کد مقاله | کد نشریه | سال انتشار | مقاله انگلیسی | نسخه تمام متن |
---|---|---|---|---|
786887 | 1466417 | 2014 | 13 صفحه PDF | دانلود رایگان |
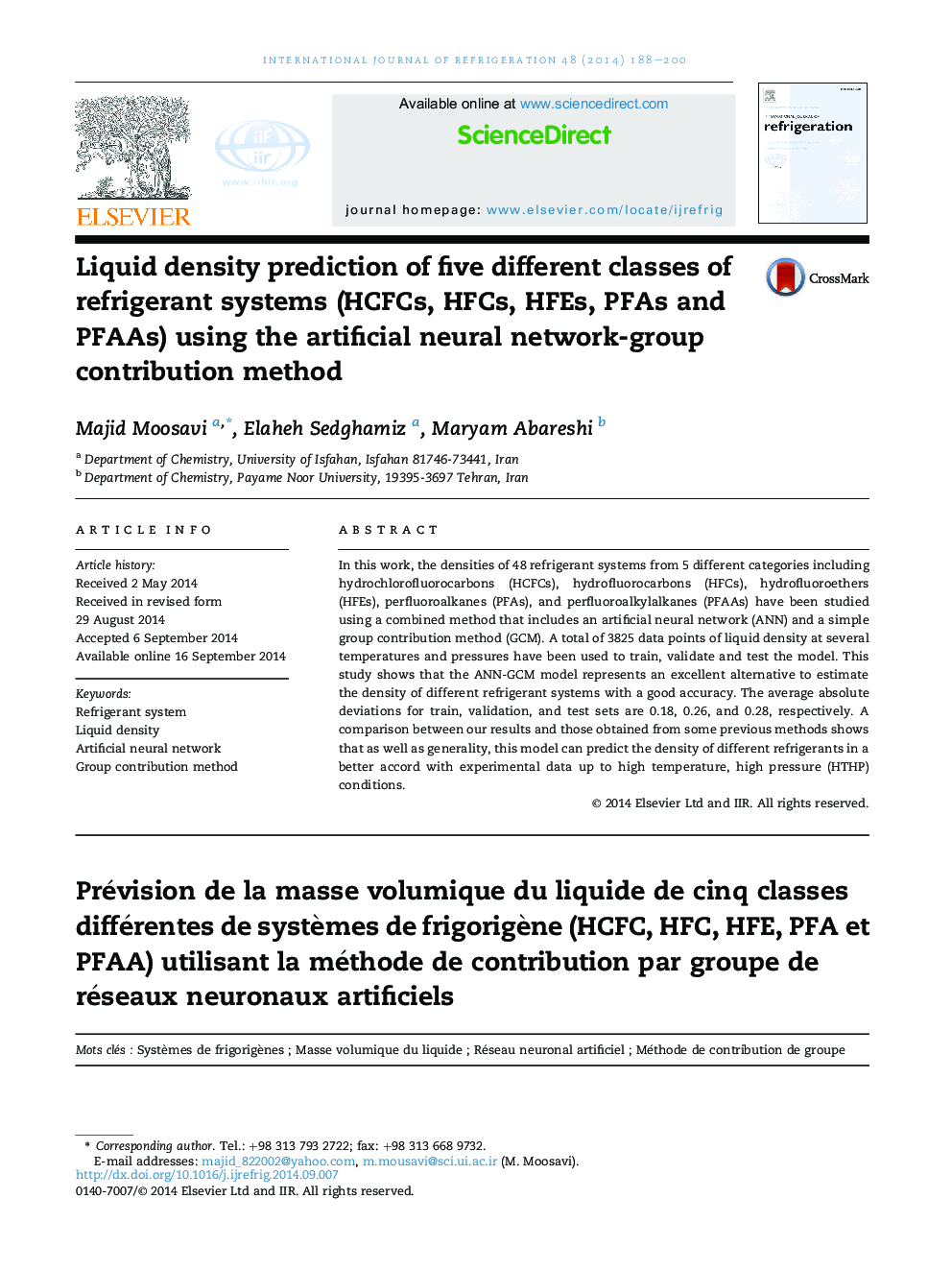
• Liquid density of 48 refrigerant systems has been estimated using ANN-GCM method.
• 5 categories of refrigerants (HCFCs, HFCs, HFEs, PFAs and PFAAs) were studied.
• The advantage of this technique is its high speed, simplicity and generalization.
• A comparison between this method and some previous works has been made.
• The AAD for train, validation, and test sets are 0.18, 0.26, and 0.28, respectively.
In this work, the densities of 48 refrigerant systems from 5 different categories including hydrochlorofluorocarbons (HCFCs), hydrofluorocarbons (HFCs), hydrofluoroethers (HFEs), perfluoroalkanes (PFAs), and perfluoroalkylalkanes (PFAAs) have been studied using a combined method that includes an artificial neural network (ANN) and a simple group contribution method (GCM). A total of 3825 data points of liquid density at several temperatures and pressures have been used to train, validate and test the model. This study shows that the ANN-GCM model represents an excellent alternative to estimate the density of different refrigerant systems with a good accuracy. The average absolute deviations for train, validation, and test sets are 0.18, 0.26, and 0.28, respectively. A comparison between our results and those obtained from some previous methods shows that as well as generality, this model can predict the density of different refrigerants in a better accord with experimental data up to high temperature, high pressure (HTHP) conditions.
Journal: International Journal of Refrigeration - Volume 48, December 2014, Pages 188–200