کد مقاله | کد نشریه | سال انتشار | مقاله انگلیسی | نسخه تمام متن |
---|---|---|---|---|
6951584 | 1451693 | 2015 | 19 صفحه PDF | دانلود رایگان |
عنوان انگلیسی مقاله ISI
Relevance factor of maximum a posteriori adaptation for GMM-NAP-SVM in speaker and language recognition
دانلود مقاله + سفارش ترجمه
دانلود مقاله ISI انگلیسی
رایگان برای ایرانیان
کلمات کلیدی
موضوعات مرتبط
مهندسی و علوم پایه
مهندسی کامپیوتر
پردازش سیگنال
پیش نمایش صفحه اول مقاله
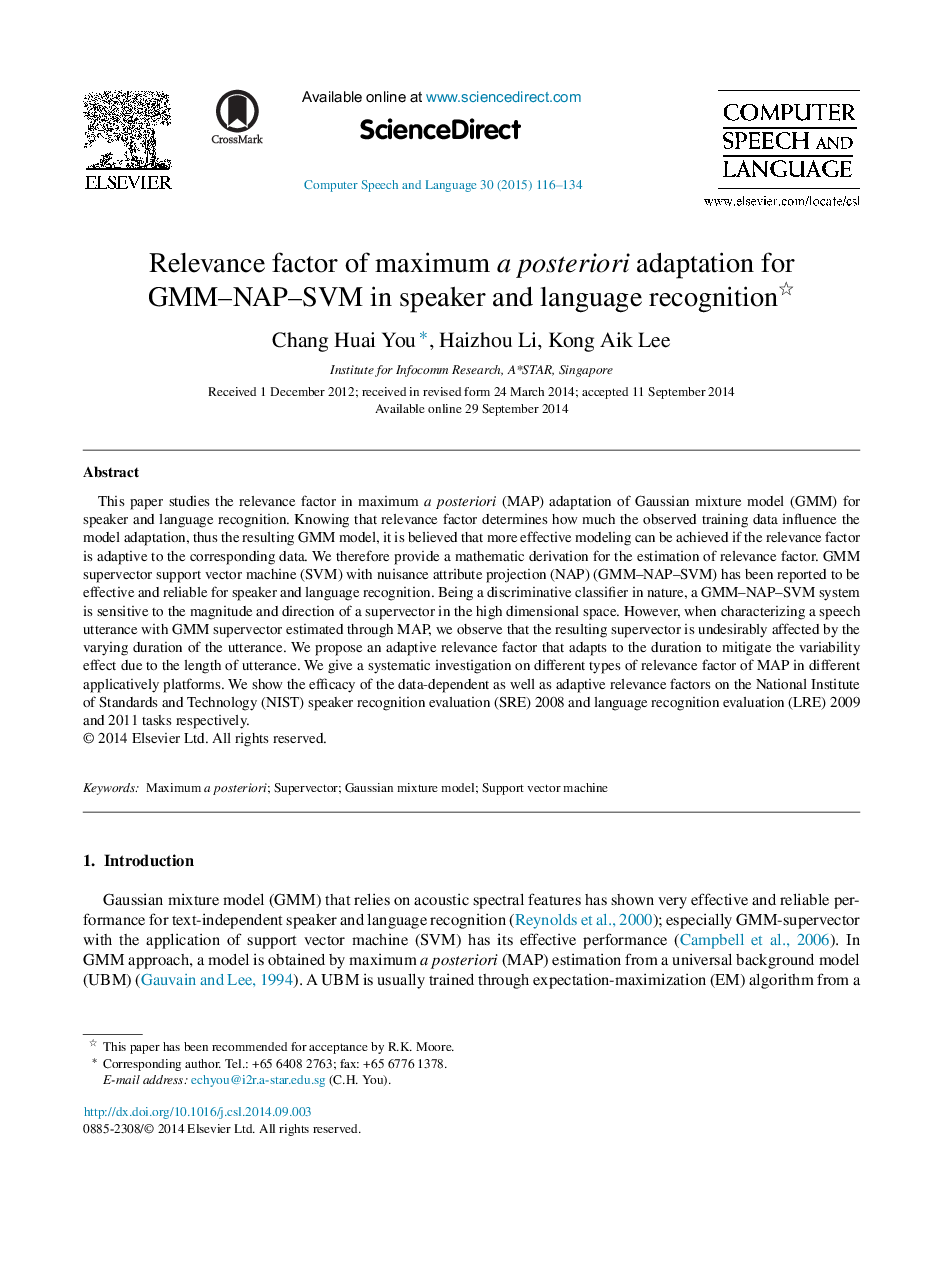
چکیده انگلیسی
This paper studies the relevance factor in maximum a posteriori (MAP) adaptation of Gaussian mixture model (GMM) for speaker and language recognition. Knowing that relevance factor determines how much the observed training data influence the model adaptation, thus the resulting GMM model, it is believed that more effective modeling can be achieved if the relevance factor is adaptive to the corresponding data. We therefore provide a mathematic derivation for the estimation of relevance factor. GMM supervector support vector machine (SVM) with nuisance attribute projection (NAP) (GMM-NAP-SVM) has been reported to be effective and reliable for speaker and language recognition. Being a discriminative classifier in nature, a GMM-NAP-SVM system is sensitive to the magnitude and direction of a supervector in the high dimensional space. However, when characterizing a speech utterance with GMM supervector estimated through MAP, we observe that the resulting supervector is undesirably affected by the varying duration of the utterance. We propose an adaptive relevance factor that adapts to the duration to mitigate the variability effect due to the length of utterance. We give a systematic investigation on different types of relevance factor of MAP in different applicatively platforms. We show the efficacy of the data-dependent as well as adaptive relevance factors on the National Institute of Standards and Technology (NIST) speaker recognition evaluation (SRE) 2008 and language recognition evaluation (LRE) 2009 and 2011 tasks respectively.
ناشر
Database: Elsevier - ScienceDirect (ساینس دایرکت)
Journal: Computer Speech & Language - Volume 30, Issue 1, March 2015, Pages 116-134
Journal: Computer Speech & Language - Volume 30, Issue 1, March 2015, Pages 116-134
نویسندگان
Chang Huai You, Haizhou Li, Kong Aik Lee,