کد مقاله | کد نشریه | سال انتشار | مقاله انگلیسی | نسخه تمام متن |
---|---|---|---|---|
1887091 | 1043571 | 2012 | 10 صفحه PDF | دانلود رایگان |
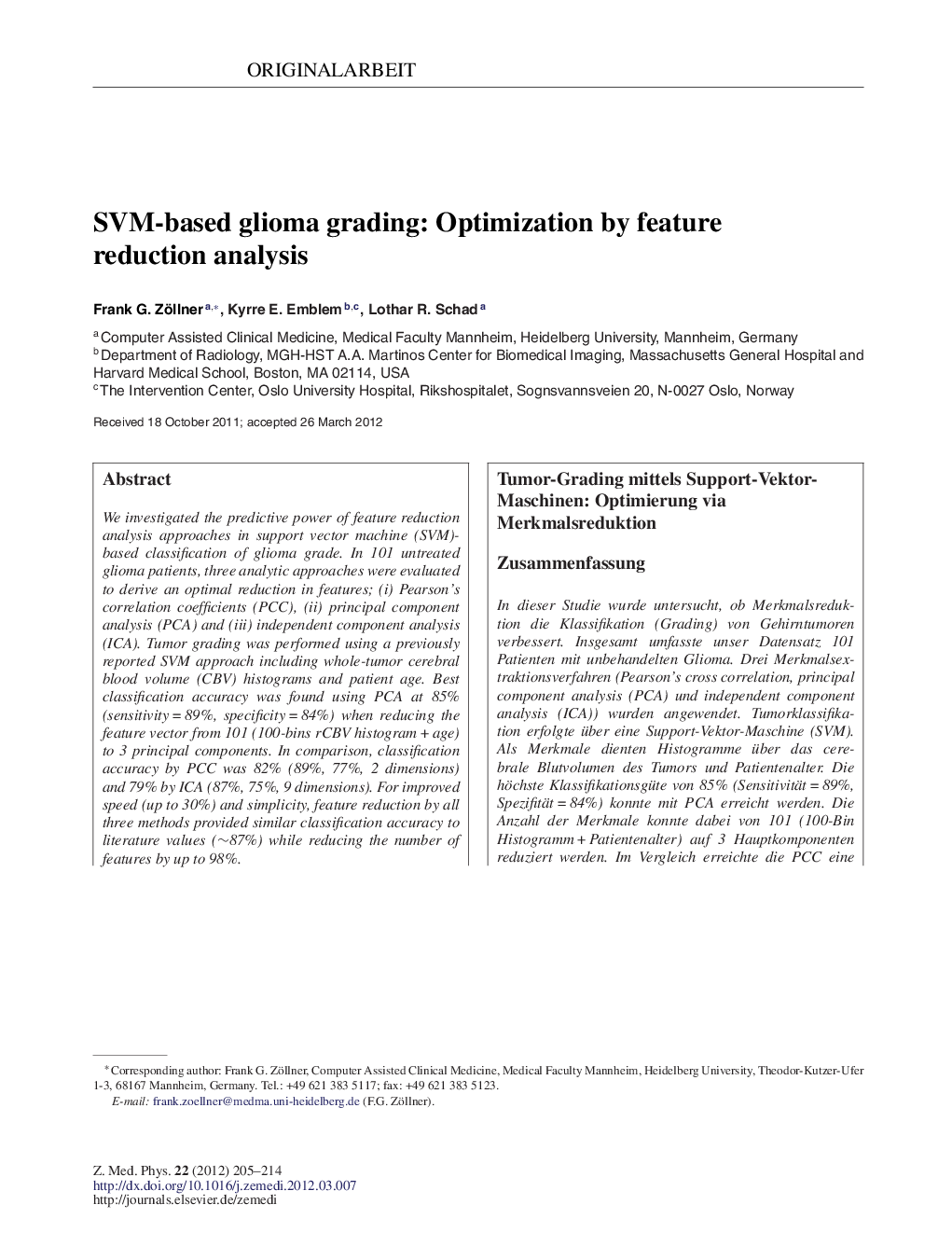
We investigated the predictive power of feature reduction analysis approaches in support vector machine (SVM)-based classification of glioma grade. In 101 untreated glioma patients, three analytic approaches were evaluated to derive an optimal reduction in features; (i) Pearson's correlation coefficients (PCC), (ii) principal component analysis (PCA) and (iii) independent component analysis (ICA). Tumor grading was performed using a previously reported SVM approach including whole-tumor cerebral blood volume (CBV) histograms and patient age. Best classification accuracy was found using PCA at 85% (sensitivity = 89%, specificity = 84%) when reducing the feature vector from 101 (100-bins rCBV histogram + age) to 3 principal components. In comparison, classification accuracy by PCC was 82% (89%, 77%, 2 dimensions) and 79% by ICA (87%, 75%, 9 dimensions). For improved speed (up to 30%) and simplicity, feature reduction by all three methods provided similar classification accuracy to literature values (∼87%) while reducing the number of features by up to 98%.
ZusammenfassungIn dieser Studie wurde untersucht, ob Merkmalsreduktion die Klassifikation (Grading) von Gehirntumoren verbessert. Insgesamt umfasste unser Datensatz 101 Patienten mit unbehandelten Glioma. Drei Merkmalsextraktionsverfahren (Pearson's cross correlation, principal component analysis (PCA) und independent component analysis (ICA)) wurden angewendet. Tumorklassifikation erfolgte über eine Support-Vektor-Maschine (SVM). Als Merkmale dienten Histogramme über das cerebrale Blutvolumen des Tumors und Patientenalter. Die höchste Klassifikationsgüte von 85% (Sensitivität = 89%, Spezifität = 84%) konnte mit PCA erreicht werden. Die Anzahl der Merkmale konnte dabei von 101 (100-Bin Histogramm + Patientenalter) auf 3 Hauptkomponenten reduziert werden. Im Vergleich erreichte die PCC eine korrekte Klassifizierung von 82% (89%, 77%, 2 Dimensionen) und die ICA von 79% (87%, 75%, 9 Dimensionen). Bei gleichbleibender Klassifikationsgüte im Vergleich zur Literatur (∼ 87%), konnten eine Reduktion von bis 98% der Merkmalesdimension als auch eine Reduktion der Berechnungszeit von bis zu 30% erreicht werden.
Journal: Zeitschrift für Medizinische Physik - Volume 22, Issue 3, September 2012, Pages 205–214