کد مقاله | کد نشریه | سال انتشار | مقاله انگلیسی | نسخه تمام متن |
---|---|---|---|---|
10347960 | 699386 | 2012 | 15 صفحه PDF | دانلود رایگان |
عنوان انگلیسی مقاله ISI
Measuring instance difficulty for combinatorial optimization problems
دانلود مقاله + سفارش ترجمه
دانلود مقاله ISI انگلیسی
رایگان برای ایرانیان
کلمات کلیدی
Algorithm selection - انتخاب الگوریتمPhase transition - انتقال فازBin-packing - بطری بسته بندیCombinatorial optimization - بهینهسازی ترکیبیاتیLandscape analysis - تجزیه و تحلیل چشم اندازGraph coloring - رنگ آمیزی گرافTimetabling - زمانبندیAssignment problem - مشکل اختصاص دادنKnapsack problem - مشکل حلقهTraveling salesman problem - مشکل فروشنده مسافرتیHardness prediction - پیش بینی سختی
موضوعات مرتبط
مهندسی و علوم پایه
مهندسی کامپیوتر
علوم کامپیوتر (عمومی)
پیش نمایش صفحه اول مقاله
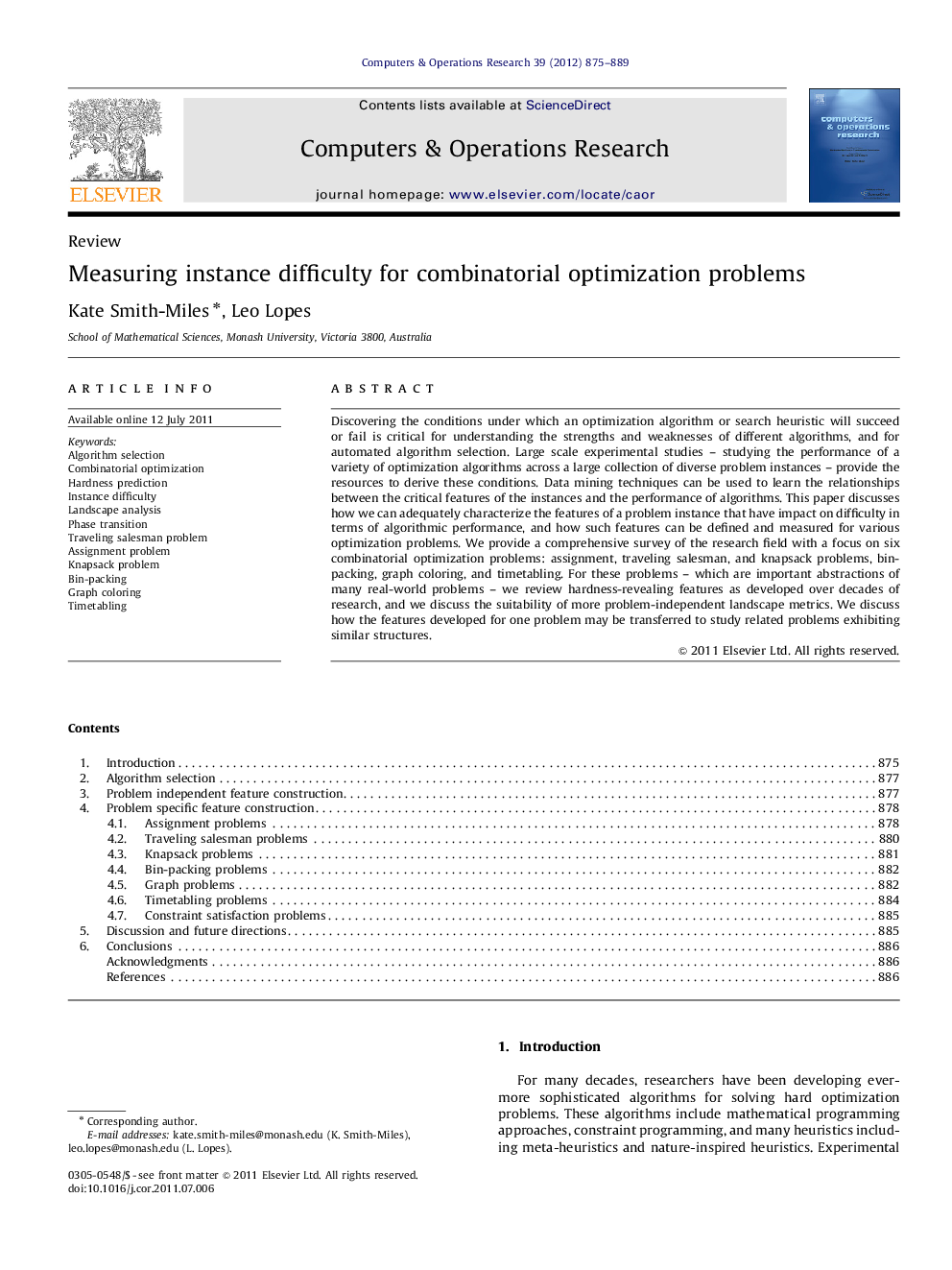
چکیده انگلیسی
Discovering the conditions under which an optimization algorithm or search heuristic will succeed or fail is critical for understanding the strengths and weaknesses of different algorithms, and for automated algorithm selection. Large scale experimental studies - studying the performance of a variety of optimization algorithms across a large collection of diverse problem instances - provide the resources to derive these conditions. Data mining techniques can be used to learn the relationships between the critical features of the instances and the performance of algorithms. This paper discusses how we can adequately characterize the features of a problem instance that have impact on difficulty in terms of algorithmic performance, and how such features can be defined and measured for various optimization problems. We provide a comprehensive survey of the research field with a focus on six combinatorial optimization problems: assignment, traveling salesman, and knapsack problems, bin-packing, graph coloring, and timetabling. For these problems - which are important abstractions of many real-world problems - we review hardness-revealing features as developed over decades of research, and we discuss the suitability of more problem-independent landscape metrics. We discuss how the features developed for one problem may be transferred to study related problems exhibiting similar structures.
ناشر
Database: Elsevier - ScienceDirect (ساینس دایرکت)
Journal: Computers & Operations Research - Volume 39, Issue 5, May 2012, Pages 875-889
Journal: Computers & Operations Research - Volume 39, Issue 5, May 2012, Pages 875-889
نویسندگان
Kate Smith-Miles, Leo Lopes,