کد مقاله | کد نشریه | سال انتشار | مقاله انگلیسی | نسخه تمام متن |
---|---|---|---|---|
6957246 | 1451915 | 2018 | 11 صفحه PDF | دانلود رایگان |
عنوان انگلیسی مقاله ISI
Model-driven online parameter adjustment for zero-attracting LMS
دانلود مقاله + سفارش ترجمه
دانلود مقاله ISI انگلیسی
رایگان برای ایرانیان
موضوعات مرتبط
مهندسی و علوم پایه
مهندسی کامپیوتر
پردازش سیگنال
پیش نمایش صفحه اول مقاله
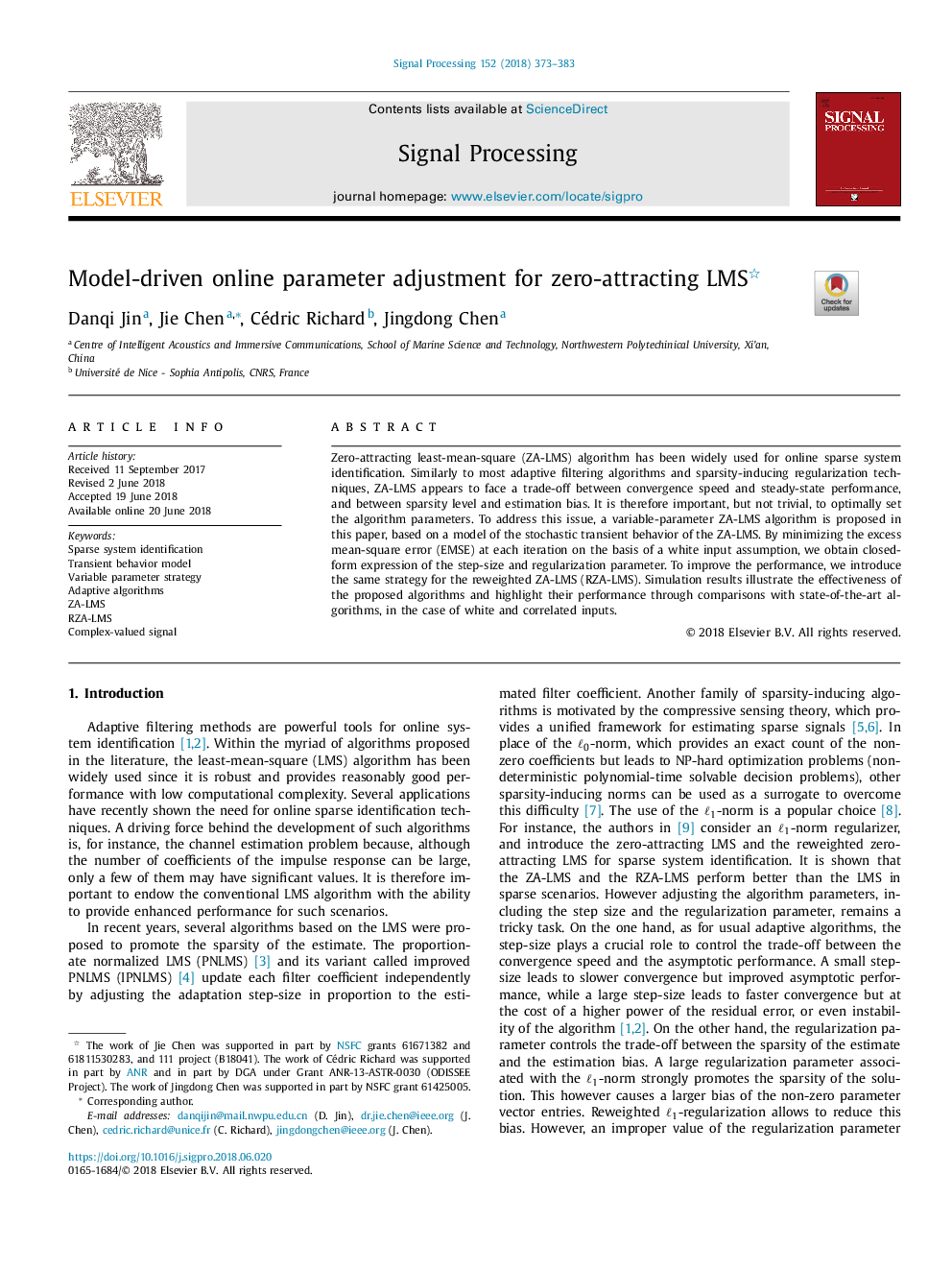
چکیده انگلیسی
Zero-attracting least-mean-square (ZA-LMS) algorithm has been widely used for online sparse system identification. Similarly to most adaptive filtering algorithms and sparsity-inducing regularization techniques, ZA-LMS appears to face a trade-off between convergence speed and steady-state performance, and between sparsity level and estimation bias. It is therefore important, but not trivial, to optimally set the algorithm parameters. To address this issue, a variable-parameter ZA-LMS algorithm is proposed in this paper, based on a model of the stochastic transient behavior of the ZA-LMS. By minimizing the excess mean-square error (EMSE) at each iteration on the basis of a white input assumption, we obtain closed-form expression of the step-size and regularization parameter. To improve the performance, we introduce the same strategy for the reweighted ZA-LMS (RZA-LMS). Simulation results illustrate the effectiveness of the proposed algorithms and highlight their performance through comparisons with state-of-the-art algorithms, in the case of white and correlated inputs.
ناشر
Database: Elsevier - ScienceDirect (ساینس دایرکت)
Journal: Signal Processing - Volume 152, November 2018, Pages 373-383
Journal: Signal Processing - Volume 152, November 2018, Pages 373-383
نویسندگان
Danqi Jin, Jie Chen, Cédric Richard, Jingdong Chen,