کد مقاله | کد نشریه | سال انتشار | مقاله انگلیسی | نسخه تمام متن |
---|---|---|---|---|
805202 | 1467864 | 2016 | 17 صفحه PDF | دانلود رایگان |
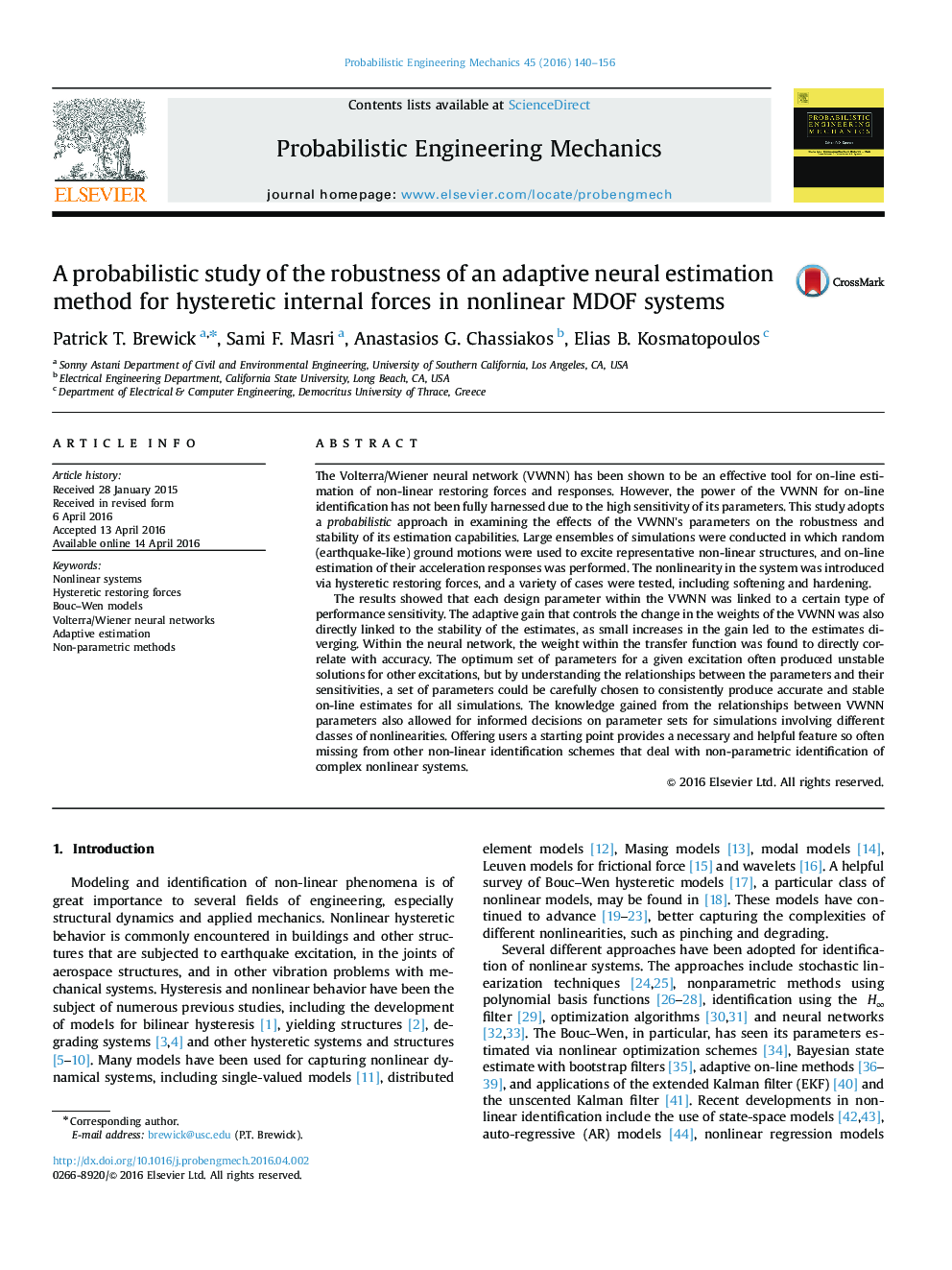
• Non-parametric, model-free identification of nonlinear hysteretic systems is performed using Volterra/Wiener neural networks.
• The Volterra/Wiener neural network identification results are examined from a probabilistic approach by conducting simulations in which Bouc-Wen hysteretic models, including both softening and hardening, are subjected to a series of random ground motions of similar intensity.
• The identification capabilities of the Volterra/Wiener neural network were tested for cases with and without adaptation, i.e. training “on” and training “off”.
• Performance of method based on RMS error between measured and predicted responses and the Volterra/Wiener neural network consistently provided accurate results with low RMS error across all simulation types.
• Guidance is provided to users of the proposed approach in regard to the optimum choice of control parameters for providing robust nonlinear computational models.
The Volterra/Wiener neural network (VWNN) has been shown to be an effective tool for on-line estimation of non-linear restoring forces and responses. However, the power of the VWNN for on-line identification has not been fully harnessed due to the high sensitivity of its parameters. This study adopts a probabilistic approach in examining the effects of the VWNN's parameters on the robustness and stability of its estimation capabilities. Large ensembles of simulations were conducted in which random (earthquake-like) ground motions were used to excite representative non-linear structures, and on-line estimation of their acceleration responses was performed. The nonlinearity in the system was introduced via hysteretic restoring forces, and a variety of cases were tested, including softening and hardening.The results showed that each design parameter within the VWNN was linked to a certain type of performance sensitivity. The adaptive gain that controls the change in the weights of the VWNN was also directly linked to the stability of the estimates, as small increases in the gain led to the estimates diverging. Within the neural network, the weight within the transfer function was found to directly correlate with accuracy. The optimum set of parameters for a given excitation often produced unstable solutions for other excitations, but by understanding the relationships between the parameters and their sensitivities, a set of parameters could be carefully chosen to consistently produce accurate and stable on-line estimates for all simulations. The knowledge gained from the relationships between VWNN parameters also allowed for informed decisions on parameter sets for simulations involving different classes of nonlinearities. Offering users a starting point provides a necessary and helpful feature so often missing from other non-linear identification schemes that deal with non-parametric identification of complex nonlinear systems.
Journal: Probabilistic Engineering Mechanics - Volume 45, July 2016, Pages 140–156