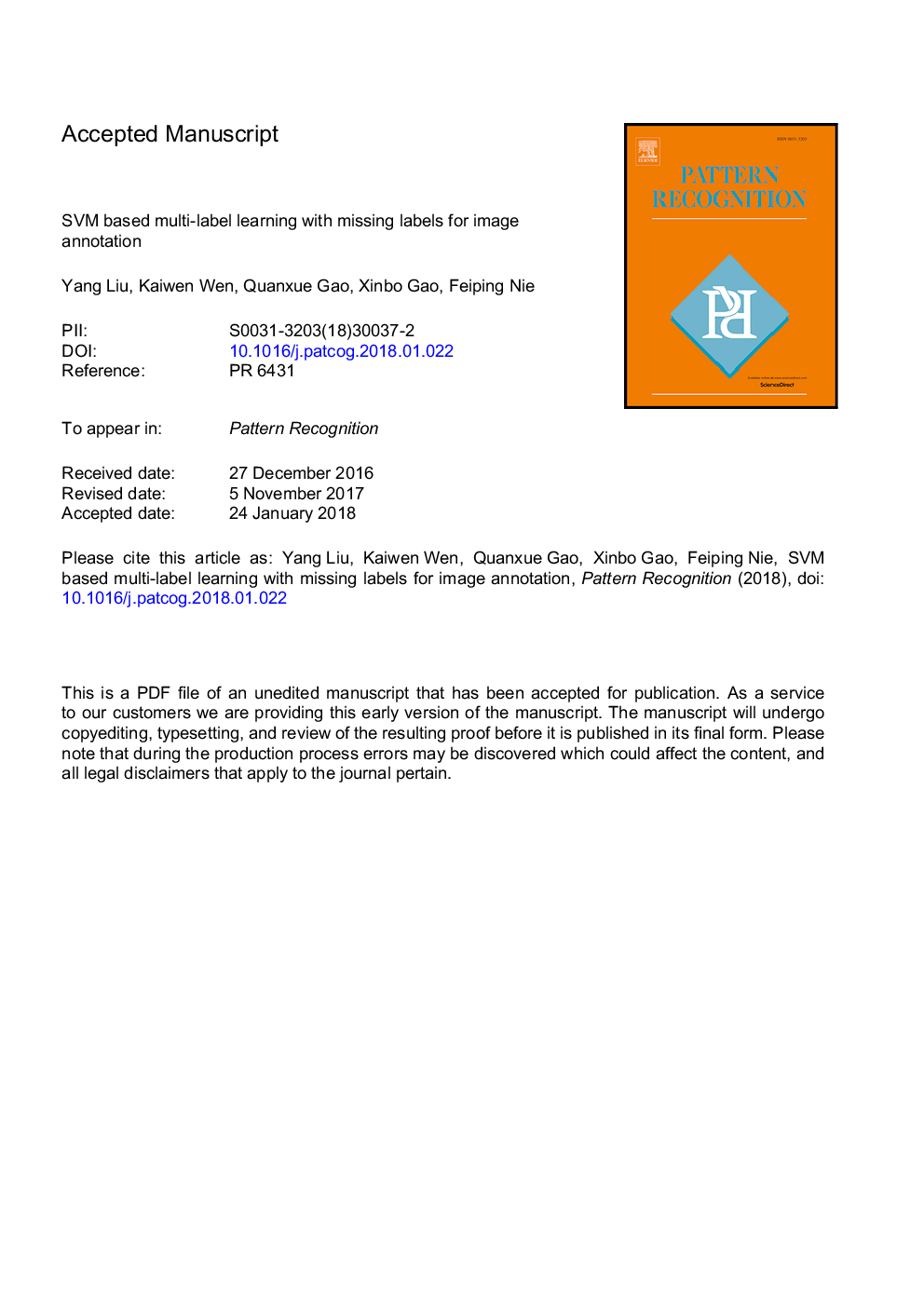
چکیده انگلیسی
Recently, multi-label learning has received much attention in the applications of image annotation and classification. However, most existing multi-label learning methods do not consider the consistency of labels, which is important in image annotation, and assume that the complete label assignment for each training image is available. In this paper, we focus on the issue of multi-label learning with missing labels, where only partial labels are available, and propose a new approach, namely SVMMN for image annotation. SVMMN integrates both example smoothness and class smoothness into the criterion function. It not only guarantees the large margin but also minimizes the number of samples that live in the large margin area. To solve SVMMN, we present an effective and efficient approximated iterative algorithm, which has good convergence. Extensive experiments on three widely used benchmark databases in image annotations illustrate that our proposed method achieves better performance than some state-of-the-art multi-label learning methods.
ناشر
Database: Elsevier - ScienceDirect (ساینس دایرکت)
Journal: Pattern Recognition - Volume 78, June 2018, Pages 307-317
Journal: Pattern Recognition - Volume 78, June 2018, Pages 307-317
نویسندگان
Yang Liu, Kaiwen Wen, Quanxue Gao, Xinbo Gao, Feiping Nie,