کد مقاله | کد نشریه | سال انتشار | مقاله انگلیسی | نسخه تمام متن |
---|---|---|---|---|
1559803 | 1513888 | 2016 | 11 صفحه PDF | دانلود رایگان |
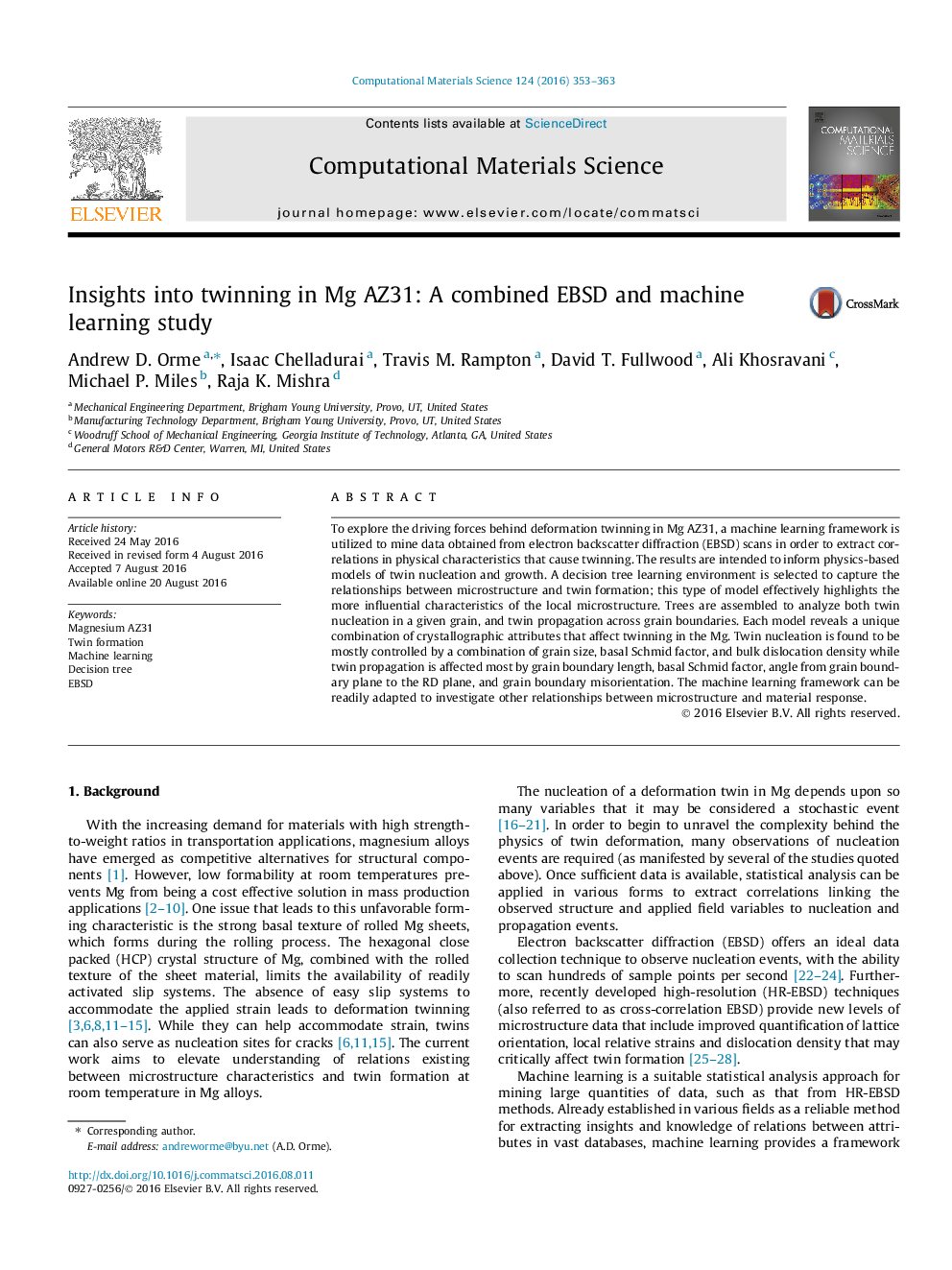
• Machine learning is applied to microstructure attributes to characterize twinning.
• Attributes influencing twin nucleation and propagation are explored.
• Machine learning highlights structure attributes leading to twinning in Mg AZ31.
• The framework developed can be readily applied to other studies of microstructure.
To explore the driving forces behind deformation twinning in Mg AZ31, a machine learning framework is utilized to mine data obtained from electron backscatter diffraction (EBSD) scans in order to extract correlations in physical characteristics that cause twinning. The results are intended to inform physics-based models of twin nucleation and growth. A decision tree learning environment is selected to capture the relationships between microstructure and twin formation; this type of model effectively highlights the more influential characteristics of the local microstructure. Trees are assembled to analyze both twin nucleation in a given grain, and twin propagation across grain boundaries. Each model reveals a unique combination of crystallographic attributes that affect twinning in the Mg. Twin nucleation is found to be mostly controlled by a combination of grain size, basal Schmid factor, and bulk dislocation density while twin propagation is affected most by grain boundary length, basal Schmid factor, angle from grain boundary plane to the RD plane, and grain boundary misorientation. The machine learning framework can be readily adapted to investigate other relationships between microstructure and material response.
Graphical AbstractFigure optionsDownload as PowerPoint slide
Journal: Computational Materials Science - Volume 124, November 2016, Pages 353–363