کد مقاله | کد نشریه | سال انتشار | مقاله انگلیسی | نسخه تمام متن |
---|---|---|---|---|
1148580 | 957841 | 2007 | 17 صفحه PDF | دانلود رایگان |
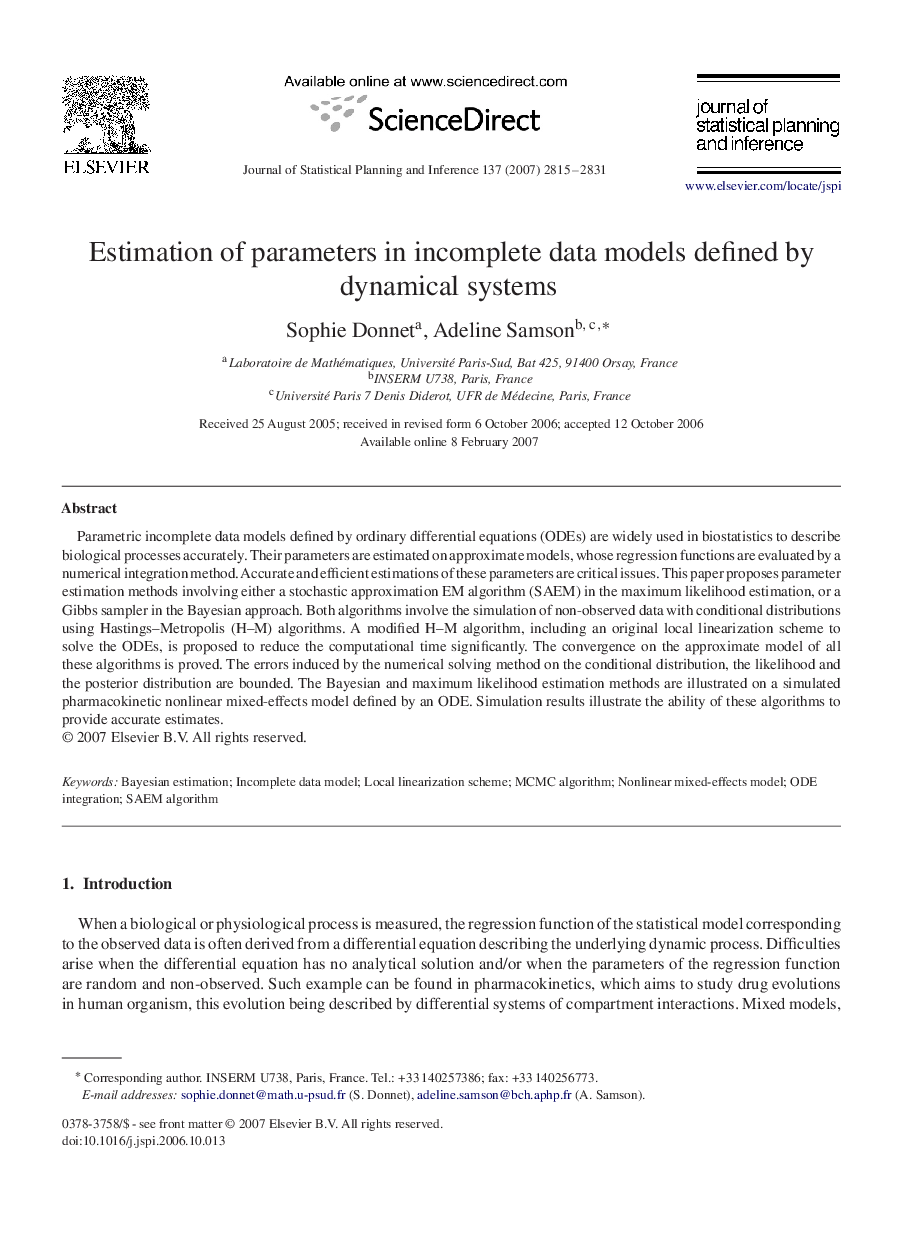
Parametric incomplete data models defined by ordinary differential equations (ODEs) are widely used in biostatistics to describe biological processes accurately. Their parameters are estimated on approximate models, whose regression functions are evaluated by a numerical integration method. Accurate and efficient estimations of these parameters are critical issues. This paper proposes parameter estimation methods involving either a stochastic approximation EM algorithm (SAEM) in the maximum likelihood estimation, or a Gibbs sampler in the Bayesian approach. Both algorithms involve the simulation of non-observed data with conditional distributions using Hastings–Metropolis (H–M) algorithms. A modified H–M algorithm, including an original local linearization scheme to solve the ODEs, is proposed to reduce the computational time significantly. The convergence on the approximate model of all these algorithms is proved. The errors induced by the numerical solving method on the conditional distribution, the likelihood and the posterior distribution are bounded. The Bayesian and maximum likelihood estimation methods are illustrated on a simulated pharmacokinetic nonlinear mixed-effects model defined by an ODE. Simulation results illustrate the ability of these algorithms to provide accurate estimates.
Journal: Journal of Statistical Planning and Inference - Volume 137, Issue 9, 1 September 2007, Pages 2815–2831