کد مقاله | کد نشریه | سال انتشار | مقاله انگلیسی | نسخه تمام متن |
---|---|---|---|---|
6949288 | 1451255 | 2016 | 15 صفحه PDF | دانلود رایگان |
عنوان انگلیسی مقاله ISI
Nonparametric feature extraction for classification of hyperspectral images with limited training samples
دانلود مقاله + سفارش ترجمه
دانلود مقاله ISI انگلیسی
رایگان برای ایرانیان
موضوعات مرتبط
مهندسی و علوم پایه
مهندسی کامپیوتر
سیستم های اطلاعاتی
پیش نمایش صفحه اول مقاله
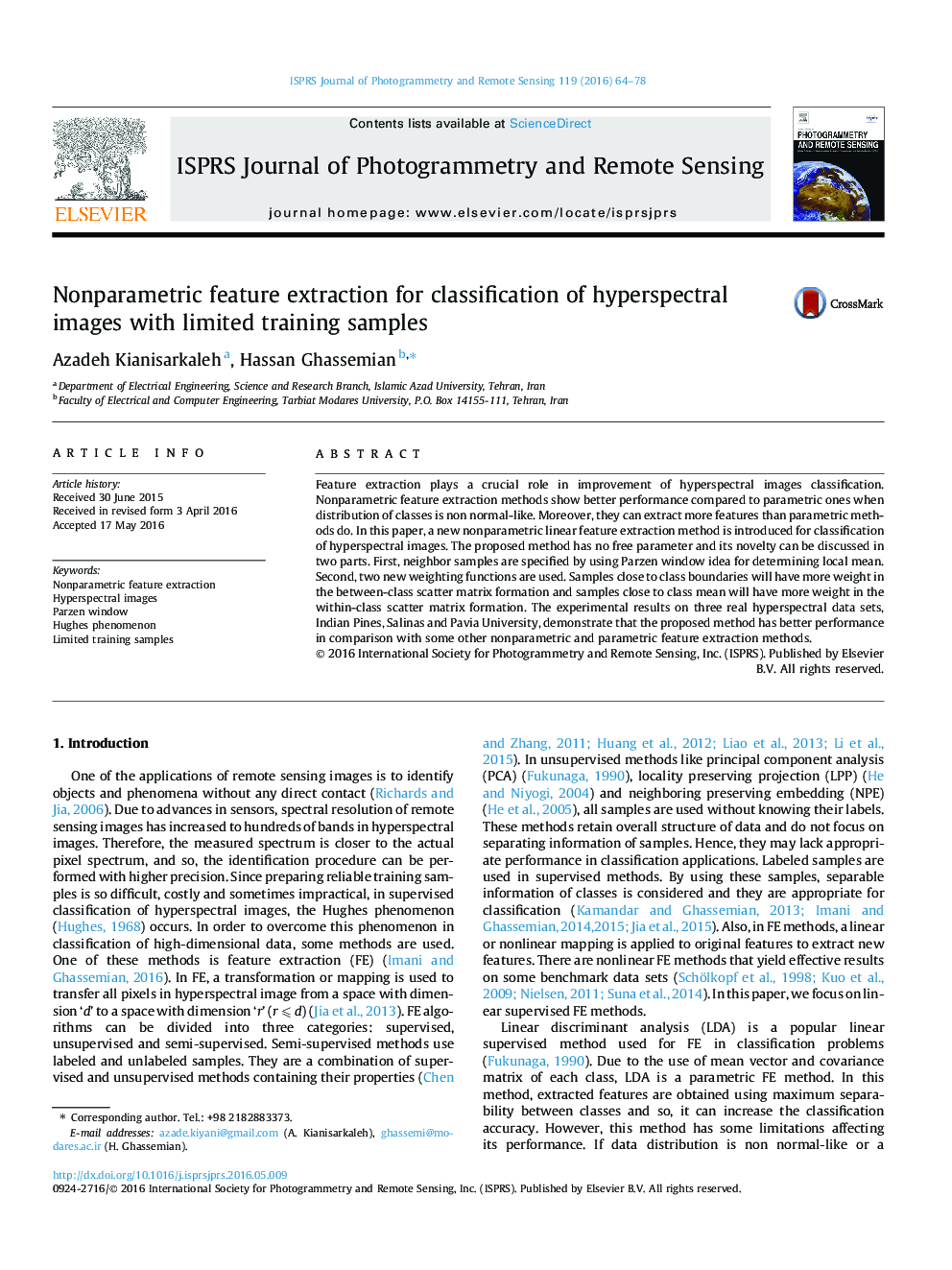
چکیده انگلیسی
Feature extraction plays a crucial role in improvement of hyperspectral images classification. Nonparametric feature extraction methods show better performance compared to parametric ones when distribution of classes is non normal-like. Moreover, they can extract more features than parametric methods do. In this paper, a new nonparametric linear feature extraction method is introduced for classification of hyperspectral images. The proposed method has no free parameter and its novelty can be discussed in two parts. First, neighbor samples are specified by using Parzen window idea for determining local mean. Second, two new weighting functions are used. Samples close to class boundaries will have more weight in the between-class scatter matrix formation and samples close to class mean will have more weight in the within-class scatter matrix formation. The experimental results on three real hyperspectral data sets, Indian Pines, Salinas and Pavia University, demonstrate that the proposed method has better performance in comparison with some other nonparametric and parametric feature extraction methods.
ناشر
Database: Elsevier - ScienceDirect (ساینس دایرکت)
Journal: ISPRS Journal of Photogrammetry and Remote Sensing - Volume 119, September 2016, Pages 64-78
Journal: ISPRS Journal of Photogrammetry and Remote Sensing - Volume 119, September 2016, Pages 64-78
نویسندگان
Azadeh Kianisarkaleh, Hassan Ghassemian,