کد مقاله | کد نشریه | سال انتشار | مقاله انگلیسی | نسخه تمام متن |
---|---|---|---|---|
818937 | 906538 | 2013 | 9 صفحه PDF | دانلود رایگان |
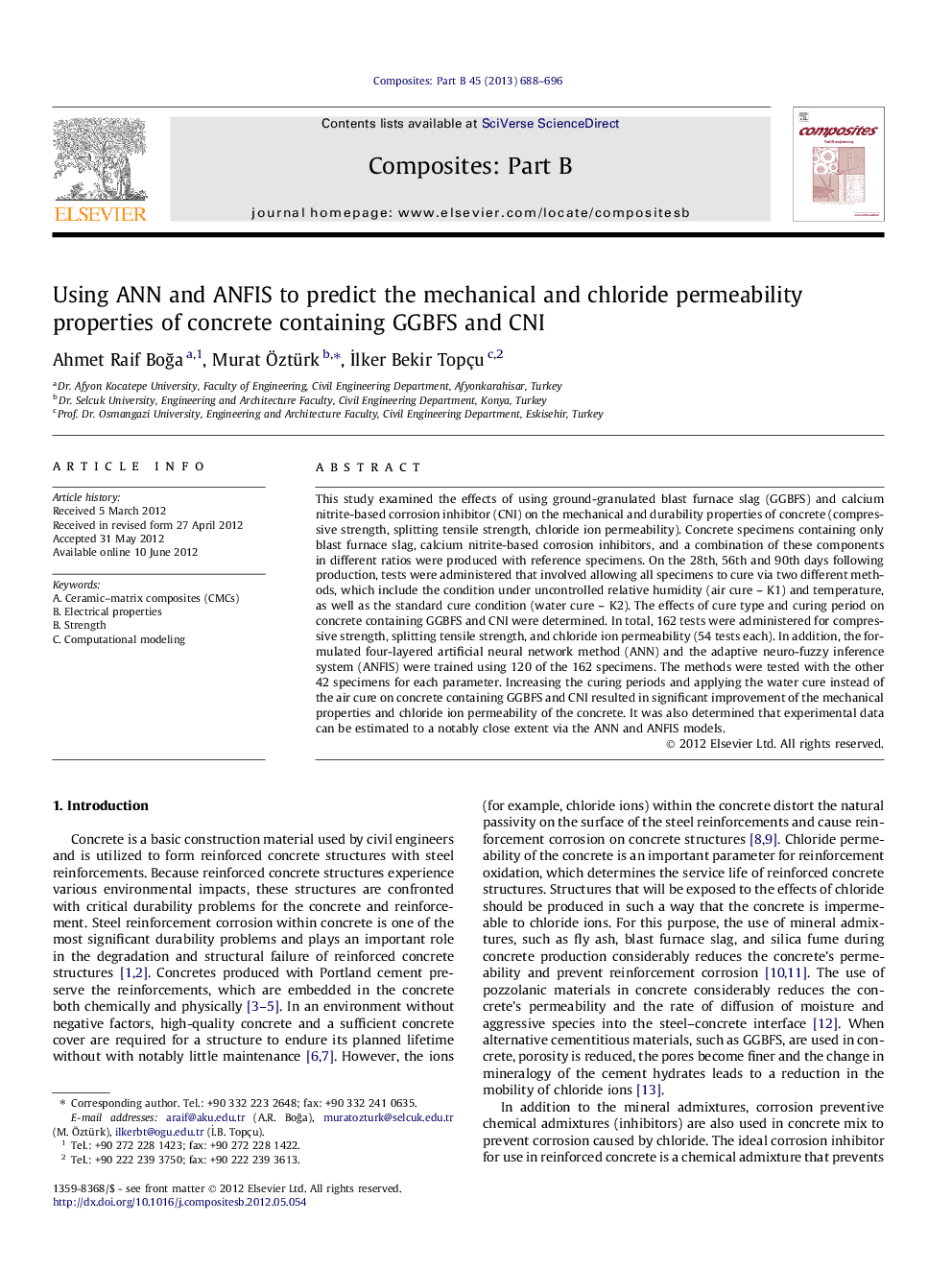
This study examined the effects of using ground-granulated blast furnace slag (GGBFS) and calcium nitrite-based corrosion inhibitor (CNI) on the mechanical and durability properties of concrete (compressive strength, splitting tensile strength, chloride ion permeability). Concrete specimens containing only blast furnace slag, calcium nitrite-based corrosion inhibitors, and a combination of these components in different ratios were produced with reference specimens. On the 28th, 56th and 90th days following production, tests were administered that involved allowing all specimens to cure via two different methods, which include the condition under uncontrolled relative humidity (air cure – K1) and temperature, as well as the standard cure condition (water cure – K2). The effects of cure type and curing period on concrete containing GGBFS and CNI were determined. In total, 162 tests were administered for compressive strength, splitting tensile strength, and chloride ion permeability (54 tests each). In addition, the formulated four-layered artificial neural network method (ANN) and the adaptive neuro-fuzzy inference system (ANFIS) were trained using 120 of the 162 specimens. The methods were tested with the other 42 specimens for each parameter. Increasing the curing periods and applying the water cure instead of the air cure on concrete containing GGBFS and CNI resulted in significant improvement of the mechanical properties and chloride ion permeability of the concrete. It was also determined that experimental data can be estimated to a notably close extent via the ANN and ANFIS models.
Journal: Composites Part B: Engineering - Volume 45, Issue 1, February 2013, Pages 688–696