کد مقاله | کد نشریه | سال انتشار | مقاله انگلیسی | نسخه تمام متن |
---|---|---|---|---|
9952421 | 1451674 | 2019 | 25 صفحه PDF | دانلود رایگان |
عنوان انگلیسی مقاله ISI
A Bi-LSTM memory network for end-to-end goal-oriented dialog learning
دانلود مقاله + سفارش ترجمه
دانلود مقاله ISI انگلیسی
رایگان برای ایرانیان
موضوعات مرتبط
مهندسی و علوم پایه
مهندسی کامپیوتر
پردازش سیگنال
پیش نمایش صفحه اول مقاله
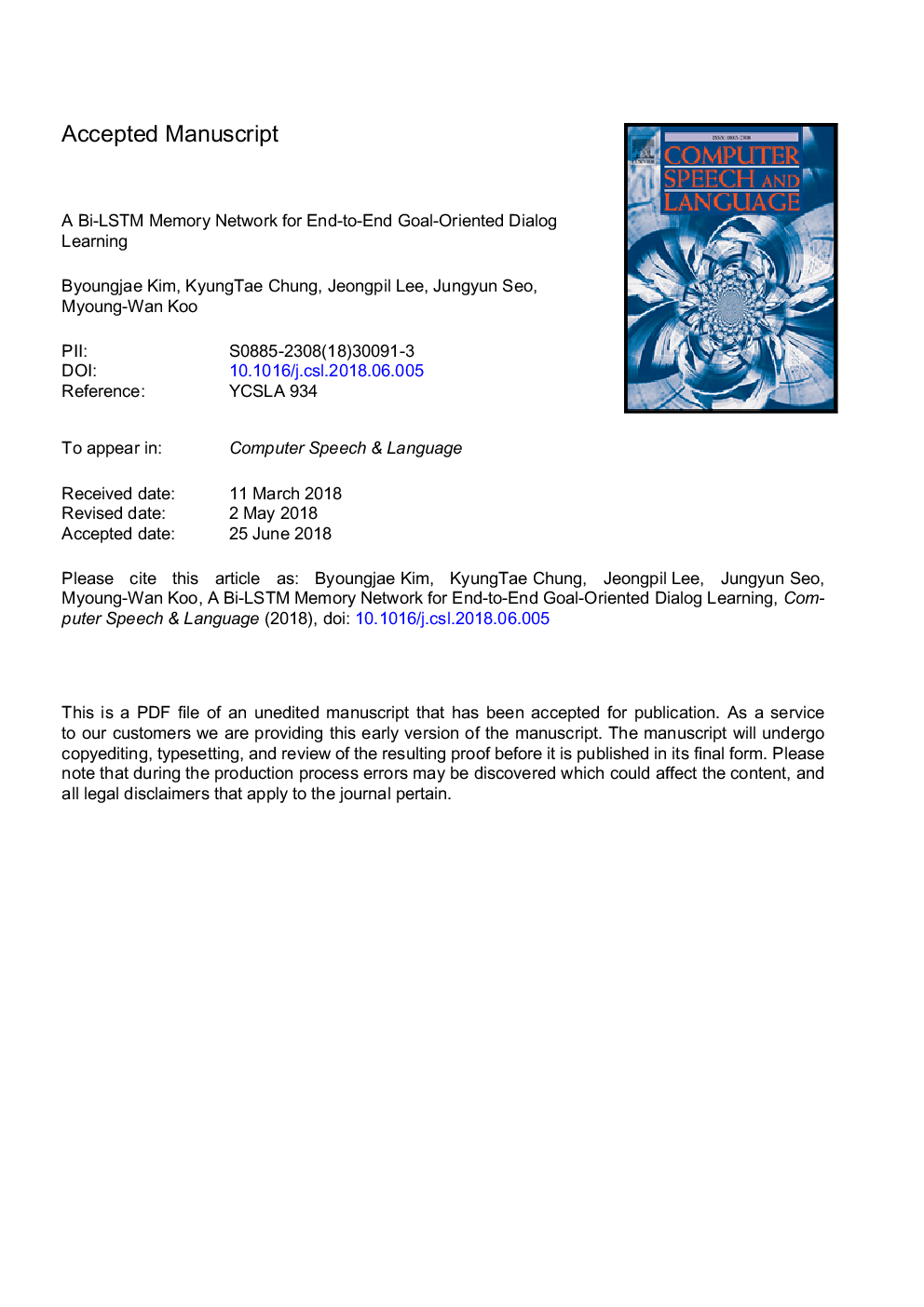
چکیده انگلیسی
We develop a model to satisfy the requirements of Dialog System Technology Challenge 6 (DSTC6) Track 1: building an end-to-end dialog systems for goal-oriented applications. This task involves learning a dialog policy from transactional dialogs in a given domain. Automatic system responses are generated using given task-oriented dialog data (http://workshop.colips.org/dstc6/index.html). As this task has a similar structure to a question answering task (Weston et al., 2015), we employ the MemN2N architecture (Sukhbaatar et al., 2015), which outperforms models based on recurrent neural networks or long short-term memory (LSTM). However, two problems arise when applying this model to the DSTC6 task. First, we encounter an out-of-vocabulary problem, which we resolve by categorizing the metadata types of words that exist in the knowledge base; the metadata is similar to the named entity. Second, the original memory network model has a weak ability to reflect sufficient temporal information, because it only uses sentence-level embeddings. Therefore, we add bidirectional LSTM (Bi-LSTM) at the beginning of the model to better reflect temporal information. The experimental results demonstrate that our model reflects temporal features well. Furthermore, our model achieves state-of-the-art performance among the memory networks, and is comparable to hybrid code networks (Ham et al., 2017) and hierarchical LSTM model (Bai et al., 2017) which is not an end-to-end architecture.
ناشر
Database: Elsevier - ScienceDirect (ساینس دایرکت)
Journal: Computer Speech & Language - Volume 53, January 2019, Pages 217-230
Journal: Computer Speech & Language - Volume 53, January 2019, Pages 217-230
نویسندگان
Byoungjae Kim, KyungTae Chung, Jeongpil Lee, Jungyun Seo, Myoung-Wan Koo,