کد مقاله | کد نشریه | سال انتشار | مقاله انگلیسی | نسخه تمام متن |
---|---|---|---|---|
11012491 | 1798846 | 2018 | 53 صفحه PDF | دانلود رایگان |
عنوان انگلیسی مقاله ISI
STSCR: Exploring spatial-temporal sequential influence and social information for location recommendation
دانلود مقاله + سفارش ترجمه
دانلود مقاله ISI انگلیسی
رایگان برای ایرانیان
موضوعات مرتبط
مهندسی و علوم پایه
مهندسی کامپیوتر
هوش مصنوعی
پیش نمایش صفحه اول مقاله
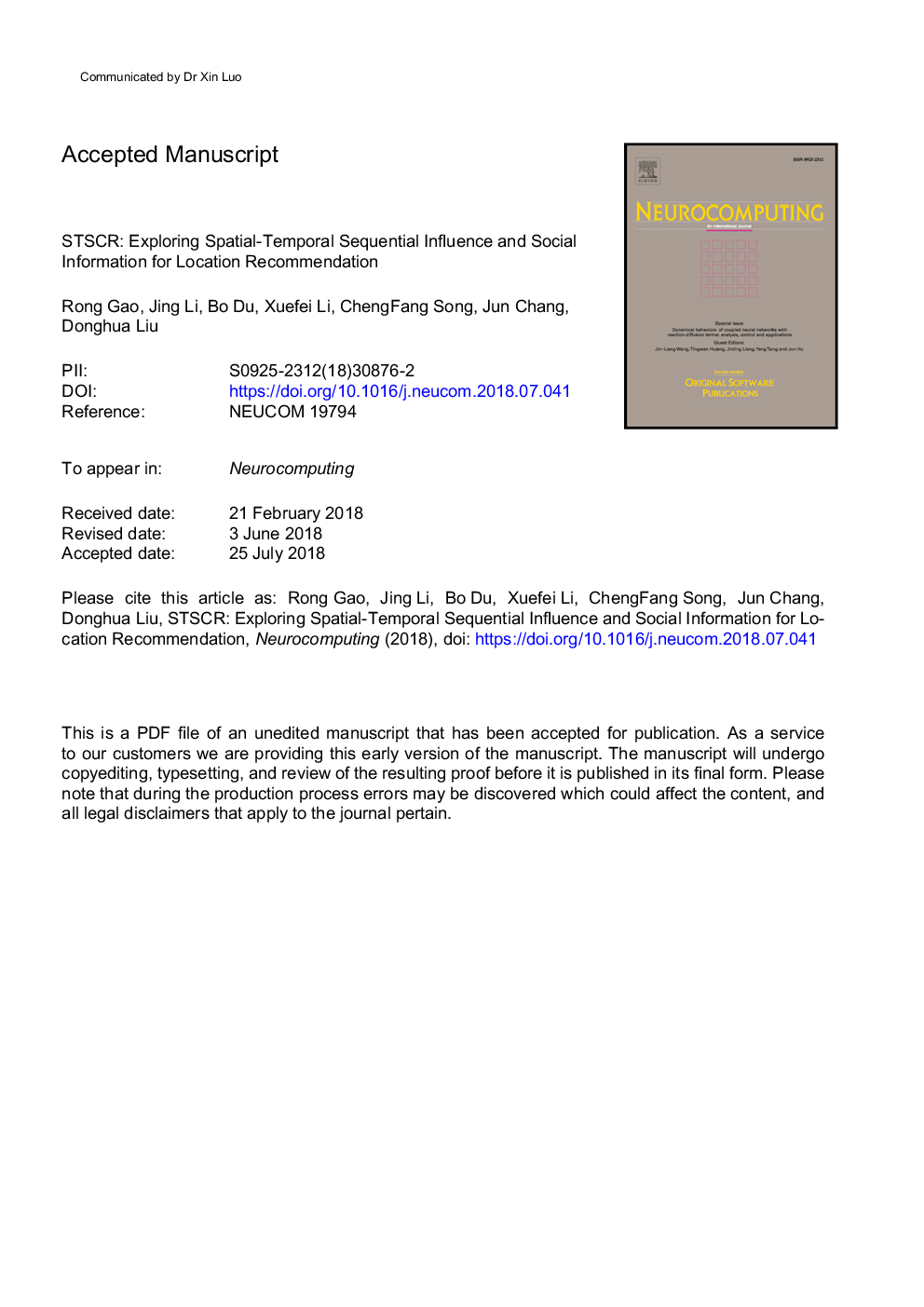
چکیده انگلیسی
One remarkable progress in spatial data analysis field lies on location-based social networks (LBSNs), where location recommendation has become an important way to help people locate the interesting places. When providing the location recommendations for users, spatial-temporal sequence of locations on users check-in behaviors have been intensively studied based on the fact that the human movement usually exhibits a pattern of spatial-temporal sequence. However, despite that some works have been proposed to focus on the spatial-temporal correlation among the sequences of users checked-in locations, they still suffer to the following their limitations: (1) these works are unable to handle time intervals between nearby check-in behaviors properly in modeling sequential data. In fact, POIs also have different probabilities of being checked in different time periods; (2) these works fail to employ the social relations adequately to improve final recommendation results especially when the check-in data is sparse; (3) these works are essentially based on the point-wise theory that aims to regress a real-valued score, whereas few work attempt to build a ranking-based estimator for recommendation. To solve the problems mentioned above, a new location recommendation model called Spatial-Temporal aware Social Collaborative Ranking(STSCR) model is proposed to explore the impact of time, spatial-temporal sequential influence and social influence. In particular, the proposed model is built upon a unified tensor factorization framework in which the ne-grained modeling of user-location, user-friend, friend-location, location-time, and location-location interactions. Then, the Bayesian personalized ranking technique is used to optimized the loss function of unified tensor factorization and fit the partial order of locations. As is shown in the experimental results derived from real datasets, our proposed STSCR achieves better recommendation performances than other state-of-the-art location recommendation algorithms.
ناشر
Database: Elsevier - ScienceDirect (ساینس دایرکت)
Journal: Neurocomputing - Volume 319, 30 November 2018, Pages 118-133
Journal: Neurocomputing - Volume 319, 30 November 2018, Pages 118-133
نویسندگان
Rong Gao, Jing Li, Xuefei Li, Chenfang Song, Jun Chang, Donghua Liu, Chunzhi Wang,