کد مقاله | کد نشریه | سال انتشار | مقاله انگلیسی | نسخه تمام متن |
---|---|---|---|---|
6939686 | 1449972 | 2018 | 16 صفحه PDF | دانلود رایگان |
عنوان انگلیسی مقاله ISI
Parametric local multiview hamming distance metric learning
دانلود مقاله + سفارش ترجمه
دانلود مقاله ISI انگلیسی
رایگان برای ایرانیان
موضوعات مرتبط
مهندسی و علوم پایه
مهندسی کامپیوتر
چشم انداز کامپیوتر و تشخیص الگو
پیش نمایش صفحه اول مقاله
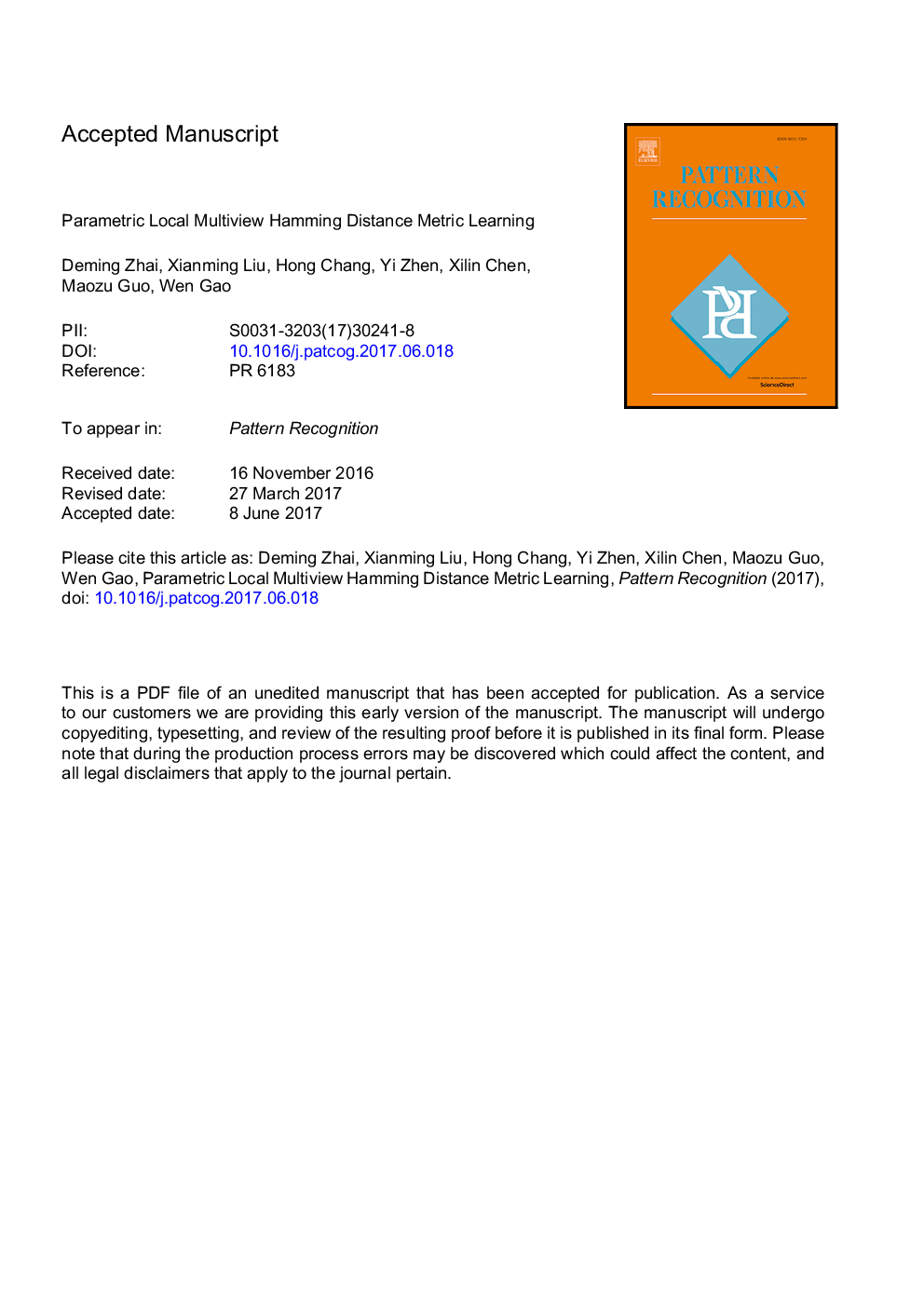
چکیده انگلیسی
Learning an appropriate distance metric is a crucial problem in pattern recognition. To confront with the scalability issue of massive data, hamming distance on binary codes is advocated since it permits exact sub-linear kNN search and meanwhile shares the advantage of efficient storage. In this paper, we study hamming metric learning in the context of multimodal data for cross-view similarity search. We present a new method called Parametric Local Multiview Hamming metric (PLMH), which learns multiview metric based on a set of local hash functions to locally adapt to the data structure of each modality. To balance locality and computational efficiency, the hash projection matrix of each instance is parameterized, with guaranteed approximation error bound, as a linear combination of basis hash projections associated with a small set of anchor points. The weak-supervisory information (side information) provided by pairwise and triplet constraints are incorporated in a coherent way to achieve semantically effective hash codes. A local optimal conjugate gradient algorithm with orthogonal rotations is designed to learn the hash functions for each bit, and the overall hash codes are learned in a sequential manner to progressively minimize the bias. Experimental evaluations on cross-media retrieval tasks demonstrate that PLMH performs competitively against the state-of-the-art methods.
ناشر
Database: Elsevier - ScienceDirect (ساینس دایرکت)
Journal: Pattern Recognition - Volume 75, March 2018, Pages 250-262
Journal: Pattern Recognition - Volume 75, March 2018, Pages 250-262
نویسندگان
Deming Zhai, Xianming Liu, Hong Chang, Yi Zhen, Xilin Chen, Maozu Guo, Wen Gao,