کد مقاله | کد نشریه | سال انتشار | مقاله انگلیسی | نسخه تمام متن |
---|---|---|---|---|
156967 | 456955 | 2010 | 9 صفحه PDF | دانلود رایگان |
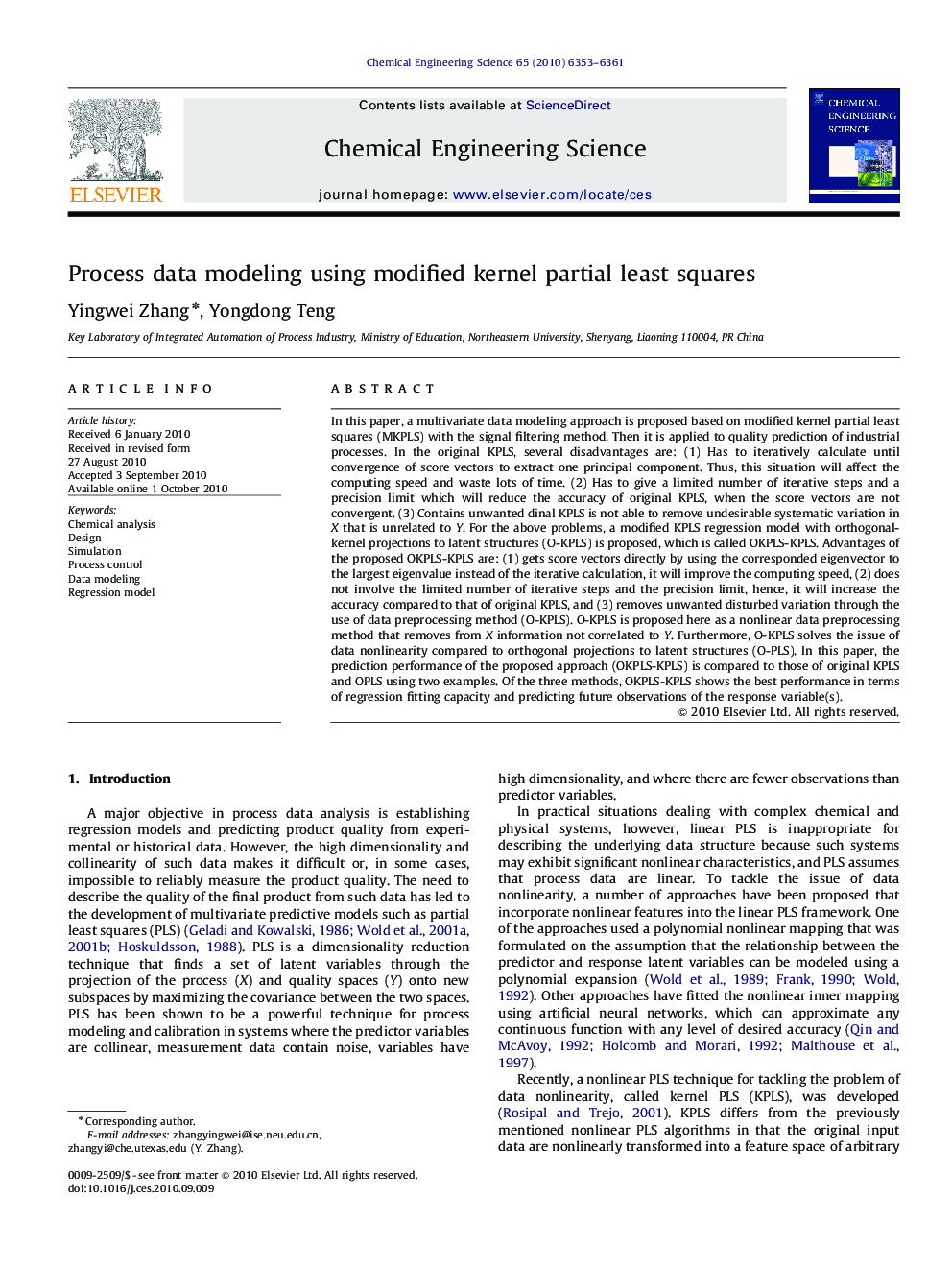
In this paper, a multivariate data modeling approach is proposed based on modified kernel partial least squares (MKPLS) with the signal filtering method. Then it is applied to quality prediction of industrial processes. In the original KPLS, several disadvantages are: (1) Has to iteratively calculate until convergence of score vectors to extract one principal component. Thus, this situation will affect the computing speed and waste lots of time. (2) Has to give a limited number of iterative steps and a precision limit which will reduce the accuracy of original KPLS, when the score vectors are not convergent. (3) Contains unwanted dinal KPLS is not able to remove undesirable systematic variation in X that is unrelated to Y. For the above problems, a modified KPLS regression model with orthogonal-kernel projections to latent structures (O-KPLS) is proposed, which is called OKPLS-KPLS. Advantages of the proposed OKPLS-KPLS are: (1) gets score vectors directly by using the corresponded eigenvector to the largest eigenvalue instead of the iterative calculation, it will improve the computing speed, (2) does not involve the limited number of iterative steps and the precision limit, hence, it will increase the accuracy compared to that of original KPLS, and (3) removes unwanted disturbed variation through the use of data preprocessing method (O-KPLS). O-KPLS is proposed here as a nonlinear data preprocessing method that removes from X information not correlated to Y. Furthermore, O-KPLS solves the issue of data nonlinearity compared to orthogonal projections to latent structures (O-PLS). In this paper, the prediction performance of the proposed approach (OKPLS-KPLS) is compared to those of original KPLS and OPLS using two examples. Of the three methods, OKPLS-KPLS shows the best performance in terms of regression fitting capacity and predicting future observations of the response variable(s).
Journal: Chemical Engineering Science - Volume 65, Issue 24, 15 December 2010, Pages 6353–6361