کد مقاله | کد نشریه | سال انتشار | مقاله انگلیسی | نسخه تمام متن |
---|---|---|---|---|
753544 | 895547 | 2013 | 11 صفحه PDF | دانلود رایگان |
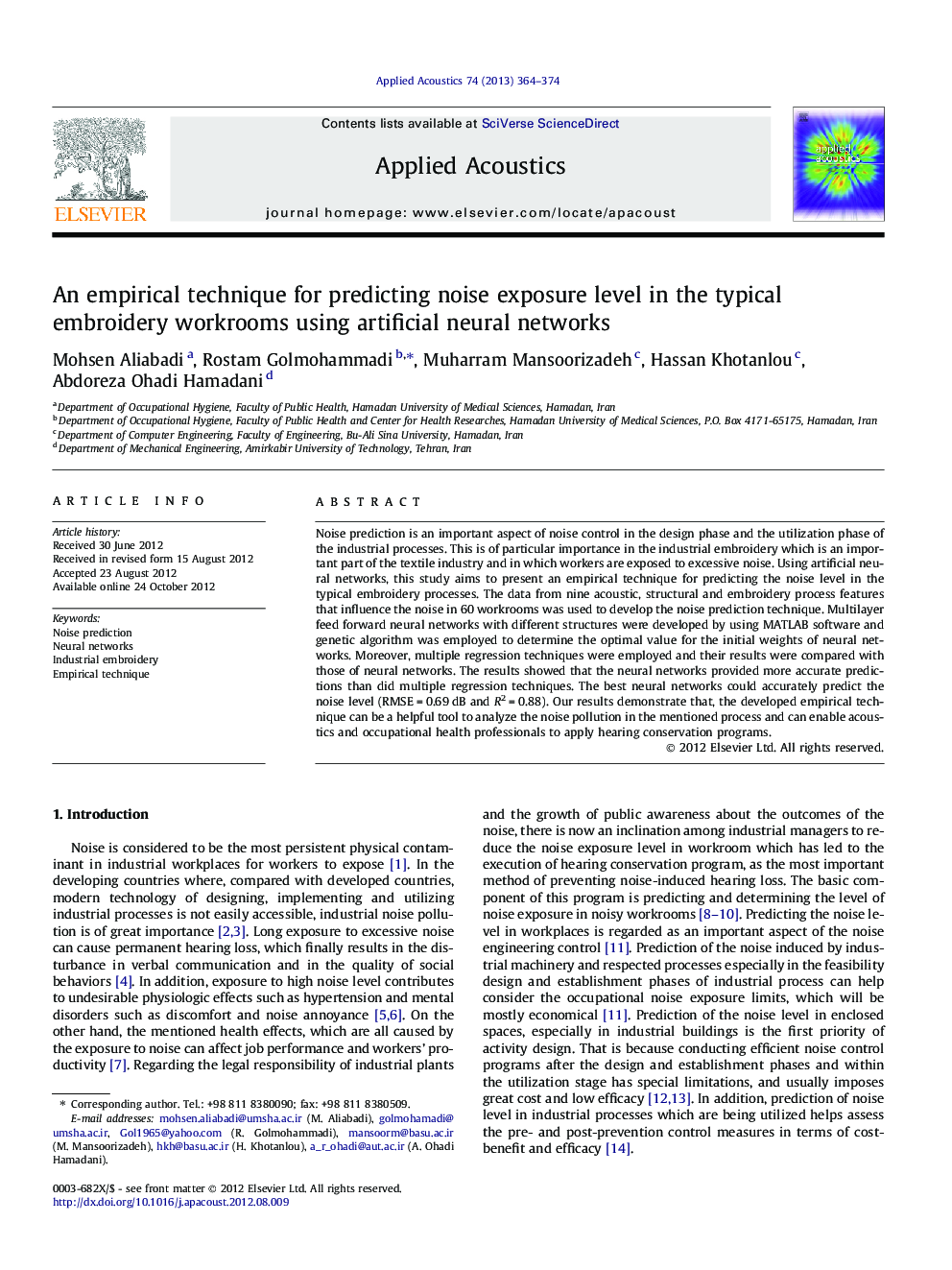
Noise prediction is an important aspect of noise control in the design phase and the utilization phase of the industrial processes. This is of particular importance in the industrial embroidery which is an important part of the textile industry and in which workers are exposed to excessive noise. Using artificial neural networks, this study aims to present an empirical technique for predicting the noise level in the typical embroidery processes. The data from nine acoustic, structural and embroidery process features that influence the noise in 60 workrooms was used to develop the noise prediction technique. Multilayer feed forward neural networks with different structures were developed by using MATLAB software and genetic algorithm was employed to determine the optimal value for the initial weights of neural networks. Moreover, multiple regression techniques were employed and their results were compared with those of neural networks. The results showed that the neural networks provided more accurate predictions than did multiple regression techniques. The best neural networks could accurately predict the noise level (RMSE = 0.69 dB and R2 = 0.88). Our results demonstrate that, the developed empirical technique can be a helpful tool to analyze the noise pollution in the mentioned process and can enable acoustics and occupational health professionals to apply hearing conservation programs.
Journal: Applied Acoustics - Volume 74, Issue 3, March 2013, Pages 364–374