کد مقاله | کد نشریه | سال انتشار | مقاله انگلیسی | نسخه تمام متن |
---|---|---|---|---|
740130 | 1462097 | 2014 | 7 صفحه PDF | دانلود رایگان |
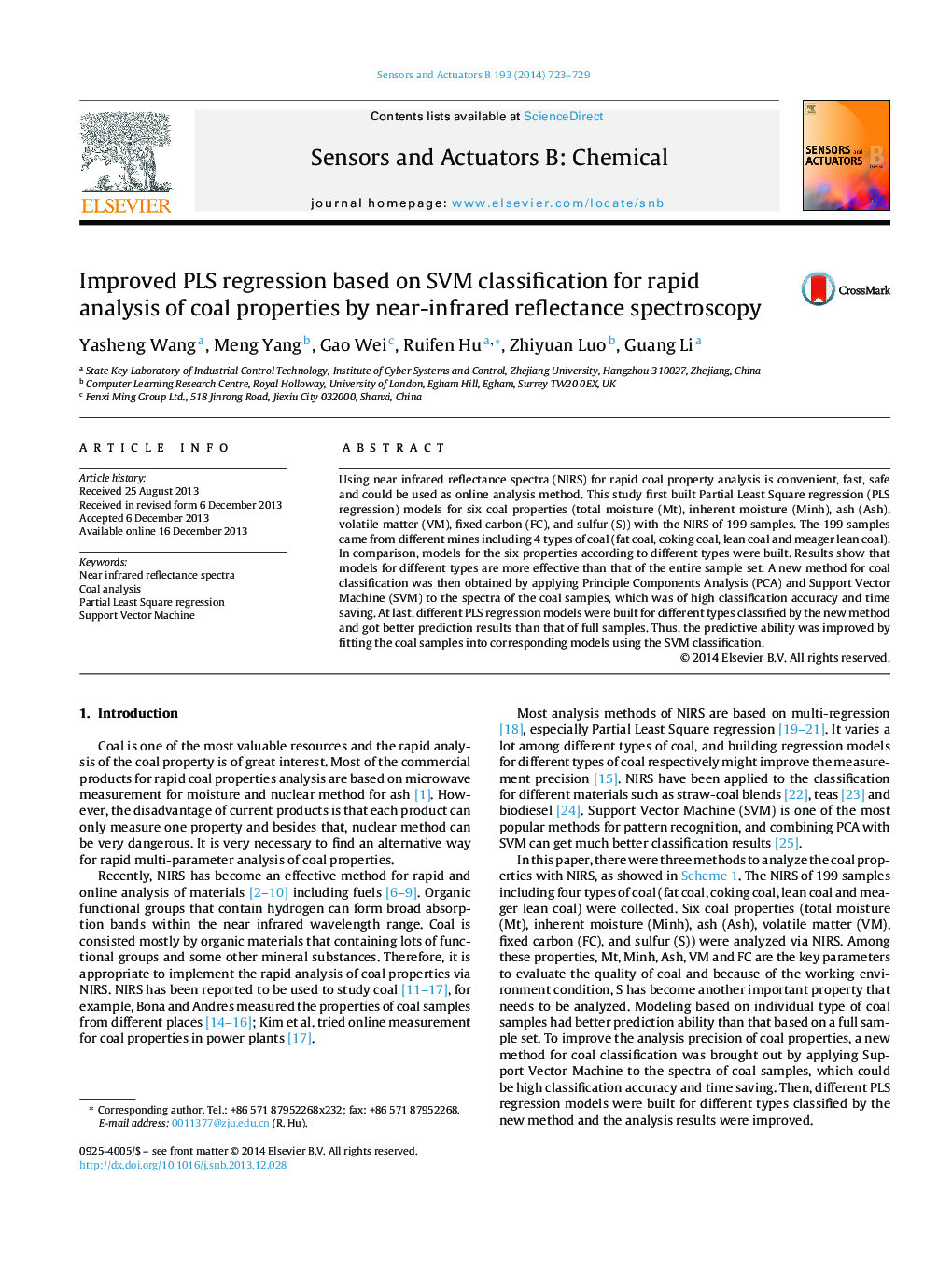
• PLS regression models based on NIRS were built for the rapid analysis of coal properties.
• Models for different kind of coal samples show better result than models for the whole sample set.
• A method for the coal classification was obtained by applying PCA and SVM to the NIRS of coal samples.
• Different regression models for different categories classified by the SVM method get better prediction results.
Using near infrared reflectance spectra (NIRS) for rapid coal property analysis is convenient, fast, safe and could be used as online analysis method. This study first built Partial Least Square regression (PLS regression) models for six coal properties (total moisture (Mt), inherent moisture (Minh), ash (Ash), volatile matter (VM), fixed carbon (FC), and sulfur (S)) with the NIRS of 199 samples. The 199 samples came from different mines including 4 types of coal (fat coal, coking coal, lean coal and meager lean coal). In comparison, models for the six properties according to different types were built. Results show that models for different types are more effective than that of the entire sample set. A new method for coal classification was then obtained by applying Principle Components Analysis (PCA) and Support Vector Machine (SVM) to the spectra of the coal samples, which was of high classification accuracy and time saving. At last, different PLS regression models were built for different types classified by the new method and got better prediction results than that of full samples. Thus, the predictive ability was improved by fitting the coal samples into corresponding models using the SVM classification.
Journal: Sensors and Actuators B: Chemical - Volume 193, 31 March 2014, Pages 723–729