کد مقاله | کد نشریه | سال انتشار | مقاله انگلیسی | نسخه تمام متن |
---|---|---|---|---|
10321800 | 660751 | 2015 | 50 صفحه PDF | دانلود رایگان |
عنوان انگلیسی مقاله ISI
Detection of masses in mammograms with adaption to breast density using genetic algorithm, phylogenetic trees, LBP and SVM
دانلود مقاله + سفارش ترجمه
دانلود مقاله ISI انگلیسی
رایگان برای ایرانیان
کلمات کلیدی
موضوعات مرتبط
مهندسی و علوم پایه
مهندسی کامپیوتر
هوش مصنوعی
پیش نمایش صفحه اول مقاله
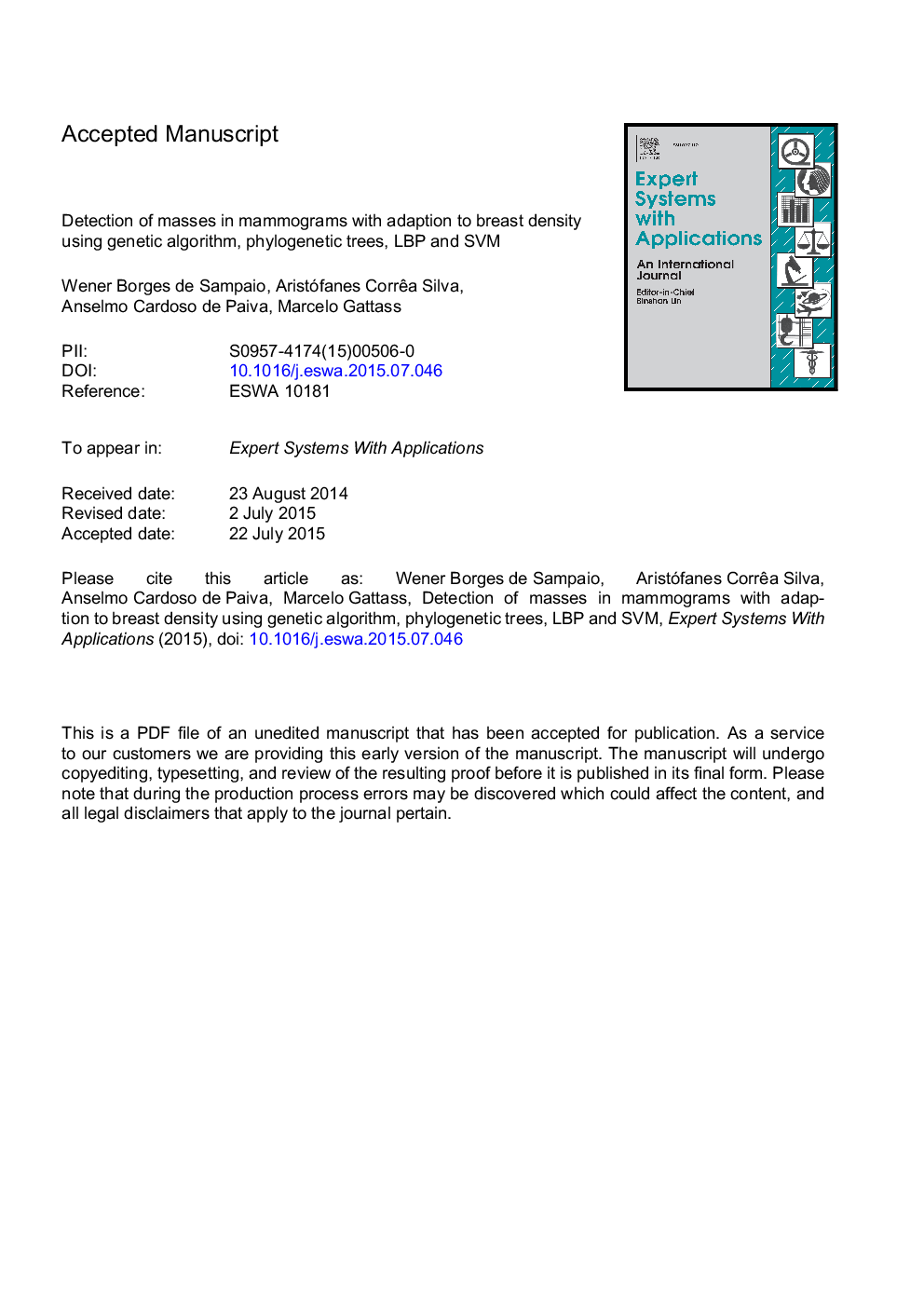
چکیده انگلیسی
Breast cancer is the second commonest type of cancer in the world, and the commonest among women, corresponding to 22% of the new cases every year. This work presents a new computational methodology, which helps the specialists in the detection of breast masses based on the breast density. The proposed methodology is divided into stages with the objective of overcoming several difficulties associated with the detection of masses. In many of these stages, we brought contributions to the areas. The first stage is intended to detect the type of density of the breast, which can be either dense or non-dense. We proposed an adaptive algorithm capable of analyzing and image and telling if it is dense or non-dense. The first stage consists in the segmentation of the regions that look like masses. We propose a novel use of the micro-genetic algorithm to create a texture proximity mask and select the regions suspect of containing lesions. The next stage is the reduction of false positives, which were generated in the previous stage. To this end, we proposed two new approaches. The first reduction of false positives used DBSCAN and a proximity ranking of the textures extracted from the ROIs. In the second reduction of false positives, the resulting regions have their textures analyzed by the combination of Phylogenetic Trees, Local Binary Patterns and Support Vector Machines (SVM). A micro-genetic algorithm was used to choose the suspect regions that generate the best training models and maximize the classification of masses and non-masses used in the SVM. The best result produced a sensitivity of 92.99%, a rate of 0.15 false positives per image and an area under the FROC curve of 0.96 in the analysis of the non-dense breasts; and a sensitivity of 83.70%, a rate of 0.19 false positives per image and an area under the FROC curve of 0.85, in the analysis of the dense breasts.
ناشر
Database: Elsevier - ScienceDirect (ساینس دایرکت)
Journal: Expert Systems with Applications - Volume 42, Issue 22, 1 December 2015, Pages 8911-8928
Journal: Expert Systems with Applications - Volume 42, Issue 22, 1 December 2015, Pages 8911-8928
نویسندگان
Wener Borges de Sampaio, Aristófanes Corrêa Silva, Anselmo Cardoso de Paiva, Marcelo Gattass,