کد مقاله | کد نشریه | سال انتشار | مقاله انگلیسی | نسخه تمام متن |
---|---|---|---|---|
11020319 | 1717552 | 2019 | 16 صفحه PDF | دانلود رایگان |
عنوان انگلیسی مقاله ISI
Optimality of training/test size and resampling effectiveness in cross-validation
دانلود مقاله + سفارش ترجمه
دانلود مقاله ISI انگلیسی
رایگان برای ایرانیان
موضوعات مرتبط
مهندسی و علوم پایه
ریاضیات
ریاضیات کاربردی
پیش نمایش صفحه اول مقاله
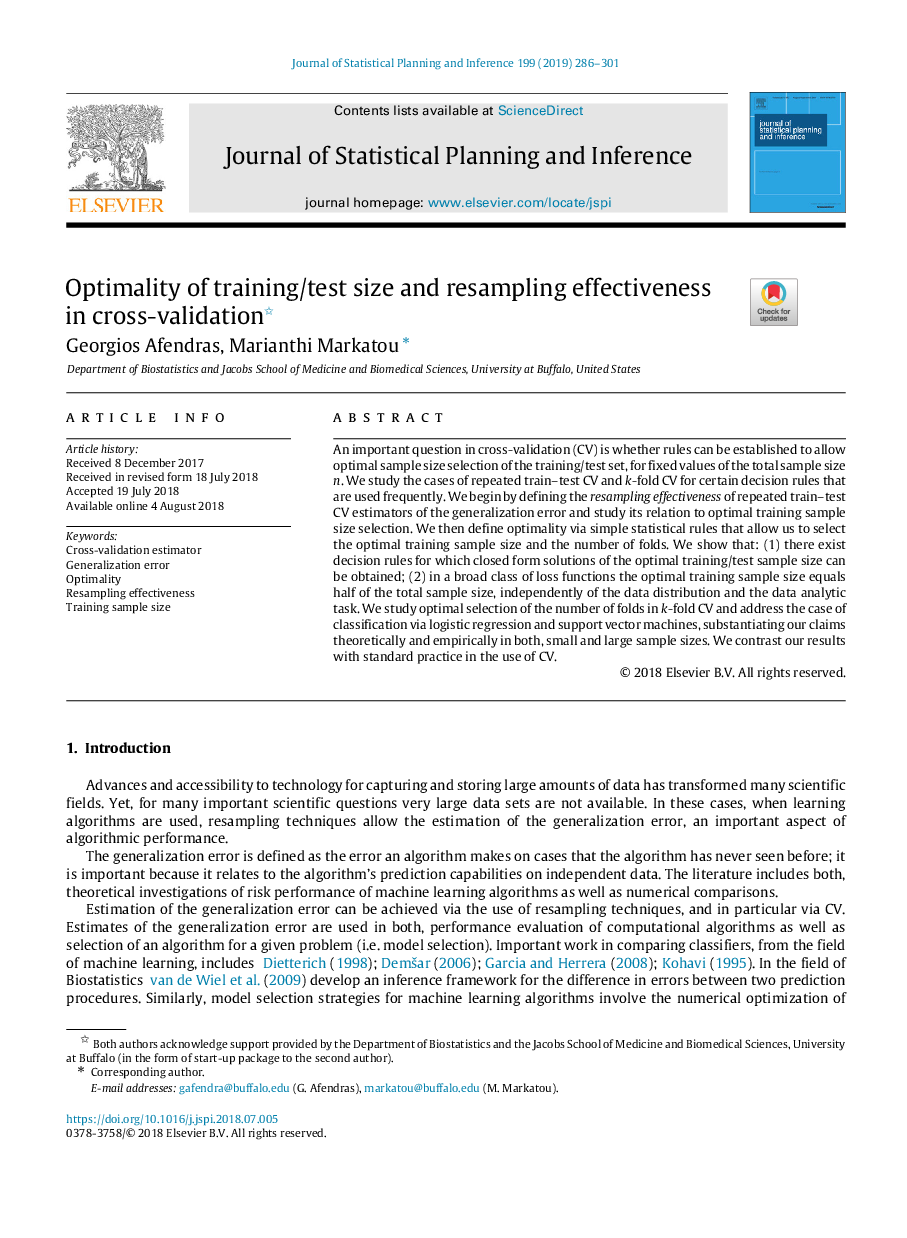
چکیده انگلیسی
An important question in cross-validation (CV) is whether rules can be established to allow optimal sample size selection of the training/test set, for fixed values of the total sample size n. We study the cases of repeated train-test CV and k-fold CV for certain decision rules that are used frequently. We begin by defining the resampling effectiveness of repeated train-test CV estimators of the generalization error and study its relation to optimal training sample size selection. We then define optimality via simple statistical rules that allow us to select the optimal training sample size and the number of folds. We show that: (1) there exist decision rules for which closed form solutions of the optimal training/test sample size can be obtained; (2) in a broad class of loss functions the optimal training sample size equals half of the total sample size, independently of the data distribution and the data analytic task. We study optimal selection of the number of folds in k-fold CV and address the case of classification via logistic regression and support vector machines, substantiating our claims theoretically and empirically in both, small and large sample sizes. We contrast our results with standard practice in the use of CV.
ناشر
Database: Elsevier - ScienceDirect (ساینس دایرکت)
Journal: Journal of Statistical Planning and Inference - Volume 199, March 2019, Pages 286-301
Journal: Journal of Statistical Planning and Inference - Volume 199, March 2019, Pages 286-301
نویسندگان
Georgios Afendras, Marianthi Markatou,