کد مقاله | کد نشریه | سال انتشار | مقاله انگلیسی | نسخه تمام متن |
---|---|---|---|---|
6949764 | 1451287 | 2014 | 14 صفحه PDF | دانلود رایگان |
عنوان انگلیسی مقاله ISI
Optimizing multi-resolution segmentation scale using empirical methods: Exploring the sensitivity of the supervised discrepancy measure Euclidean distance 2 (ED2)
دانلود مقاله + سفارش ترجمه
دانلود مقاله ISI انگلیسی
رایگان برای ایرانیان
کلمات کلیدی
موضوعات مرتبط
مهندسی و علوم پایه
مهندسی کامپیوتر
سیستم های اطلاعاتی
پیش نمایش صفحه اول مقاله
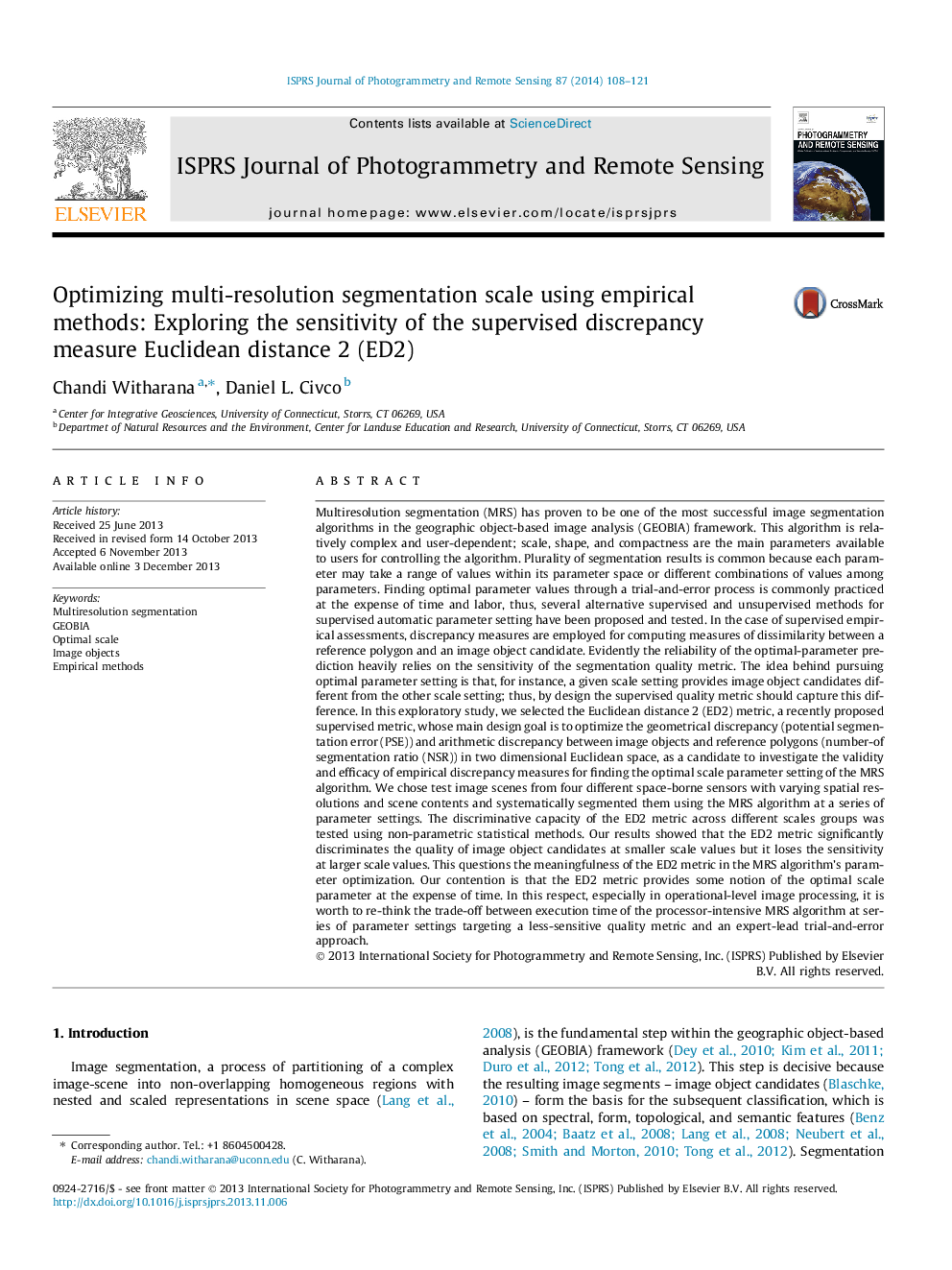
چکیده انگلیسی
Multiresolution segmentation (MRS) has proven to be one of the most successful image segmentation algorithms in the geographic object-based image analysis (GEOBIA) framework. This algorithm is relatively complex and user-dependent; scale, shape, and compactness are the main parameters available to users for controlling the algorithm. Plurality of segmentation results is common because each parameter may take a range of values within its parameter space or different combinations of values among parameters. Finding optimal parameter values through a trial-and-error process is commonly practiced at the expense of time and labor, thus, several alternative supervised and unsupervised methods for supervised automatic parameter setting have been proposed and tested. In the case of supervised empirical assessments, discrepancy measures are employed for computing measures of dissimilarity between a reference polygon and an image object candidate. Evidently the reliability of the optimal-parameter prediction heavily relies on the sensitivity of the segmentation quality metric. The idea behind pursuing optimal parameter setting is that, for instance, a given scale setting provides image object candidates different from the other scale setting; thus, by design the supervised quality metric should capture this difference. In this exploratory study, we selected the Euclidean distance 2 (ED2) metric, a recently proposed supervised metric, whose main design goal is to optimize the geometrical discrepancy (potential segmentation error (PSE)) and arithmetic discrepancy between image objects and reference polygons (number-of segmentation ratio (NSR)) in two dimensional Euclidean space, as a candidate to investigate the validity and efficacy of empirical discrepancy measures for finding the optimal scale parameter setting of the MRS algorithm. We chose test image scenes from four different space-borne sensors with varying spatial resolutions and scene contents and systematically segmented them using the MRS algorithm at a series of parameter settings. The discriminative capacity of the ED2 metric across different scales groups was tested using non-parametric statistical methods. Our results showed that the ED2 metric significantly discriminates the quality of image object candidates at smaller scale values but it loses the sensitivity at larger scale values. This questions the meaningfulness of the ED2 metric in the MRS algorithm's parameter optimization. Our contention is that the ED2 metric provides some notion of the optimal scale parameter at the expense of time. In this respect, especially in operational-level image processing, it is worth to re-think the trade-off between execution time of the processor-intensive MRS algorithm at series of parameter settings targeting a less-sensitive quality metric and an expert-lead trial-and-error approach.
ناشر
Database: Elsevier - ScienceDirect (ساینس دایرکت)
Journal: ISPRS Journal of Photogrammetry and Remote Sensing - Volume 87, January 2014, Pages 108-121
Journal: ISPRS Journal of Photogrammetry and Remote Sensing - Volume 87, January 2014, Pages 108-121
نویسندگان
Chandi Witharana, Daniel L. Civco,