کد مقاله | کد نشریه | سال انتشار | مقاله انگلیسی | نسخه تمام متن |
---|---|---|---|---|
10326074 | 677481 | 2005 | 18 صفحه PDF | دانلود رایگان |
عنوان انگلیسی مقاله ISI
Graph kernels for chemical informatics
دانلود مقاله + سفارش ترجمه
دانلود مقاله ISI انگلیسی
رایگان برای ایرانیان
کلمات کلیدی
موضوعات مرتبط
مهندسی و علوم پایه
مهندسی کامپیوتر
هوش مصنوعی
پیش نمایش صفحه اول مقاله
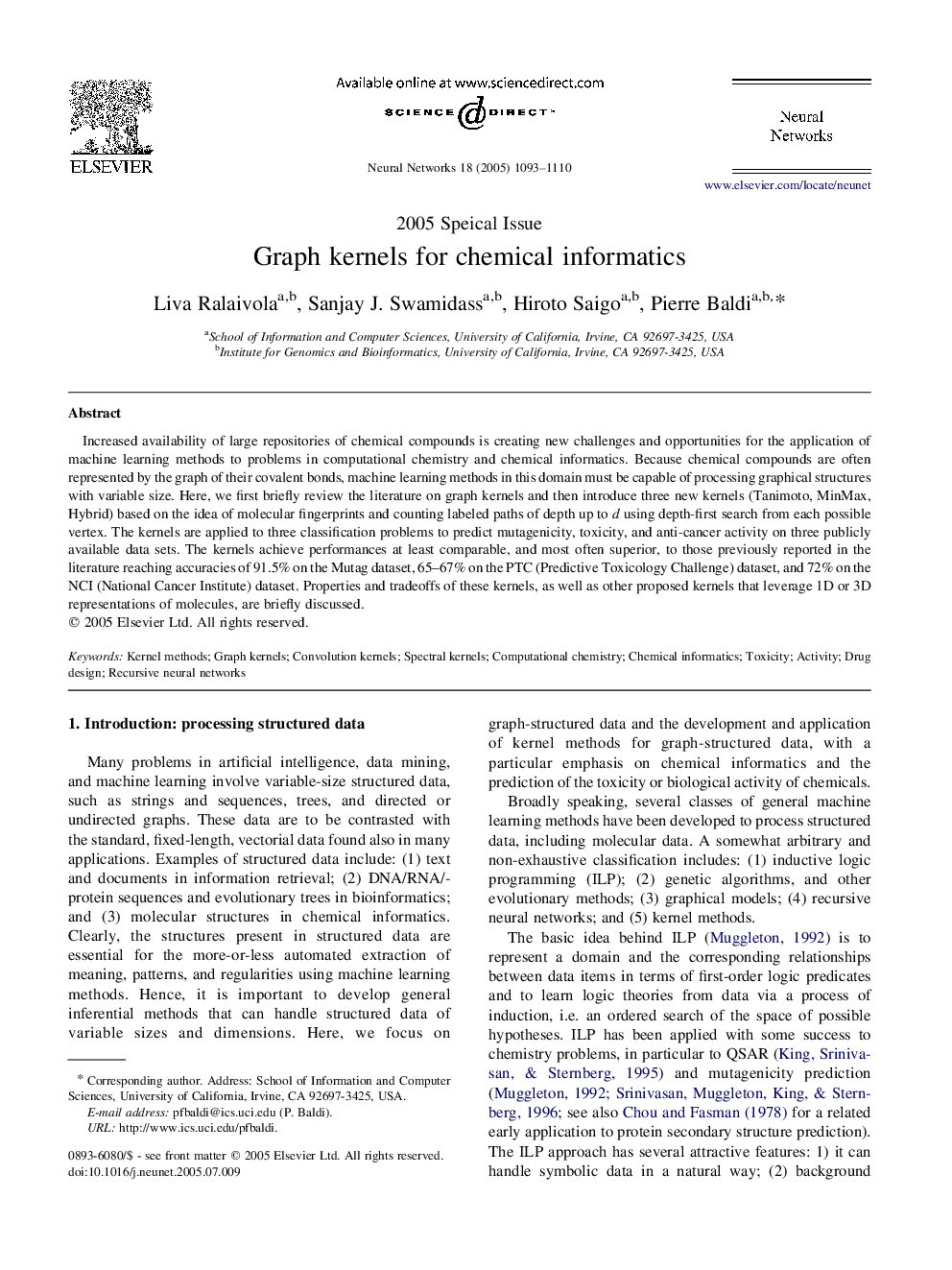
چکیده انگلیسی
Increased availability of large repositories of chemical compounds is creating new challenges and opportunities for the application of machine learning methods to problems in computational chemistry and chemical informatics. Because chemical compounds are often represented by the graph of their covalent bonds, machine learning methods in this domain must be capable of processing graphical structures with variable size. Here, we first briefly review the literature on graph kernels and then introduce three new kernels (Tanimoto, MinMax, Hybrid) based on the idea of molecular fingerprints and counting labeled paths of depth up to d using depth-first search from each possible vertex. The kernels are applied to three classification problems to predict mutagenicity, toxicity, and anti-cancer activity on three publicly available data sets. The kernels achieve performances at least comparable, and most often superior, to those previously reported in the literature reaching accuracies of 91.5% on the Mutag dataset, 65-67% on the PTC (Predictive Toxicology Challenge) dataset, and 72% on the NCI (National Cancer Institute) dataset. Properties and tradeoffs of these kernels, as well as other proposed kernels that leverage 1D or 3D representations of molecules, are briefly discussed.
ناشر
Database: Elsevier - ScienceDirect (ساینس دایرکت)
Journal: Neural Networks - Volume 18, Issue 8, October 2005, Pages 1093-1110
Journal: Neural Networks - Volume 18, Issue 8, October 2005, Pages 1093-1110
نویسندگان
Liva Ralaivola, Sanjay J. Swamidass, Hiroto Saigo, Pierre Baldi,