کد مقاله | کد نشریه | سال انتشار | مقاله انگلیسی | نسخه تمام متن |
---|---|---|---|---|
155566 | 456902 | 2012 | 9 صفحه PDF | دانلود رایگان |
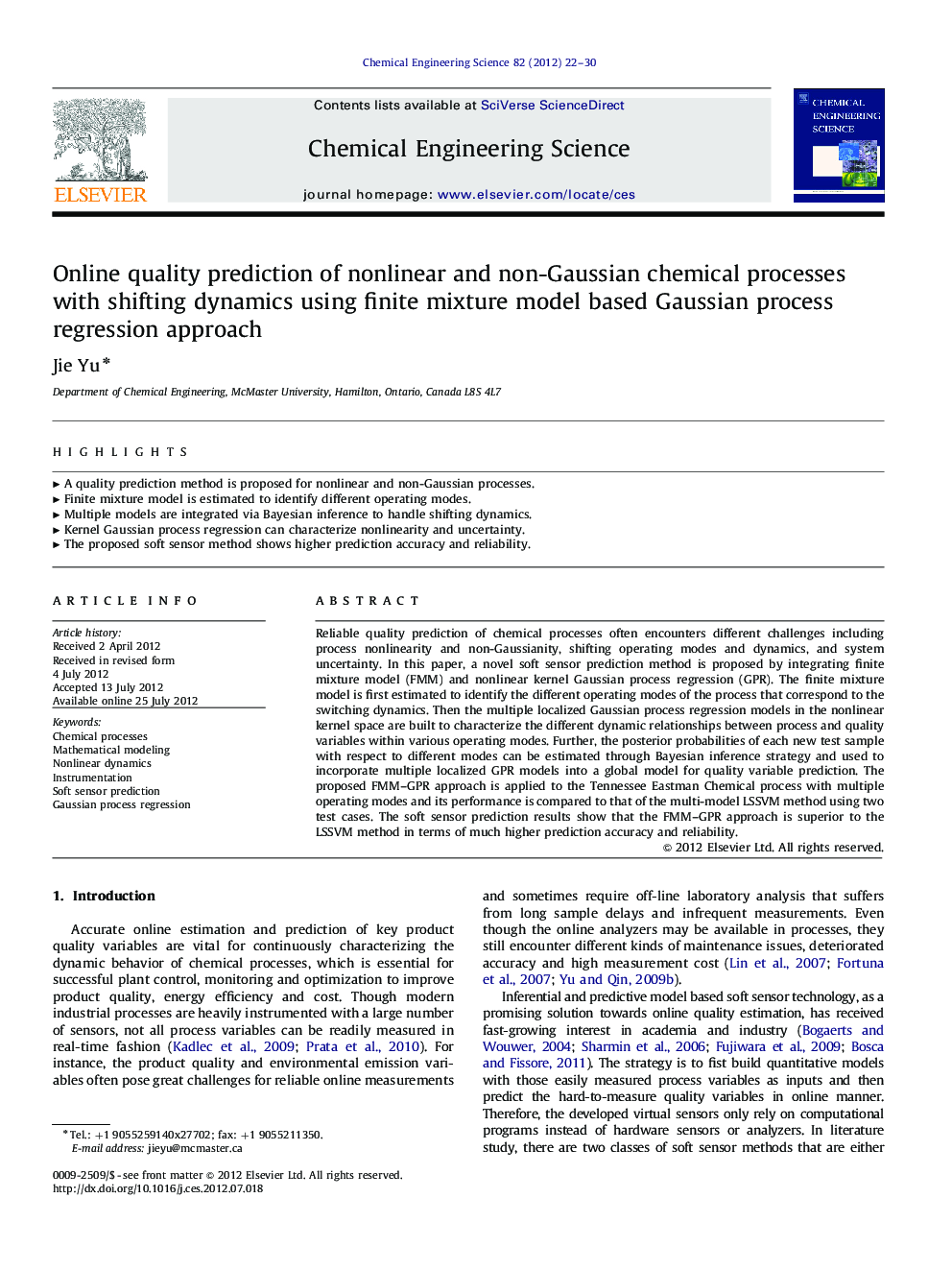
Reliable quality prediction of chemical processes often encounters different challenges including process nonlinearity and non-Gaussianity, shifting operating modes and dynamics, and system uncertainty. In this paper, a novel soft sensor prediction method is proposed by integrating finite mixture model (FMM) and nonlinear kernel Gaussian process regression (GPR). The finite mixture model is first estimated to identify the different operating modes of the process that correspond to the switching dynamics. Then the multiple localized Gaussian process regression models in the nonlinear kernel space are built to characterize the different dynamic relationships between process and quality variables within various operating modes. Further, the posterior probabilities of each new test sample with respect to different modes can be estimated through Bayesian inference strategy and used to incorporate multiple localized GPR models into a global model for quality variable prediction. The proposed FMM–GPR approach is applied to the Tennessee Eastman Chemical process with multiple operating modes and its performance is compared to that of the multi-model LSSVM method using two test cases. The soft sensor prediction results show that the FMM–GPR approach is superior to the LSSVM method in terms of much higher prediction accuracy and reliability.
► A quality prediction method is proposed for nonlinear and non-Gaussian processes.
► Finite mixture model is estimated to identify different operating modes.
► Multiple models are integrated via Bayesian inference to handle shifting dynamics.
► Kernel Gaussian process regression can characterize nonlinearity and uncertainty.
► The proposed soft sensor method shows higher prediction accuracy and reliability.
Journal: Chemical Engineering Science - Volume 82, 12 September 2012, Pages 22–30