کد مقاله | کد نشریه | سال انتشار | مقاله انگلیسی | نسخه تمام متن |
---|---|---|---|---|
11021192 | 1715031 | 2019 | 27 صفحه PDF | دانلود رایگان |
عنوان انگلیسی مقاله ISI
Multiobjective Hooke-Jeeves algorithm with a stochastic Newton-Raphson-like step-size method
دانلود مقاله + سفارش ترجمه
دانلود مقاله ISI انگلیسی
رایگان برای ایرانیان
کلمات کلیدی
موضوعات مرتبط
مهندسی و علوم پایه
مهندسی کامپیوتر
هوش مصنوعی
پیش نمایش صفحه اول مقاله
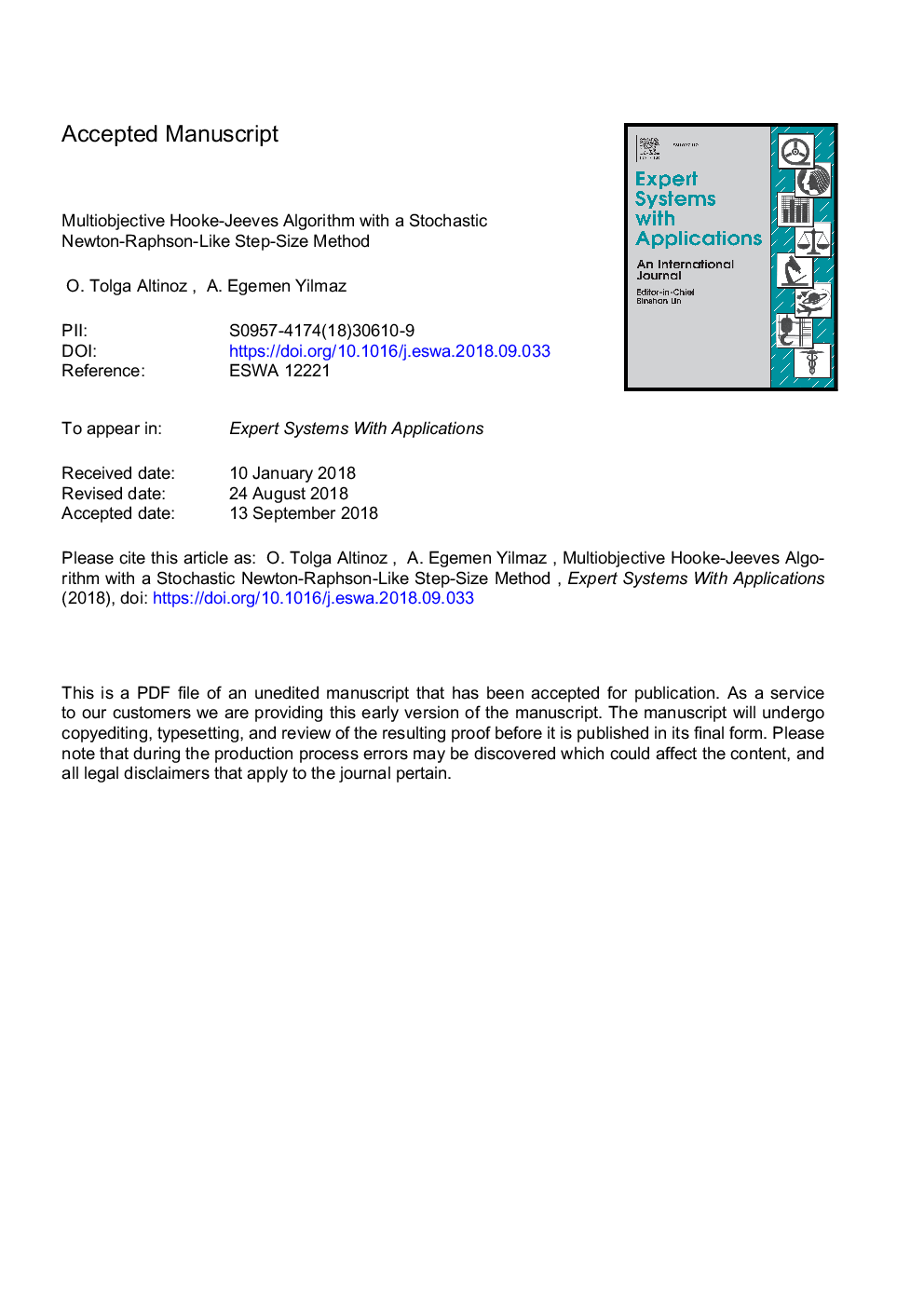
چکیده انگلیسی
Computational optimization algorithms are focused on the improvement of meta-heuristic algorithms in a way that they can able to handle problems with more than one objective; such improved algorithms are called multiobjective optimization algorithms. As the number of objectives is increased, the complexity of the algorithm is increased with respect to the computational cost. Because classical optimization algorithms follow the direction of descending values by calculating derivations of the function, it is possible to evaluate a classical optimization algorithm as the core of a novel multiobjective optimization algorithm. Among the classical optimization algorithms, in this study, the Hooke-Jeeves (HJ) algorithm is selected as the basis of the proposed multiobjective optimization algorithm, in which members of the proposed population-based HJ algorithm move to the Pareto front by checking two neighborhood solutions at each dimension, with a dynamic distance that is calculated by using the Newton-Raphson-like stochastic step-size method. Unlike various multiobjective optimization algorithms, the performance of the proposed algorithm is greatly dependent on the decision space dimension instead of the number of objectives. As the number of objectives increases without changing the decision dimension, the computational cost almost remains the same. In addition, the proposed algorithm can be applied to single, multiple and many objective optimization problems. In this study, initially, the behaviors of the HJ and proposed multiobjective HJ algorithms are evaluated by theoretical and graphical demonstrations. Next, the performance of the proposed method is evaluated on well-known benchmark problems, and the performance of this algorithm is compared with the Nondominated Sorting Genetic Algorithm-II (NSGA-II) algorithm by using three different metric calculations. Finally, the algorithm is applied to many-objective optimization problems, and the performance of the proposed algorithm is evaluated based on the obtained results.
ناشر
Database: Elsevier - ScienceDirect (ساینس دایرکت)
Journal: Expert Systems with Applications - Volume 117, 1 March 2019, Pages 166-175
Journal: Expert Systems with Applications - Volume 117, 1 March 2019, Pages 166-175
نویسندگان
O. Tolga Altinoz, A. Egemen Yilmaz,