کد مقاله | کد نشریه | سال انتشار | مقاله انگلیسی | نسخه تمام متن |
---|---|---|---|---|
1135115 | 956089 | 2012 | 16 صفحه PDF | دانلود رایگان |
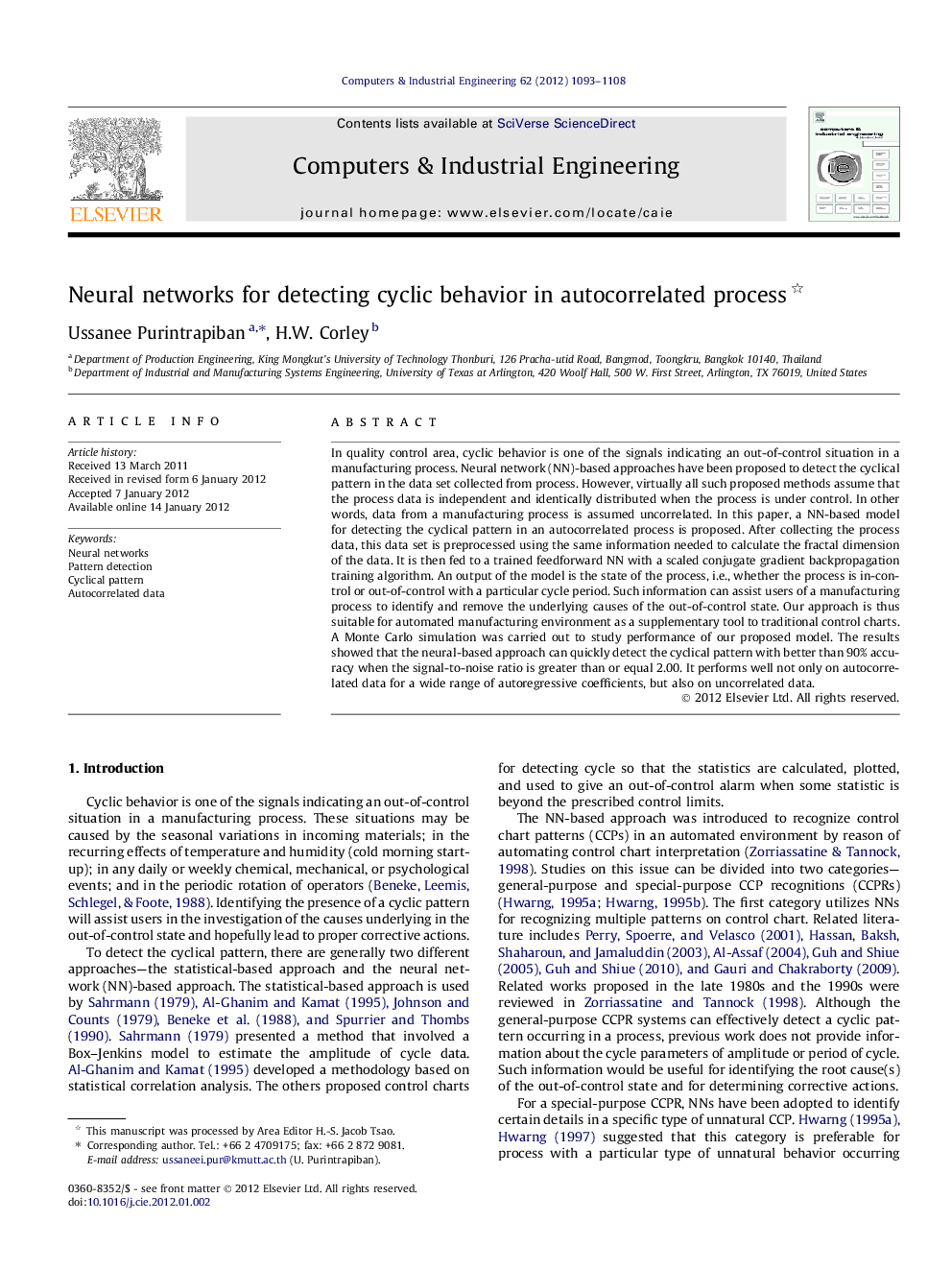
In quality control area, cyclic behavior is one of the signals indicating an out-of-control situation in a manufacturing process. Neural network (NN)-based approaches have been proposed to detect the cyclical pattern in the data set collected from process. However, virtually all such proposed methods assume that the process data is independent and identically distributed when the process is under control. In other words, data from a manufacturing process is assumed uncorrelated. In this paper, a NN-based model for detecting the cyclical pattern in an autocorrelated process is proposed. After collecting the process data, this data set is preprocessed using the same information needed to calculate the fractal dimension of the data. It is then fed to a trained feedforward NN with a scaled conjugate gradient backpropagation training algorithm. An output of the model is the state of the process, i.e., whether the process is in-control or out-of-control with a particular cycle period. Such information can assist users of a manufacturing process to identify and remove the underlying causes of the out-of-control state. Our approach is thus suitable for automated manufacturing environment as a supplementary tool to traditional control charts. A Monte Carlo simulation was carried out to study performance of our proposed model. The results showed that the neural-based approach can quickly detect the cyclical pattern with better than 90% accuracy when the signal-to-noise ratio is greater than or equal 2.00. It performs well not only on autocorrelated data for a wide range of autoregressive coefficients, but also on uncorrelated data.
► A NN-based CCP recognizer for a cyclic pattern in autocorrelated process is proposed.
► The fractal dimension calculation is applied as a preprocessing technique.
► The fractal dimension calculation is effective to deal with dynamic patterns.
► This special-purpose CCPR can provide the period value when a cyclical pattern existed in the data.
Journal: Computers & Industrial Engineering - Volume 62, Issue 4, May 2012, Pages 1093–1108