کد مقاله | کد نشریه | سال انتشار | مقاله انگلیسی | نسخه تمام متن |
---|---|---|---|---|
10345604 | 698352 | 2012 | 13 صفحه PDF | دانلود رایگان |
عنوان انگلیسی مقاله ISI
Wrapper feature selection for small sample size data driven by complete error estimates
دانلود مقاله + سفارش ترجمه
دانلود مقاله ISI انگلیسی
رایگان برای ایرانیان
کلمات کلیدی
موضوعات مرتبط
مهندسی و علوم پایه
مهندسی کامپیوتر
علوم کامپیوتر (عمومی)
پیش نمایش صفحه اول مقاله
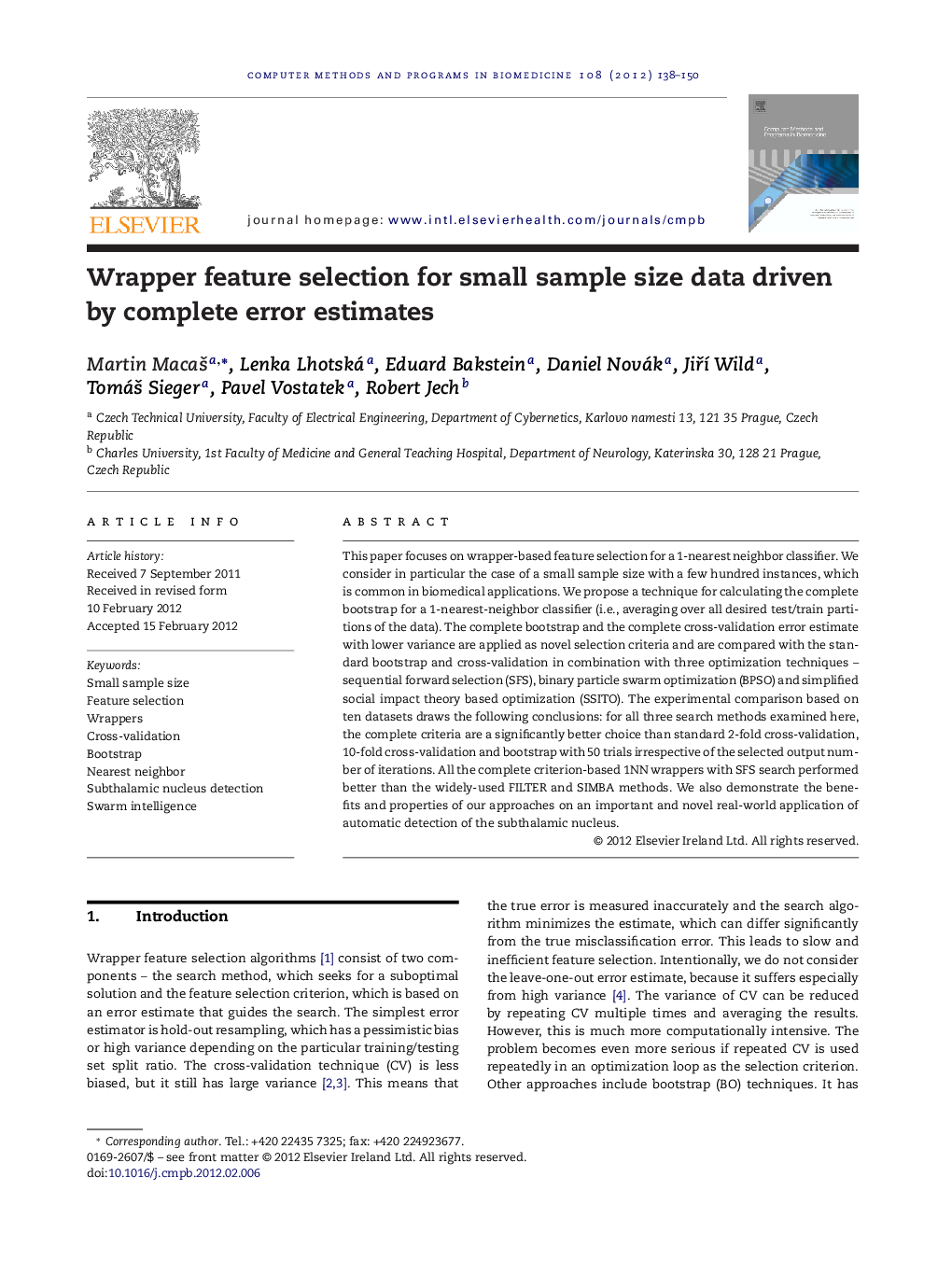
چکیده انگلیسی
This paper focuses on wrapper-based feature selection for a 1-nearest neighbor classifier. We consider in particular the case of a small sample size with a few hundred instances, which is common in biomedical applications. We propose a technique for calculating the complete bootstrap for a 1-nearest-neighbor classifier (i.e., averaging over all desired test/train partitions of the data). The complete bootstrap and the complete cross-validation error estimate with lower variance are applied as novel selection criteria and are compared with the standard bootstrap and cross-validation in combination with three optimization techniques - sequential forward selection (SFS), binary particle swarm optimization (BPSO) and simplified social impact theory based optimization (SSITO). The experimental comparison based on ten datasets draws the following conclusions: for all three search methods examined here, the complete criteria are a significantly better choice than standard 2-fold cross-validation, 10-fold cross-validation and bootstrap with 50 trials irrespective of the selected output number of iterations. All the complete criterion-based 1NN wrappers with SFS search performed better than the widely-used FILTER and SIMBA methods. We also demonstrate the benefits and properties of our approaches on an important and novel real-world application of automatic detection of the subthalamic nucleus.
ناشر
Database: Elsevier - ScienceDirect (ساینس دایرکت)
Journal: Computer Methods and Programs in Biomedicine - Volume 108, Issue 1, October 2012, Pages 138-150
Journal: Computer Methods and Programs in Biomedicine - Volume 108, Issue 1, October 2012, Pages 138-150
نویسندگان
Martin MacaÅ¡, Lenka Lhotská, Eduard Bakstein, Daniel Novák, JiÅà Wild, TomáÅ¡ Sieger, Pavel Vostatek, Robert Jech,