کد مقاله | کد نشریه | سال انتشار | مقاله انگلیسی | نسخه تمام متن |
---|---|---|---|---|
13428539 | 1842259 | 2020 | 12 صفحه PDF | دانلود رایگان |
عنوان انگلیسی مقاله ISI
Application of multi-feature fusion and random forests to the automated detection of myocardial infarction
دانلود مقاله + سفارش ترجمه
دانلود مقاله ISI انگلیسی
رایگان برای ایرانیان
کلمات کلیدی
موضوعات مرتبط
مهندسی و علوم پایه
مهندسی کامپیوتر
هوش مصنوعی
پیش نمایش صفحه اول مقاله
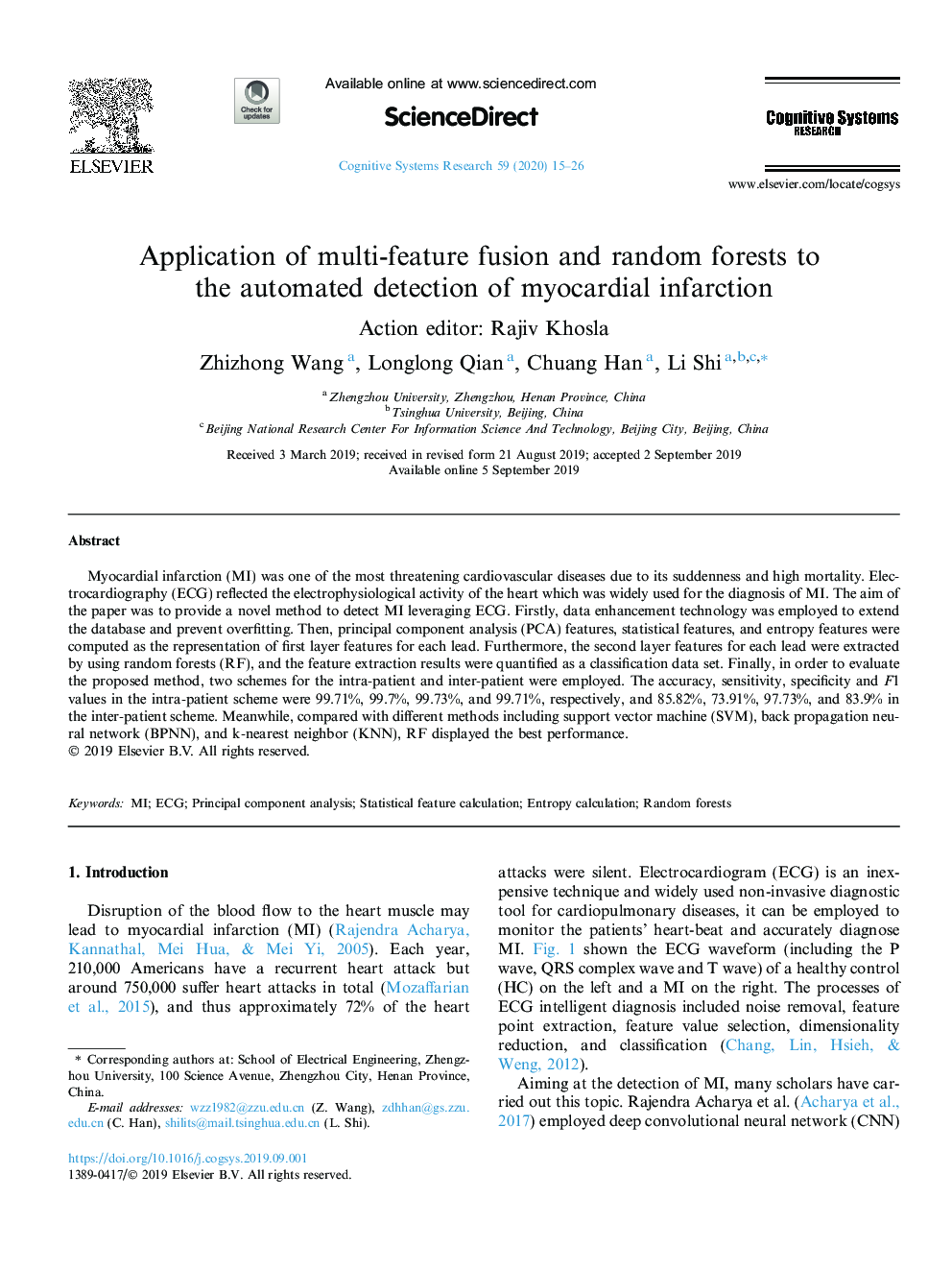
چکیده انگلیسی
Myocardial infarction (MI) was one of the most threatening cardiovascular diseases due to its suddenness and high mortality. Electrocardiography (ECG) reflected the electrophysiological activity of the heart which was widely used for the diagnosis of MI. The aim of the paper was to provide a novel method to detect MI leveraging ECG. Firstly, data enhancement technology was employed to extend the database and prevent overfitting. Then, principal component analysis (PCA) features, statistical features, and entropy features were computed as the representation of first layer features for each lead. Furthermore, the second layer features for each lead were extracted by using random forests (RF), and the feature extraction results were quantified as a classification data set. Finally, in order to evaluate the proposed method, two schemes for the intra-patient and inter-patient were employed. The accuracy, sensitivity, specificity and F1 values in the intra-patient scheme were 99.71%, 99.7%, 99.73%, and 99.71%, respectively, and 85.82%, 73.91%, 97.73%, and 83.9% in the inter-patient scheme. Meanwhile, compared with different methods including support vector machine (SVM), back propagation neural network (BPNN), and k-nearest neighbor (KNN), RF displayed the best performance.
ناشر
Database: Elsevier - ScienceDirect (ساینس دایرکت)
Journal: Cognitive Systems Research - Volume 59, January 2020, Pages 15-26
Journal: Cognitive Systems Research - Volume 59, January 2020, Pages 15-26
نویسندگان
Zhizhong Wang, Longlong Qian, Chuang Han, Li Shi,