کد مقاله | کد نشریه | سال انتشار | مقاله انگلیسی | نسخه تمام متن |
---|---|---|---|---|
6957796 | 1451922 | 2018 | 10 صفحه PDF | دانلود رایگان |
عنوان انگلیسی مقاله ISI
Cramer-Rao type bounds for sparsity-aware multi-sensor multi-target tracking
دانلود مقاله + سفارش ترجمه
دانلود مقاله ISI انگلیسی
رایگان برای ایرانیان
کلمات کلیدی
موضوعات مرتبط
مهندسی و علوم پایه
مهندسی کامپیوتر
پردازش سیگنال
پیش نمایش صفحه اول مقاله
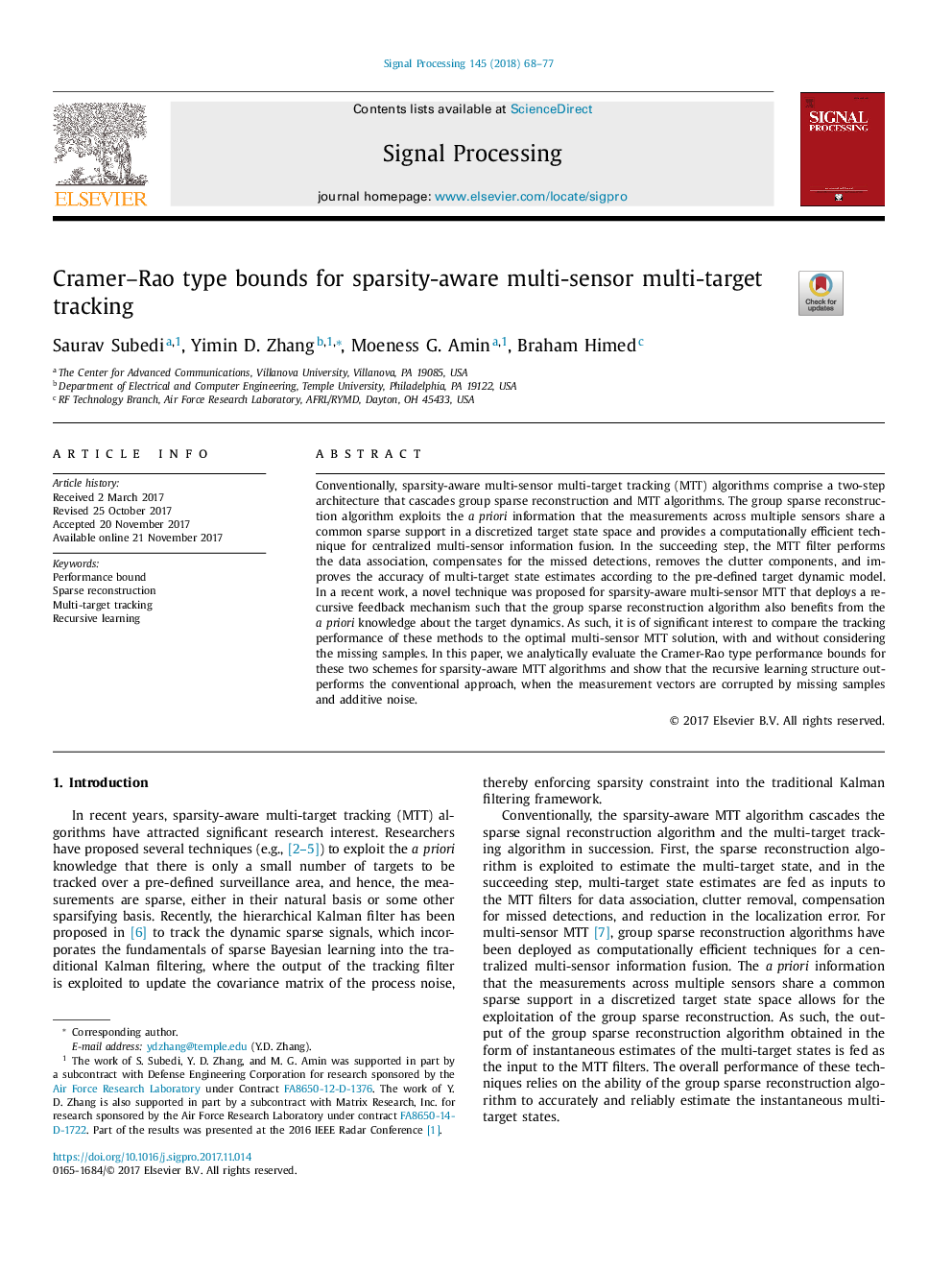
چکیده انگلیسی
Conventionally, sparsity-aware multi-sensor multi-target tracking (MTT) algorithms comprise a two-step architecture that cascades group sparse reconstruction and MTT algorithms. The group sparse reconstruction algorithm exploits the apriori information that the measurements across multiple sensors share a common sparse support in a discretized target state space and provides a computationally efficient technique for centralized multi-sensor information fusion. In the succeeding step, the MTT filter performs the data association, compensates for the missed detections, removes the clutter components, and improves the accuracy of multi-target state estimates according to the pre-defined target dynamic model. In a recent work, a novel technique was proposed for sparsity-aware multi-sensor MTT that deploys a recursive feedback mechanism such that the group sparse reconstruction algorithm also benefits from the apriori knowledge about the target dynamics. As such, it is of significant interest to compare the tracking performance of these methods to the optimal multi-sensor MTT solution, with and without considering the missing samples. In this paper, we analytically evaluate the Cramer-Rao type performance bounds for these two schemes for sparsity-aware MTT algorithms and show that the recursive learning structure outperforms the conventional approach, when the measurement vectors are corrupted by missing samples and additive noise.
ناشر
Database: Elsevier - ScienceDirect (ساینس دایرکت)
Journal: Signal Processing - Volume 145, April 2018, Pages 68-77
Journal: Signal Processing - Volume 145, April 2018, Pages 68-77
نویسندگان
Saurav Subedi, Yimin D. Zhang, Moeness G. Amin, Braham Himed,