کد مقاله | کد نشریه | سال انتشار | مقاله انگلیسی | نسخه تمام متن |
---|---|---|---|---|
1137337 | 1489169 | 2010 | 5 صفحه PDF | دانلود رایگان |
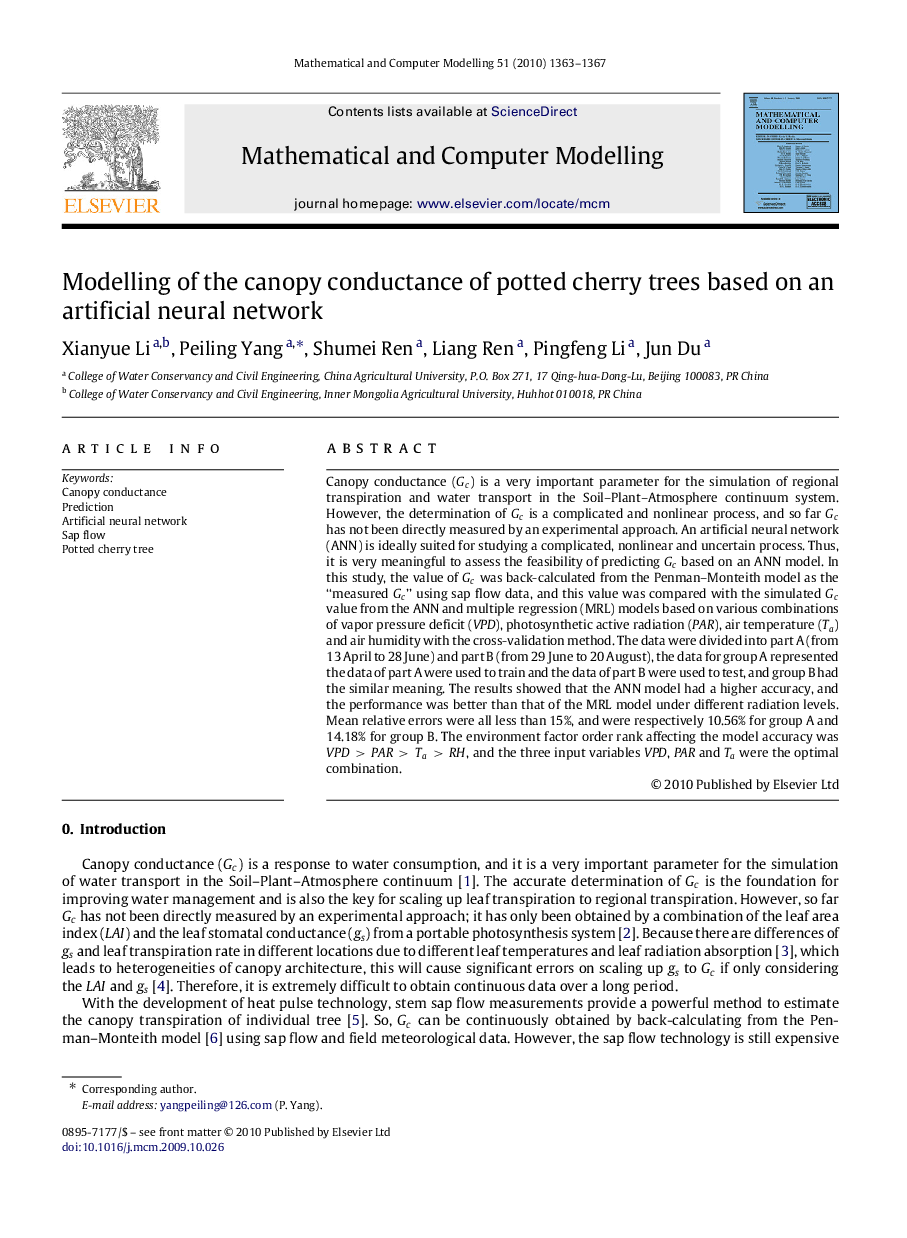
Canopy conductance (GcGc) is a very important parameter for the simulation of regional transpiration and water transport in the Soil–Plant–Atmosphere continuum system. However, the determination of GcGc is a complicated and nonlinear process, and so far GcGc has not been directly measured by an experimental approach. An artificial neural network (ANN) is ideally suited for studying a complicated, nonlinear and uncertain process. Thus, it is very meaningful to assess the feasibility of predicting GcGc based on an ANN model. In this study, the value of GcGc was back-calculated from the Penman–Monteith model as the “measured GcGc” using sap flow data, and this value was compared with the simulated GcGc value from the ANN and multiple regression (MRL) models based on various combinations of vapor pressure deficit (V PD), photosynthetic active radiation (PAR), air temperature (TaTa) and air humidity with the cross-validation method. The data were divided into part A (from 13 April to 28 June) and part B (from 29 June to 20 August), the data for group A represented the data of part A were used to train and the data of part B were used to test, and group B had the similar meaning. The results showed that the ANN model had a higher accuracy, and the performance was better than that of the MRL model under different radiation levels. Mean relative errors were all less than 15%, and were respectively 10.56% for group A and 14.18% for group B. The environment factor order rank affecting the model accuracy was V PD>PAR>Ta>RH, and the three input variables V PD, PAR and TaTa were the optimal combination.
Journal: Mathematical and Computer Modelling - Volume 51, Issues 11–12, June 2010, Pages 1363–1367