کد مقاله | کد نشریه | سال انتشار | مقاله انگلیسی | نسخه تمام متن |
---|---|---|---|---|
712833 | 892158 | 2006 | 6 صفحه PDF | دانلود رایگان |
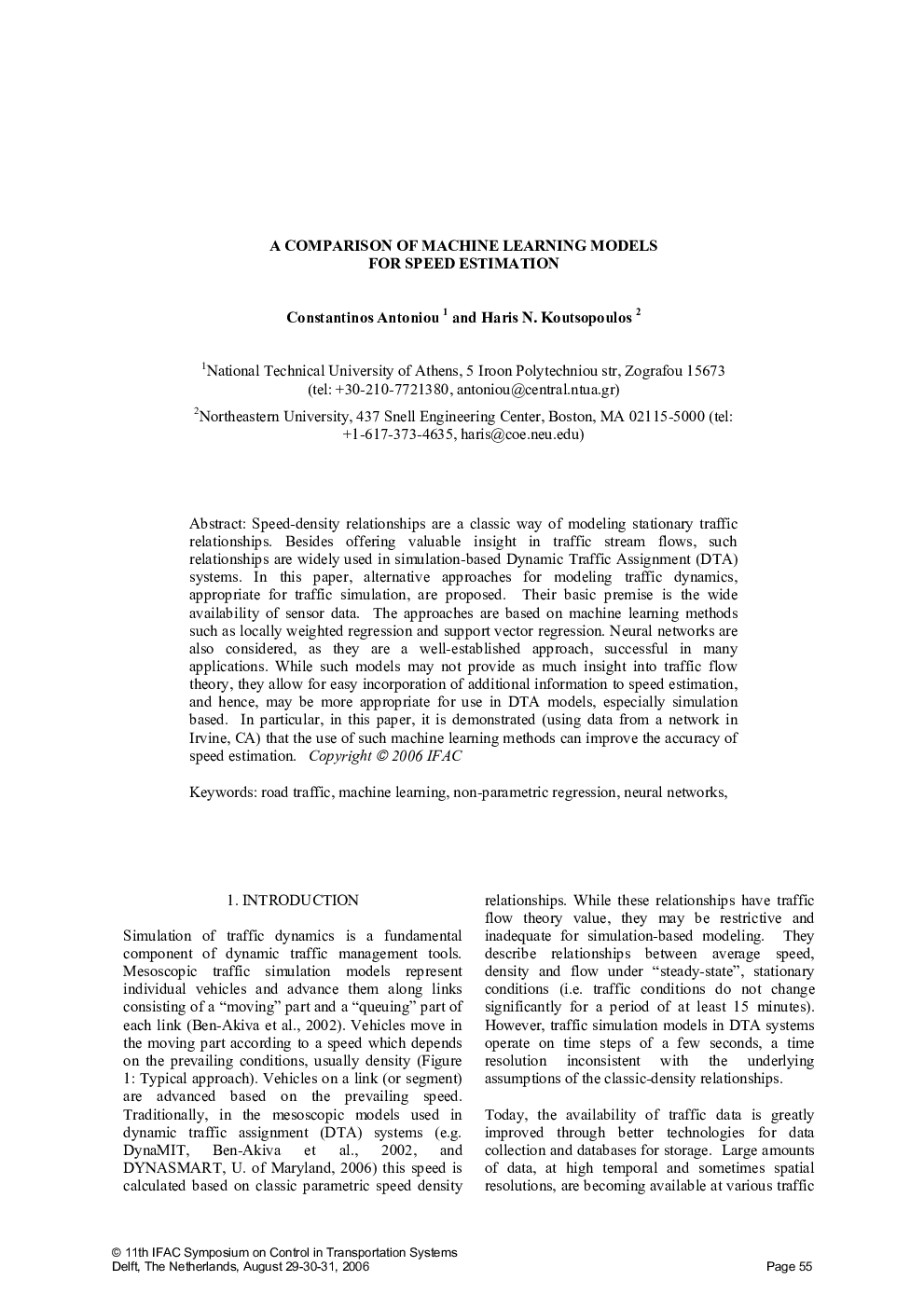
Speed-density relationships are a classic way of modeling stationary traffic relationships. Besides offering valuable insight in traffic stream flows, such relationships are widely used in simulation-based Dynamic Traffic Assignment (DTA) systems. In this paper, alternative approaches for modeling traffic dynamics, appropriate for traffic simulation, are proposed. Their basic premise is the wide availability of sensor data. The approaches are based on machine learning methods such as locally weighted regression and support vector regression. Neural networks are also considered, as they are a well-established approach, successful in many applications. While such models may not provide as much insight into traffic flow theory, they allow for easy incorporation of additional information to speed estimation, and hence, may be more appropriate for use in DTA models, especially simulation based. In particular, in this paper, it is demonstrated (using data from a network in Irvine, CA) that the use of such machine learning methods can improve the accuracy of speed estimation.
Journal: IFAC Proceedings Volumes - Volume 39, Issue 12, January 2006, Pages 55–60