کد مقاله | کد نشریه | سال انتشار | مقاله انگلیسی | نسخه تمام متن |
---|---|---|---|---|
1146244 | 957501 | 2012 | 17 صفحه PDF | دانلود رایگان |
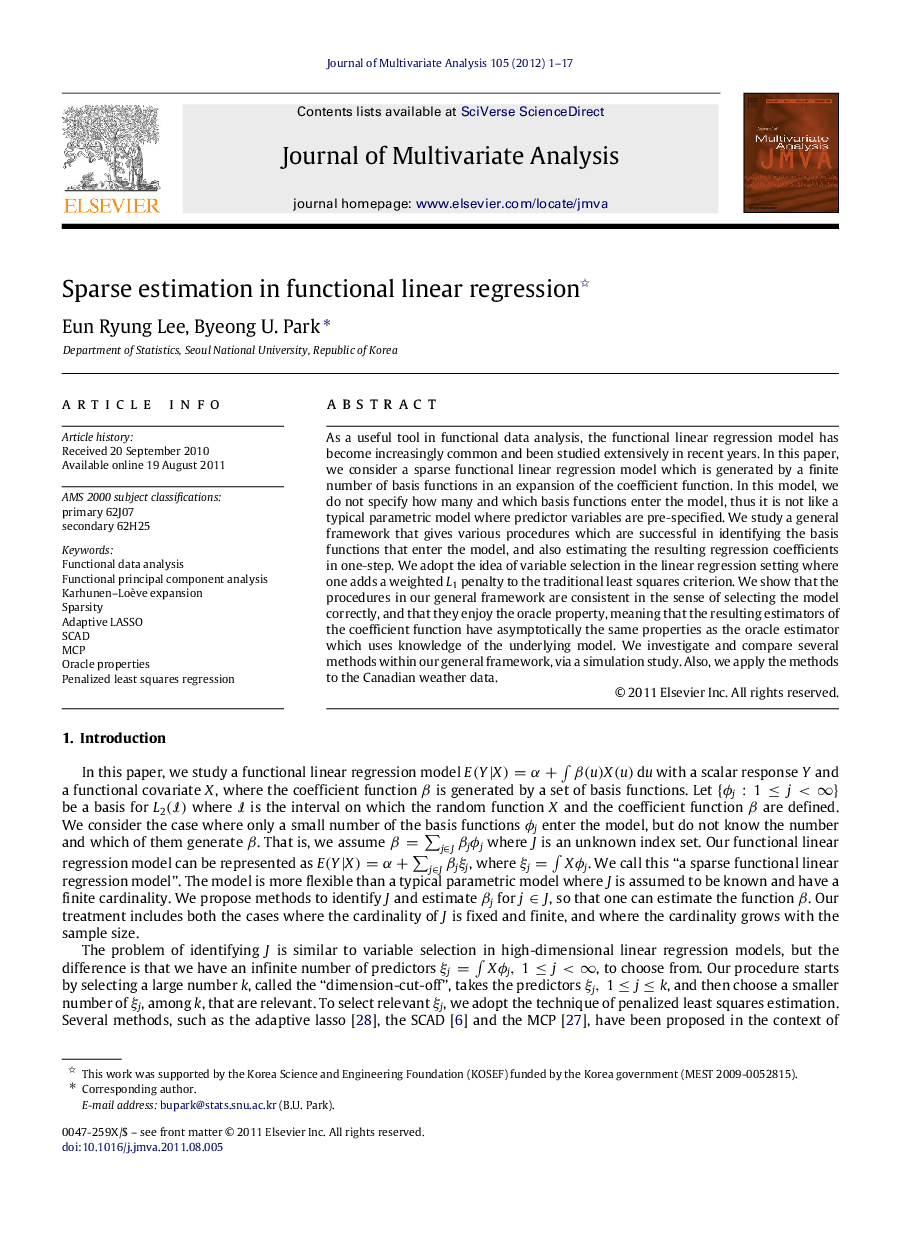
As a useful tool in functional data analysis, the functional linear regression model has become increasingly common and been studied extensively in recent years. In this paper, we consider a sparse functional linear regression model which is generated by a finite number of basis functions in an expansion of the coefficient function. In this model, we do not specify how many and which basis functions enter the model, thus it is not like a typical parametric model where predictor variables are pre-specified. We study a general framework that gives various procedures which are successful in identifying the basis functions that enter the model, and also estimating the resulting regression coefficients in one-step. We adopt the idea of variable selection in the linear regression setting where one adds a weighted L1L1 penalty to the traditional least squares criterion. We show that the procedures in our general framework are consistent in the sense of selecting the model correctly, and that they enjoy the oracle property, meaning that the resulting estimators of the coefficient function have asymptotically the same properties as the oracle estimator which uses knowledge of the underlying model. We investigate and compare several methods within our general framework, via a simulation study. Also, we apply the methods to the Canadian weather data.
Journal: Journal of Multivariate Analysis - Volume 105, Issue 1, February 2012, Pages 1–17