کد مقاله | کد نشریه | سال انتشار | مقاله انگلیسی | نسخه تمام متن |
---|---|---|---|---|
10355823 | 867543 | 2005 | 13 صفحه PDF | دانلود رایگان |
عنوان انگلیسی مقاله ISI
GMDH-based feature ranking and selection for improved classification of medical data
دانلود مقاله + سفارش ترجمه
دانلود مقاله ISI انگلیسی
رایگان برای ایرانیان
کلمات کلیدی
Feature selection - انتخاب ویژگیHeart disease - بیماری قلبیMedical diagnosis - تشخیص پزشکیClassification accuracy - دقت طبقه بندیFeature ranking - رتبه بندی ویژگیBreast cancer - سرطان پستانAbductive networks - شبکه های رهایی دهندهNeural networks - شبکه های عصبیDimensionality reduction - کاهش ابعاد، فروکاهی ابعاد
موضوعات مرتبط
مهندسی و علوم پایه
مهندسی کامپیوتر
نرم افزارهای علوم کامپیوتر
پیش نمایش صفحه اول مقاله
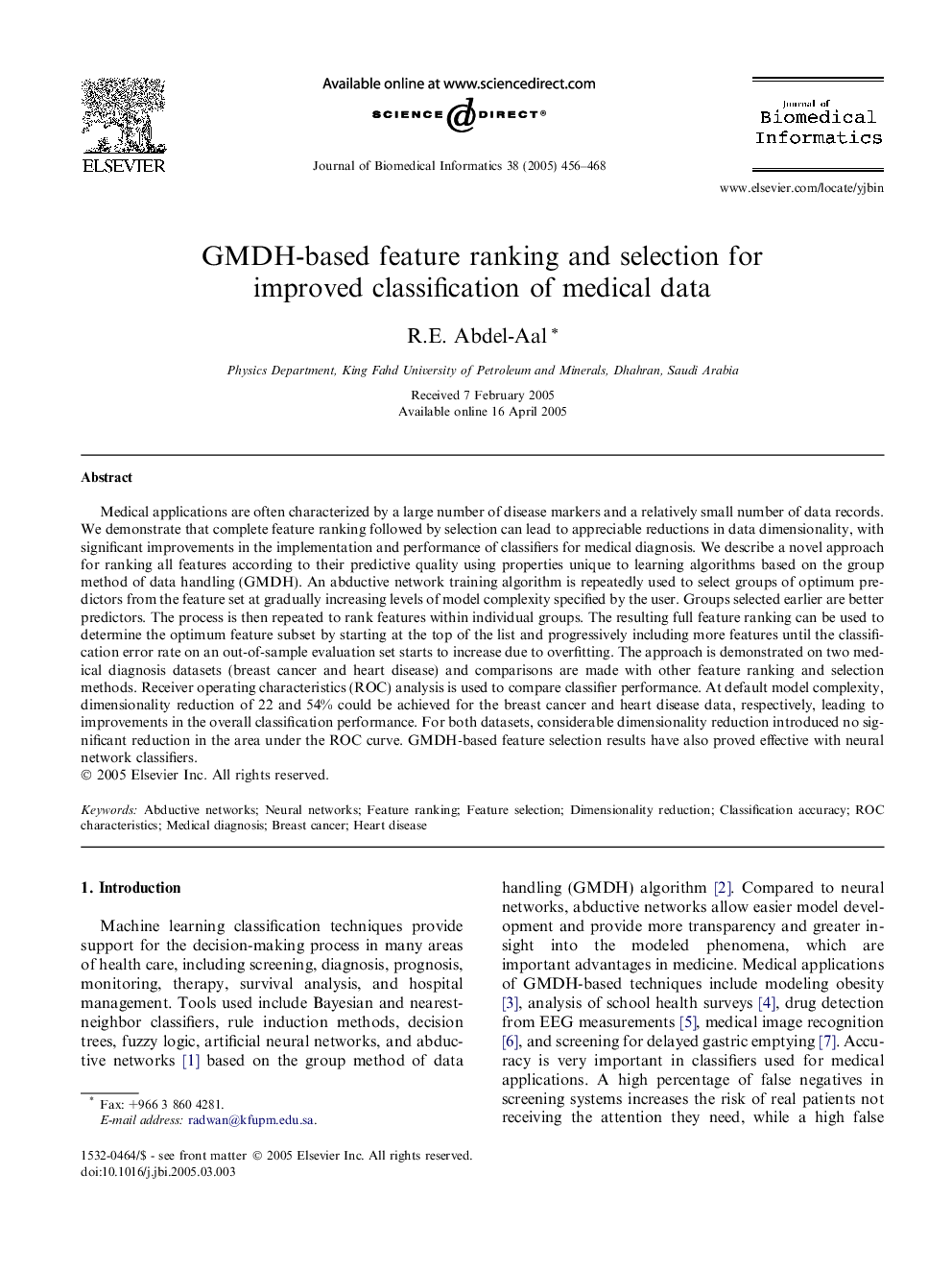
چکیده انگلیسی
Medical applications are often characterized by a large number of disease markers and a relatively small number of data records. We demonstrate that complete feature ranking followed by selection can lead to appreciable reductions in data dimensionality, with significant improvements in the implementation and performance of classifiers for medical diagnosis. We describe a novel approach for ranking all features according to their predictive quality using properties unique to learning algorithms based on the group method of data handling (GMDH). An abductive network training algorithm is repeatedly used to select groups of optimum predictors from the feature set at gradually increasing levels of model complexity specified by the user. Groups selected earlier are better predictors. The process is then repeated to rank features within individual groups. The resulting full feature ranking can be used to determine the optimum feature subset by starting at the top of the list and progressively including more features until the classification error rate on an out-of-sample evaluation set starts to increase due to overfitting. The approach is demonstrated on two medical diagnosis datasets (breast cancer and heart disease) and comparisons are made with other feature ranking and selection methods. Receiver operating characteristics (ROC) analysis is used to compare classifier performance. At default model complexity, dimensionality reduction of 22 and 54% could be achieved for the breast cancer and heart disease data, respectively, leading to improvements in the overall classification performance. For both datasets, considerable dimensionality reduction introduced no significant reduction in the area under the ROC curve. GMDH-based feature selection results have also proved effective with neural network classifiers.
ناشر
Database: Elsevier - ScienceDirect (ساینس دایرکت)
Journal: Journal of Biomedical Informatics - Volume 38, Issue 6, December 2005, Pages 456-468
Journal: Journal of Biomedical Informatics - Volume 38, Issue 6, December 2005, Pages 456-468
نویسندگان
R.E. Abdel-Aal,