کد مقاله | کد نشریه | سال انتشار | مقاله انگلیسی | نسخه تمام متن |
---|---|---|---|---|
6958233 | 1451938 | 2016 | 22 صفحه PDF | دانلود رایگان |
عنوان انگلیسی مقاله ISI
Improved DOA estimation based on real-valued array covariance using sparse Bayesian learning
دانلود مقاله + سفارش ترجمه
دانلود مقاله ISI انگلیسی
رایگان برای ایرانیان
کلمات کلیدی
موضوعات مرتبط
مهندسی و علوم پایه
مهندسی کامپیوتر
پردازش سیگنال
پیش نمایش صفحه اول مقاله
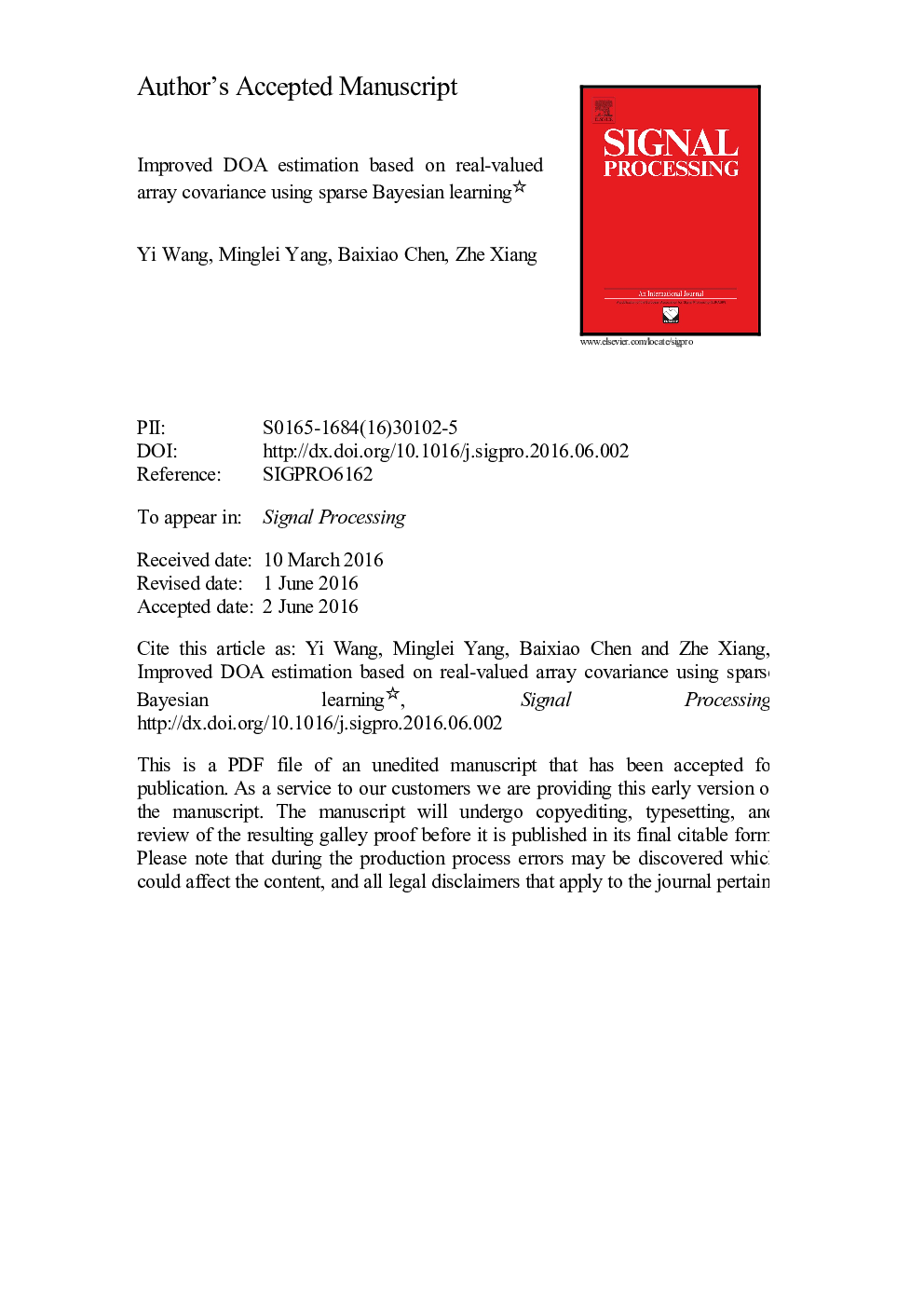
چکیده انگلیسی
To further improve the efficiency of sparse Bayesian learning (SBL) for direction of arrival (DOA) estimation, a real-valued (unitary) formulation of covariance vector-based relevance vector machine (CV-RVM) technique is proposed in this paper. The covariance matrix of the sensor output is firstly transformed into a real-valued covariance matrix via unitary transformation, and the real-valued covariance matrix can be sparsely represented in a real-valued over-complete dictionary. Then the sparse Bayesian learning technique implemented in real domain is used to estimate the DOA. According to the property of the real-valued covariance matrix, unitary single measurement vector (USMV) CV-RVM for uncorrelated signals and unitary multiple measurement vector (UMMV) CV-RVM for correlated signals are developed, respectively. Due to the fact that the proposed methods are implemented in real domain and the snapshots are doubled via unitary transformation, the proposed methods have lower computational cost and better performance compared to the original SMV CV-RVM and MMV CV-RVM. Simulation results show the effectiveness of the proposed methods.
ناشر
Database: Elsevier - ScienceDirect (ساینس دایرکت)
Journal: Signal Processing - Volume 129, December 2016, Pages 183-189
Journal: Signal Processing - Volume 129, December 2016, Pages 183-189
نویسندگان
Yi Wang, Minglei Yang, Baixiao Chen, Zhe Xiang,